Neural Decoding With Optimization of Node Activations
IEEE Communications Letters(2022)
摘要
The problem of maximum likelihood decoding with a neural decoder for error-correcting code is considered. It is shown that the neural decoder can be improved with two novel loss terms on the node’s activations. The first loss term imposes a sparse constraint on the node’s activations. Whereas, the second loss term tried to mimic the node’s activations from a teacher decoder which has better performance. The proposed method has the same run time complexity and model size as the neural Belief Propagation decoder, while improving the decoding performance by up to
$1.1dB$
on BCH codes.
更多查看译文
关键词
Information theory,deep learning,error correcting codes,neural decoder
AI 理解论文
溯源树
样例
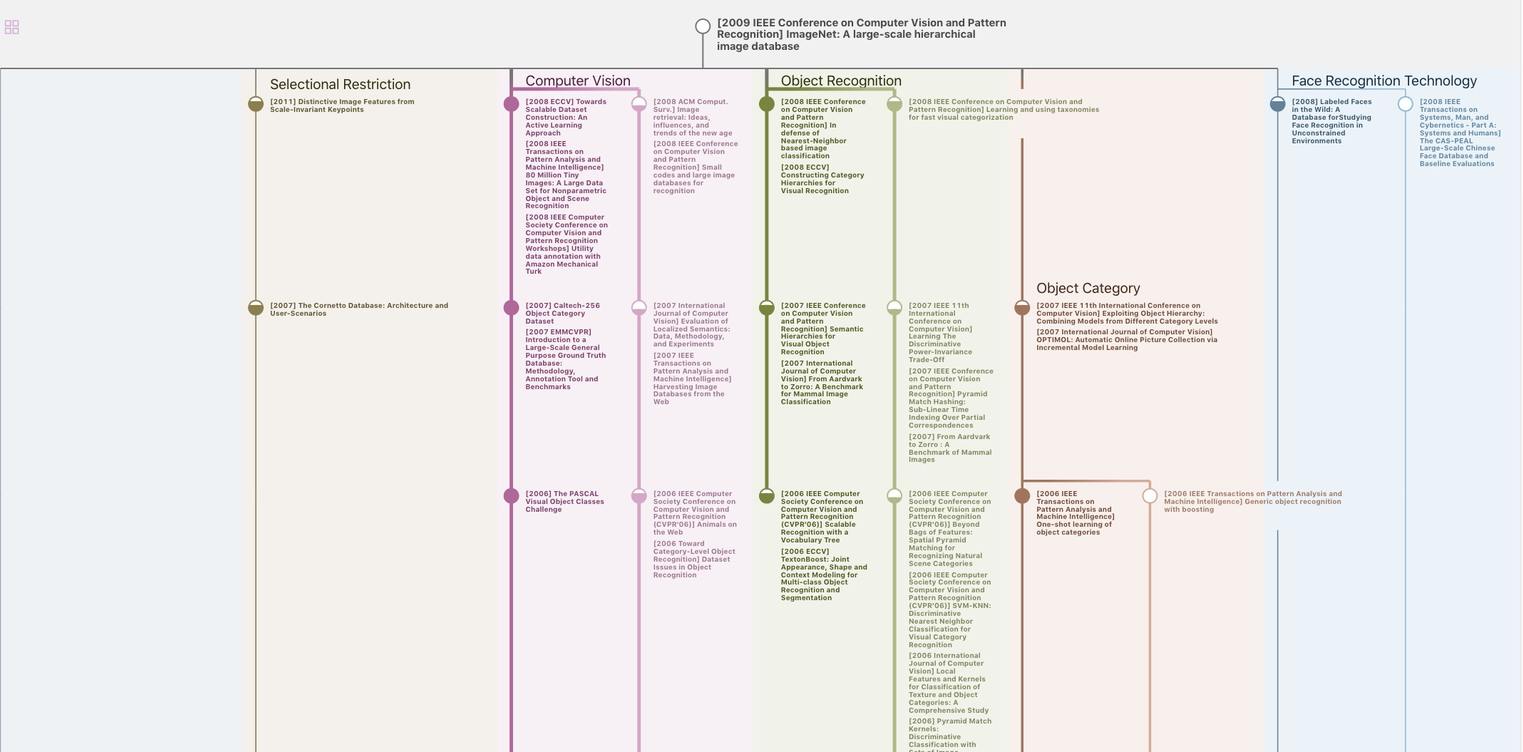
生成溯源树,研究论文发展脉络
Chat Paper
正在生成论文摘要