Deciphering the Impact of Genetic Variation on Human Polyadenylation
biorxiv(2022)
摘要
Genetic variants that disrupt polyadenylation can cause or contribute to genetic disorders. Yet, due to the complex cis-regulation of polyadenylation, variant interpretation remains challenging. Here, we introduce a residual neural network model, APARENT2 , that can infer 3’-cleavage and polyadenylation from DNA sequence more accurately than any previous model. This model generalizes to the case of alternative polyadenylation (APA) for a variable number of polyadenylation signals. We demonstrate APARENT2’s performance on several variant datasets, including functional reporter data and human 3’ aQTLs from GTEx. We apply neural network interpretation methods to gain insights into disrupted or protective higher-order features of polyadenylation. We fine-tune APARENT2 on human tissue-resolved transcriptomic data to elucidate tissue-specific variant effects. Finally, we perform in-silico saturation mutagenesis of all human polyadenylation signals and compare the predicted effects of >44 million variants against gnomAD. While loss-of-function variants were generally selected against, we also find specific clinical conditions linked to gain-of-function mutations. For example, using APARENT2’s predictions we detect an association between gain-of-function mutations in the 3’-end and Autism Spectrum Disorder.
### Competing Interest Statement
A.K. is a scientific co-founder of Ravel Biotechnology Inc., is on the SAB of PatchBio Inc., SerImmune Inc., AINovo Inc., TensorBio Inc. and OpenTargets, is a consultant with Illumina Inc. and owns shares in DeepGenomics Inc., Immuni Inc. and Freenome Inc. G.S. is a co-founder of Parse Biosciences and is on the SAB of Modulus Therapeutics.
更多查看译文
AI 理解论文
溯源树
样例
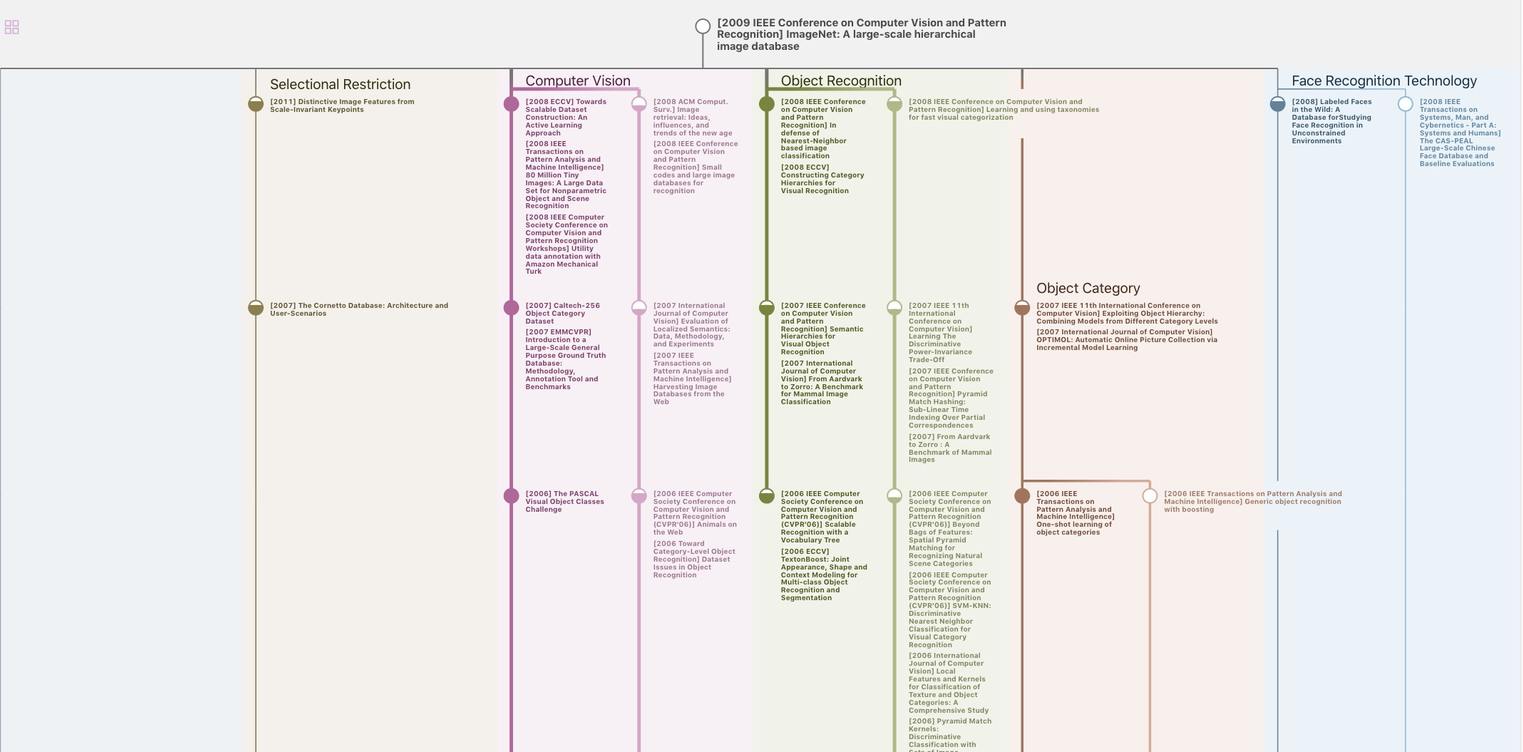
生成溯源树,研究论文发展脉络
Chat Paper
正在生成论文摘要