StopNet: Scalable Trajectory and Occupancy Prediction for Urban Autonomous Driving.
IEEE International Conference on Robotics and Automation(2022)
摘要
We introduce a motion forecasting (behavior prediction) method that meets the latency requirements for autonomous driving in dense urban environments without sacrificing accuracy. A whole-scene sparse input representation allows StopNet to scale to predicting trajectories for hundreds of road agents with reliable latency. In addition to predicting trajectories, our scene encoder lends itself to predicting whole-scene probabilistic occupancy grids, a complementary output representation suitable for busy urban environments. Occupancy grids allow the AV to reason collectively about the behavior of groups of agents without processing their individual trajectories. We demonstrate the effectiveness of our sparse input representation and our model in terms of computation and accuracy over three datasets. We further show that co-training consistent trajectory and occupancy predictions improves upon state-of-the-art performance under standard metrics.
更多查看译文
关键词
StopNet,scalable trajectory,occupancy prediction,urban autonomous driving,motion forecasting method,behavior prediction,latency requirements,dense urban environments,sacrificing accuracy,whole-scene sparse input representation,predicting trajectories,road agents,scene encoder,whole-scene probabilistic occupancy grids,complementary output representation,busy urban environments,individual trajectories,co-training consistent trajectory,occupancy predictions
AI 理解论文
溯源树
样例
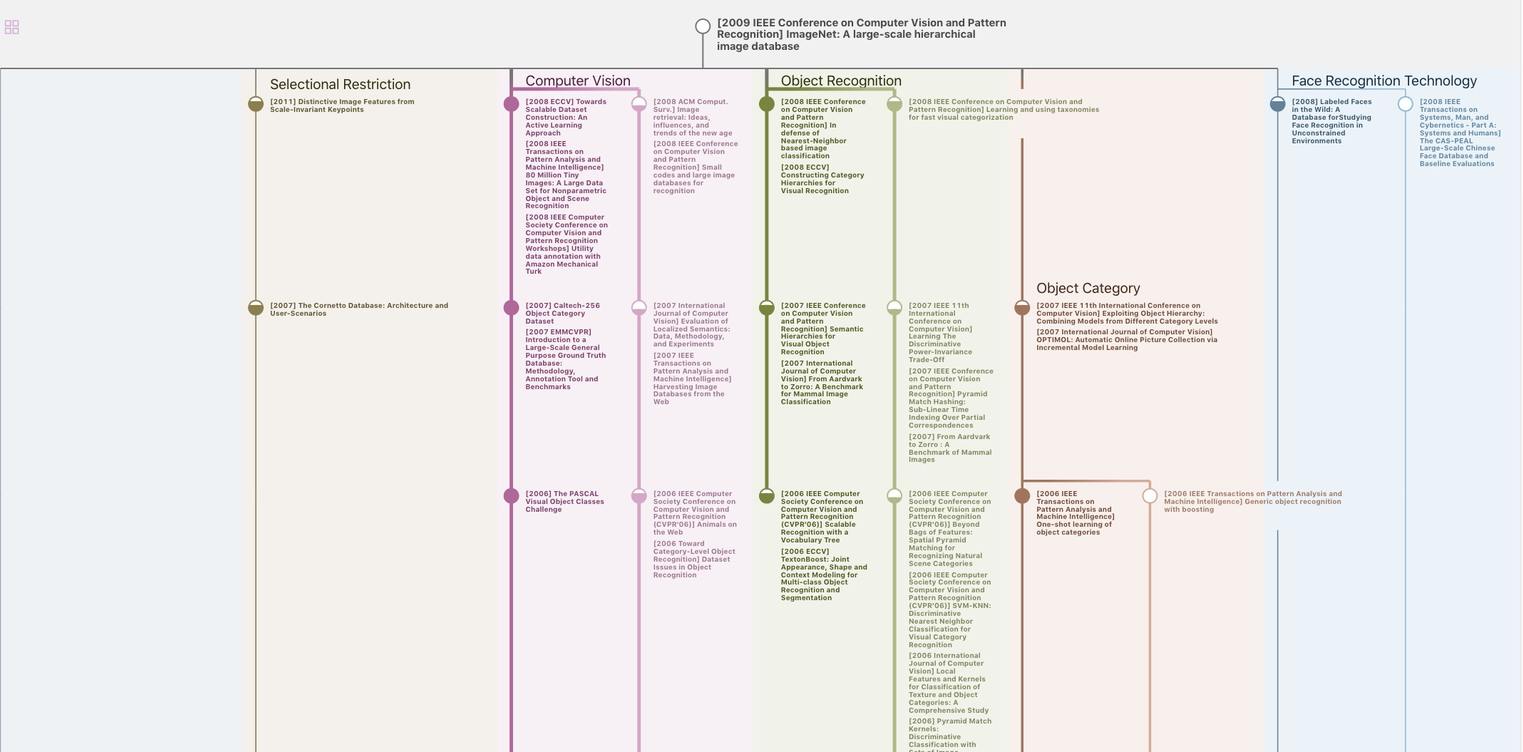
生成溯源树,研究论文发展脉络
Chat Paper
正在生成论文摘要