Causal Structure Learning: A Combinatorial Perspective
Foundations of computational mathematics (New York, N.Y.)(2022)
摘要
In this review, we discuss approaches for learning causal structure from data, also called causal discovery . In particular, we focus on approaches for learning directed acyclic graphs and various generalizations which allow for some variables to be unobserved in the available data. We devote special attention to two fundamental combinatorial aspects of causal structure learning. First, we discuss the structure of the search space over causal graphs. Second, we discuss the structure of equivalence classes over causal graphs, i.e., sets of graphs which represent what can be learned from observational data alone, and how these equivalence classes can be refined by adding interventional data.
更多查看译文
关键词
Causal inference,Causal structure discovery,Markov equivalence
AI 理解论文
溯源树
样例
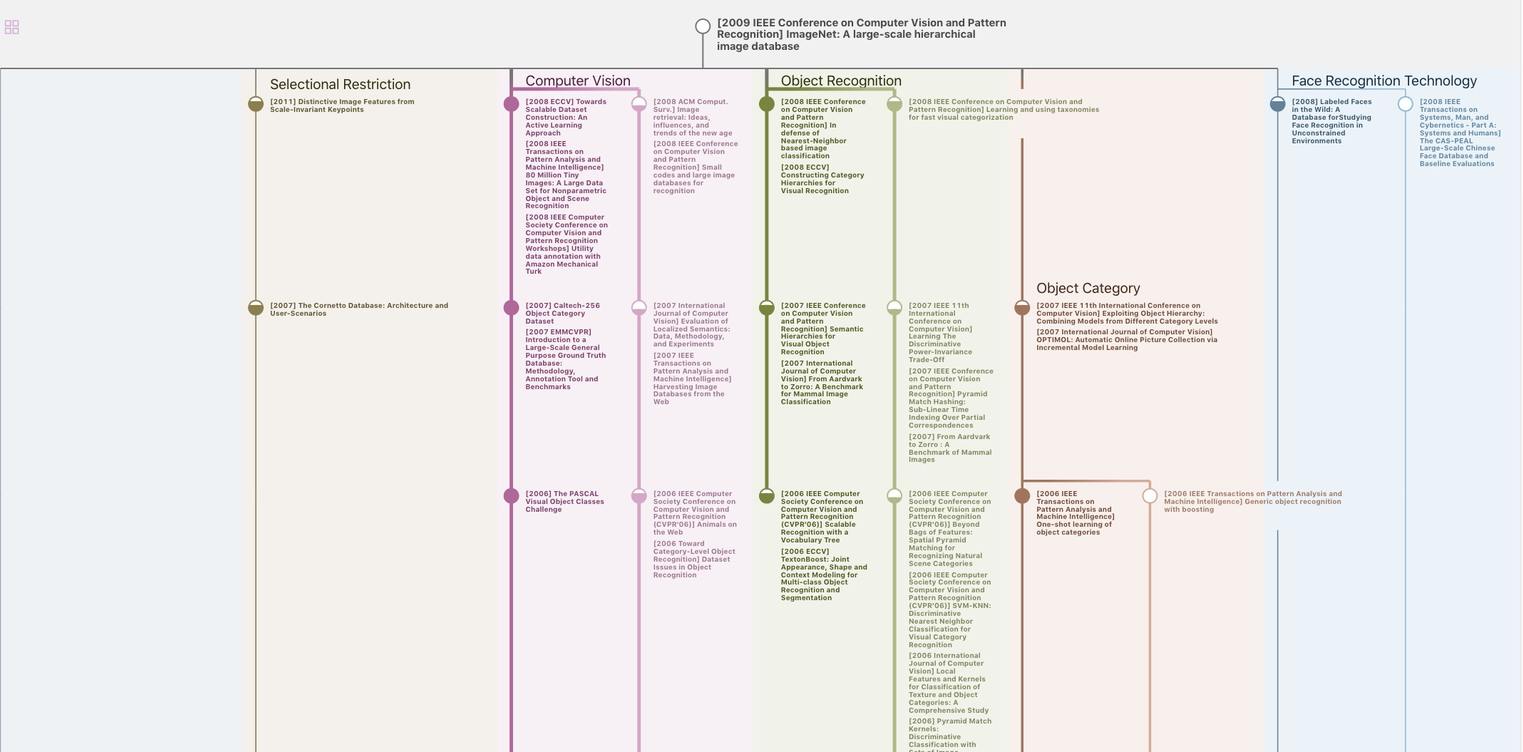
生成溯源树,研究论文发展脉络
Chat Paper
正在生成论文摘要