Deep reinforcement learning for optimal experimental design in biology.
PLoS computational biology(2022)
摘要
The field of optimal experimental design uses mathematical techniques to determine experiments that are maximally informative from a given experimental setup. Here we apply a technique from artificial intelligence-reinforcement learning-to the optimal experimental design task of maximizing confidence in estimates of model parameter values. We show that a reinforcement learning approach performs favourably in comparison with a one-step ahead optimisation algorithm and a model predictive controller for the inference of bacterial growth parameters in a simulated chemostat. Further, we demonstrate the ability of reinforcement learning to train over a distribution of parameters, indicating that this approach is robust to parametric uncertainty.
更多查看译文
关键词
optimal experimental design,deep reinforcement learning,biology
AI 理解论文
溯源树
样例
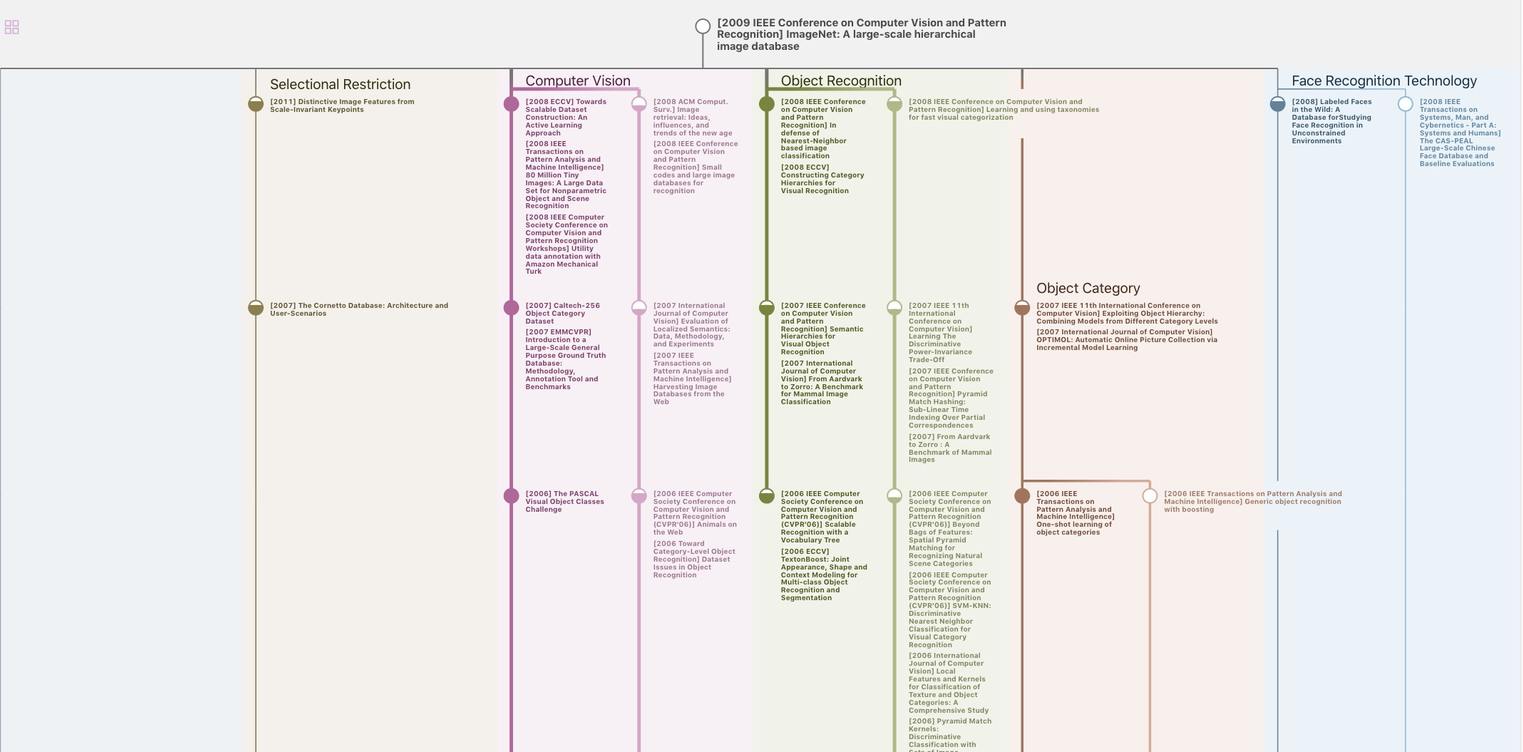
生成溯源树,研究论文发展脉络
Chat Paper
正在生成论文摘要