MegaBayesianAlphabet: Mega-scale Bayesian Regression methods for genome-wide prediction and association studies with thousands of traits
biorxiv(2022)
摘要
Large-scale phenotype data are expected to increase the accuracy of genome-wide prediction and the power of genome-wide association analyses. However, genomic analyses of high-dimensional, highly correlated data are challenging. We developed MegaBayesianAlphabet to simultaneously analyze genetic variants underlying thousands of traits using the flexible priors of the Bayesian Alphabet family. As a demonstration, we implemented the BayesC prior in the R package MegaLMM and applied it to both simulated and real data sets. Our analyses show that the resulting model MegaBayesC can effectively use high-dimensional phenotypic data to improve the accuracy of genetic value prediction, the reliability of marker discovery, and the accuracy of marker effect size estimation in genome-wide analyses.
更多查看译文
关键词
Bayesian regression models,genome-wide association studies,genomic prediction,high-throughput phenotyping,multi-trait
AI 理解论文
溯源树
样例
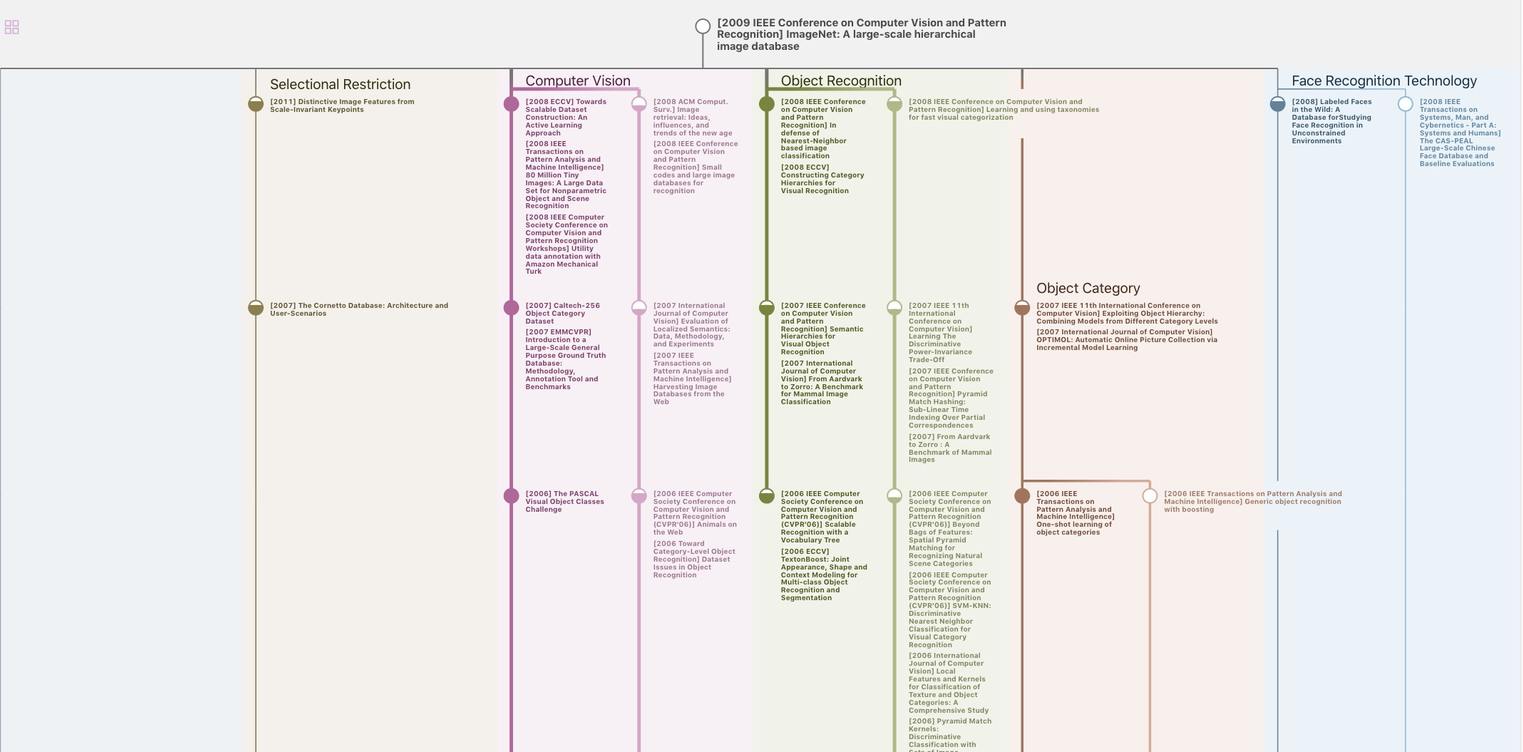
生成溯源树,研究论文发展脉络
Chat Paper
正在生成论文摘要