Incorporating selection pressures into risk predictions of chemical resistance evolution in pest organisms
biorxiv(2022)
摘要
The evolution of chemical resistance in pests is a global threat to food security and human health, yet resistance issues are mostly dealt with after they occur. Predictive models of resistance risk provide an opportunity for field practitioners to implement proactive strategies to manage pest species more effectively. These models require identification of variables that are associated with resistance risk. Phagy and voltinism, for example, have been previously shown as useful predictors of resistance evolution. Yet despite the importance of chemical selection pressure in resistance evolution, this predictor has been difficult to incorporate into predictive models due to incomplete and (or) poor datasets on chemical usage patterns. Here we test whether chemical registrations of pesticide products can act as a proxy for selection pressures in predictive models. We obtained pesticide product registrations from publicly available chemical databases for 427 agricultural arthropod pest species in the USA and 209 Australian species. We constructed Bayesian logistic regressions of resistance status as a function of the number of pesticide product registrations for 42 chemical Mode of Action (MoA) groups, as well as phagy and voltinism. These models were well supported by our data and cross-validation analyses and could discriminate between observations of resistance and susceptibility in both USA and Australian species sets. Moreover, we found strong support for a positive correlation between pesticide product registrations and resistance status. We propose a “registration–selection–resistance” hypothesis to explain this positive association, involving a positive feedback loop between marketing and social factors of pesticide use with the evolutionary processes of selection and adaptation. Pesticide product registrations provide a predictive variable that is specific to both pest species and chemical MoA groups. Our work aids the development of predictive models for proactive resistance management, combining ecological and operational factors, with broad applicability across a suite of agricultural pests, including weeds and fungi.
### Competing Interest Statement
The authors have declared no competing interest.
更多查看译文
AI 理解论文
溯源树
样例
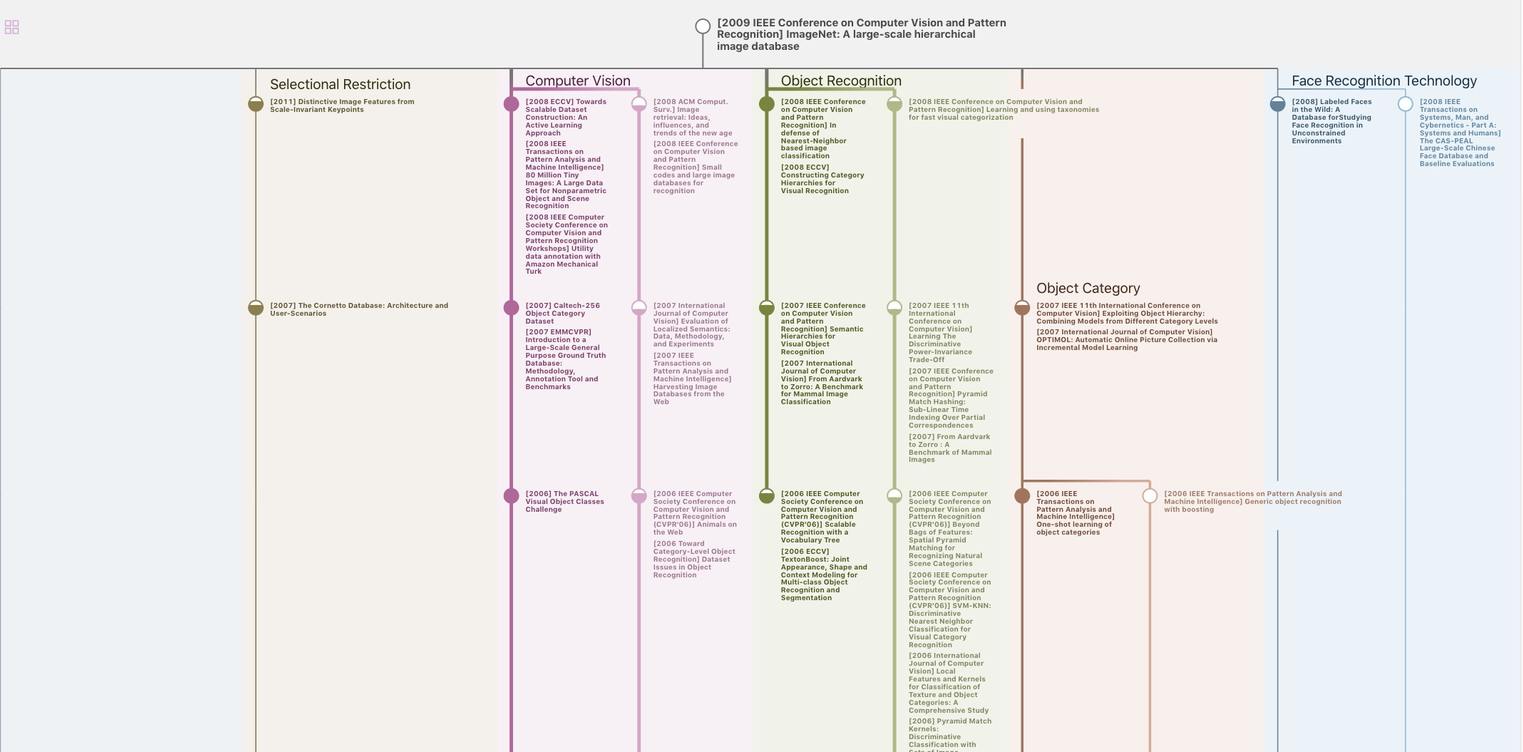
生成溯源树,研究论文发展脉络
Chat Paper
正在生成论文摘要