Introducing One Sided Margin Loss for Solving Classification Problems in Deep Networks
arxiv(2022)
摘要
This paper introduces a new loss function, OSM (One-Sided Margin), to solve maximum-margin classification problems effectively. Unlike the hinge loss, in OSM the margin is explicitly determined with corresponding hyperparameters and then the classification problem is solved. In experiments, we observe that using OSM loss leads to faster training speeds and better accuracies than binary and categorical cross-entropy in several commonly used deep models for classification and optical character recognition problems. OSM has consistently shown better classification accuracies over cross-entropy and hinge losses for small to large neural networks. it has also led to a more efficient training procedure. We achieved state-of-the-art accuracies for small networks on several benchmark datasets of CIFAR10(98.82\%), CIFAR100(91.56\%), Flowers(98.04\%), Stanford Cars(93.91\%) with considerable improvements over other loss functions. Moreover, the accuracies are rather better than cross-entropy and hinge loss for large networks. Therefore, we strongly believe that OSM is a powerful alternative to hinge and cross-entropy losses to train deep neural networks on classification tasks.
更多查看译文
关键词
sided margin loss,deep networks,classification problems
AI 理解论文
溯源树
样例
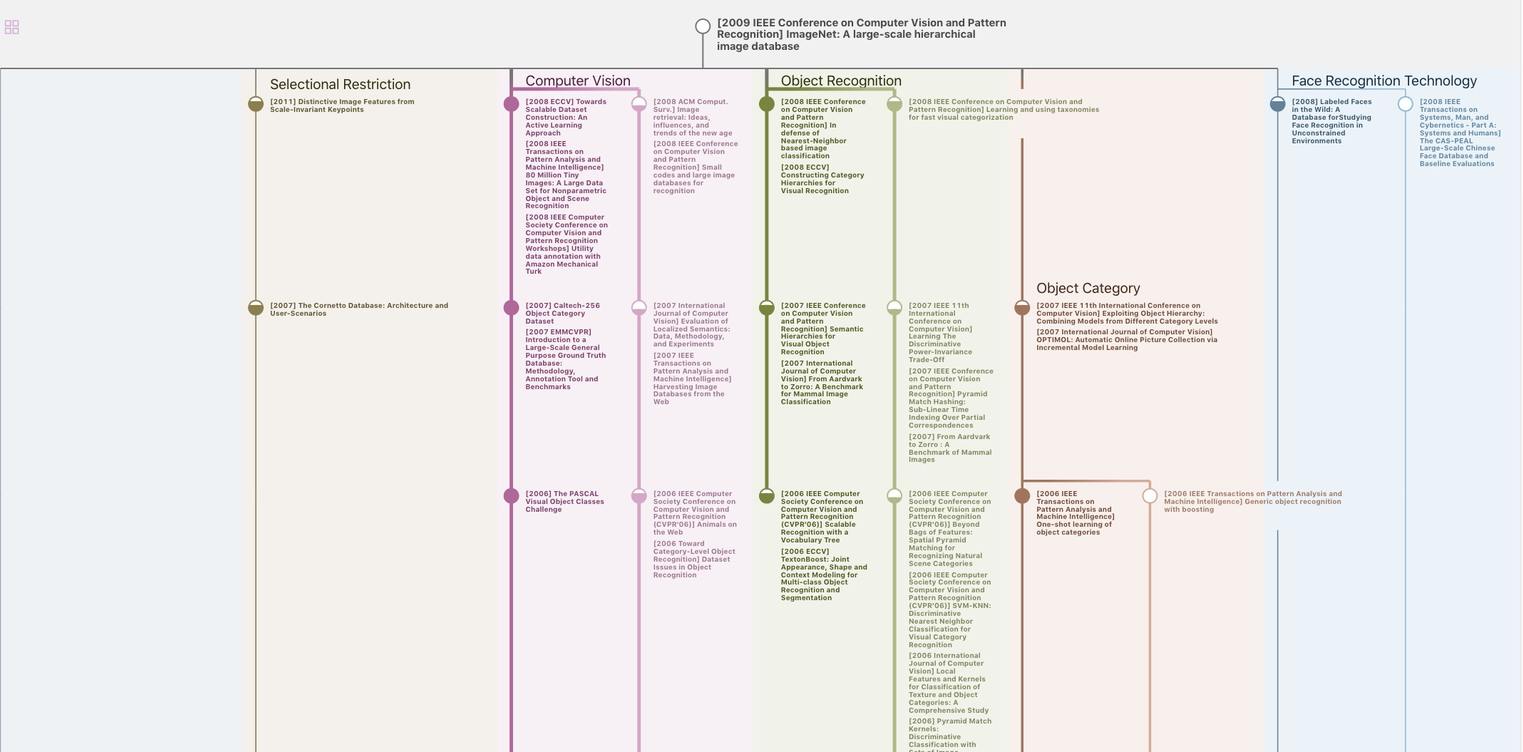
生成溯源树,研究论文发展脉络
Chat Paper
正在生成论文摘要