Sparse group selection and analysis of function-related residue for protein-state recognition
JOURNAL OF COMPUTATIONAL CHEMISTRY(2022)
摘要
Machine learning methods have helped to advance wide range of scientific and technological field in recent years, including computational chemistry. As the chemical systems could become complex with high dimension, feature selection could be critical but challenging to develop reliable machine learning based prediction models, especially for proteins as bio-macromolecules. In this study, we applied sparse group lasso (SGL) method as a general feature selection method to develop classification model for an allosteric protein in different functional states. This results into a much improved model with comparable accuracy (Acc) and only 28 selected features comparing to 289 selected features from a previous study. The Acc achieves 91.50% with 1936 selected feature, which is far higher than that of baseline methods. In addition, grouping protein amino acids into secondary structures provides additional interpretability of the selected features. The selected features are verified as associated with key allosteric residues through comparison with both experimental and computational works about the model protein, and demonstrate the effectiveness and necessity of applying rigorous feature selection and evaluation methods on complex chemical systems.
更多查看译文
关键词
classification, feature selection, function-related residues, protein states, sparse group lasso
AI 理解论文
溯源树
样例
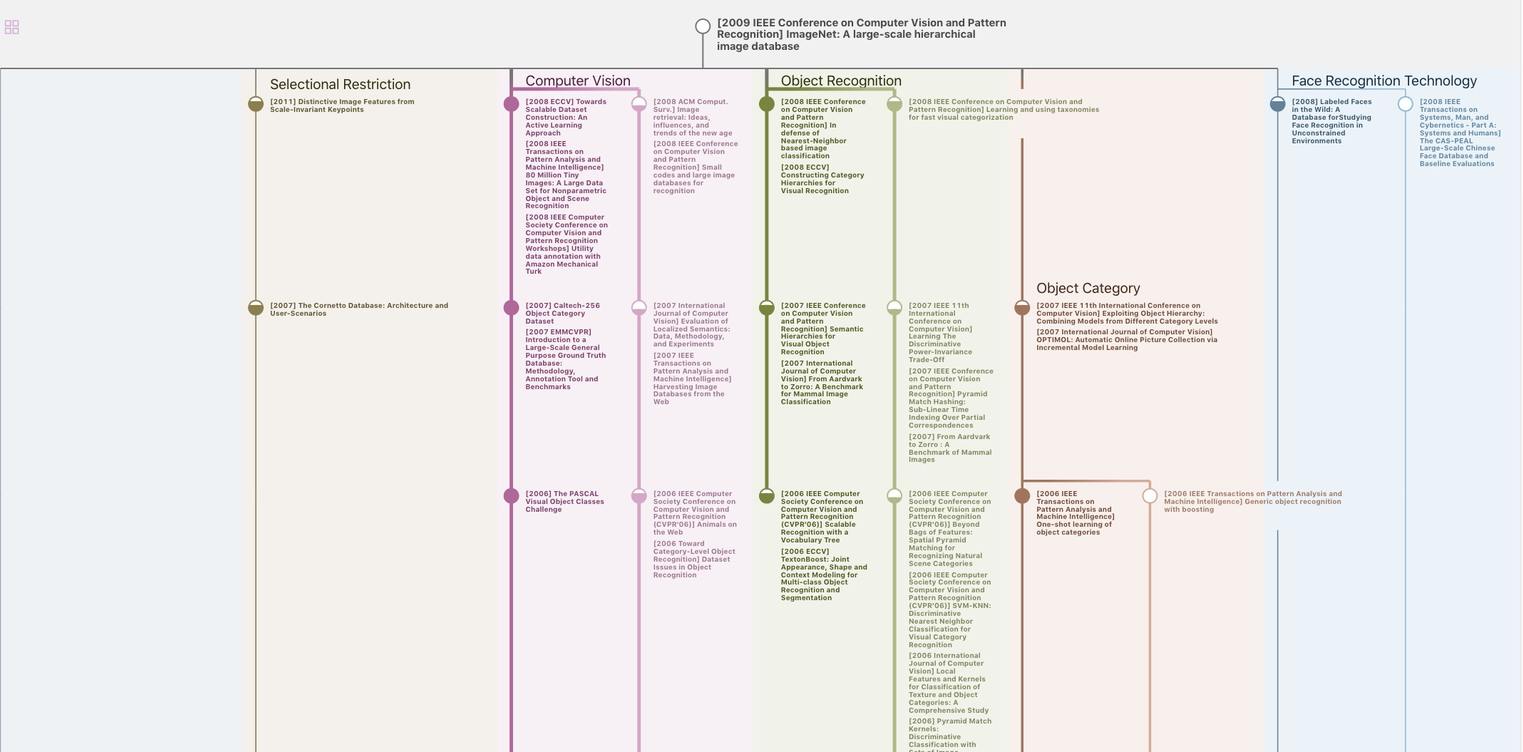
生成溯源树,研究论文发展脉络
Chat Paper
正在生成论文摘要