Topology Adaptive Graph Convolution Network With Heterogeneous Entities For Predicting Adverse Events from Drug-Drug-Interactions
biorxiv(2022)
摘要
In times like this, it is imperative to be cautious about the effects of drugs or vaccination doses on patients who are already suffering from other serious diseases. It’s not only the virus which can affect the body metabolisms, drugs to encounter the virus may also end up having unwanted negative effects. Therapeutic activities of drugs are often influenced by co-administration of drugs that may cause inevitable drug-drug interactions and inadvertent side effects. Therefore, prediction and identification of DDIs are extremely vital for patient safety and treatment modalities. In this context, we intend to develop a computational method based on functional similarity of drugs. Our objective has been enhanced by the usage of knowledge graphs which allows capturing underlying information embeddings in a biological network with heterogenous entities. On providing this knowledge graph as the input to a Topology Adaptive Graph Convolution Network which performs topologically-aware flexible convolutions, we achieve improvements on priorly proposed GCN models that has been shown as comparison.
### Competing Interest Statement
The authors have declared no competing interest.
更多查看译文
关键词
network,drug-drug-interactions
AI 理解论文
溯源树
样例
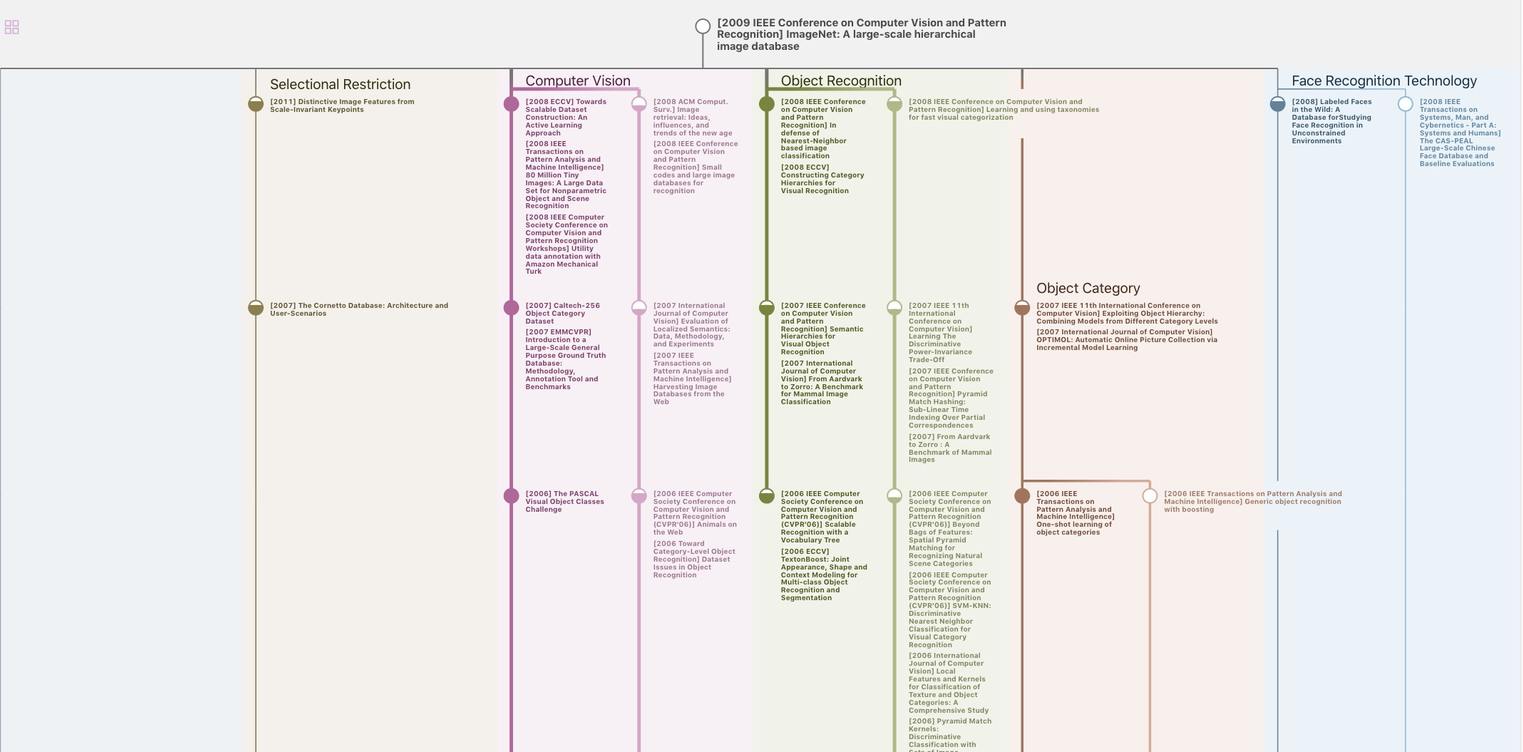
生成溯源树,研究论文发展脉络
Chat Paper
正在生成论文摘要