The Phenomenon of Policy Churn
NeurIPS 2022(2022)
摘要
We identify and study the phenomenon of policy churn, that is, the rapid change of the greedy policy in value-based reinforcement learning. Policy churn operates at a surprisingly rapid pace, changing the greedy action in a large fraction of states within a handful of learning updates (in a typical deep RL set-up such as DQN on Atari). We characterise the phenomenon empirically, verifying that it is not limited to specific algorithm or environment properties. A number of ablations help whittle down the plausible explanations on why churn occurs to just a handful, all related to deep learning. Finally, we hypothesise that policy churn is a beneficial but overlooked form of implicit exploration that casts $\epsilon$-greedy exploration in a fresh light, namely that $\epsilon$-noise plays a much smaller role than expected.
更多查看译文
关键词
Reinforcement Learning,Exploration,Deep learning,Deep RL,Policy space,Stability
AI 理解论文
溯源树
样例
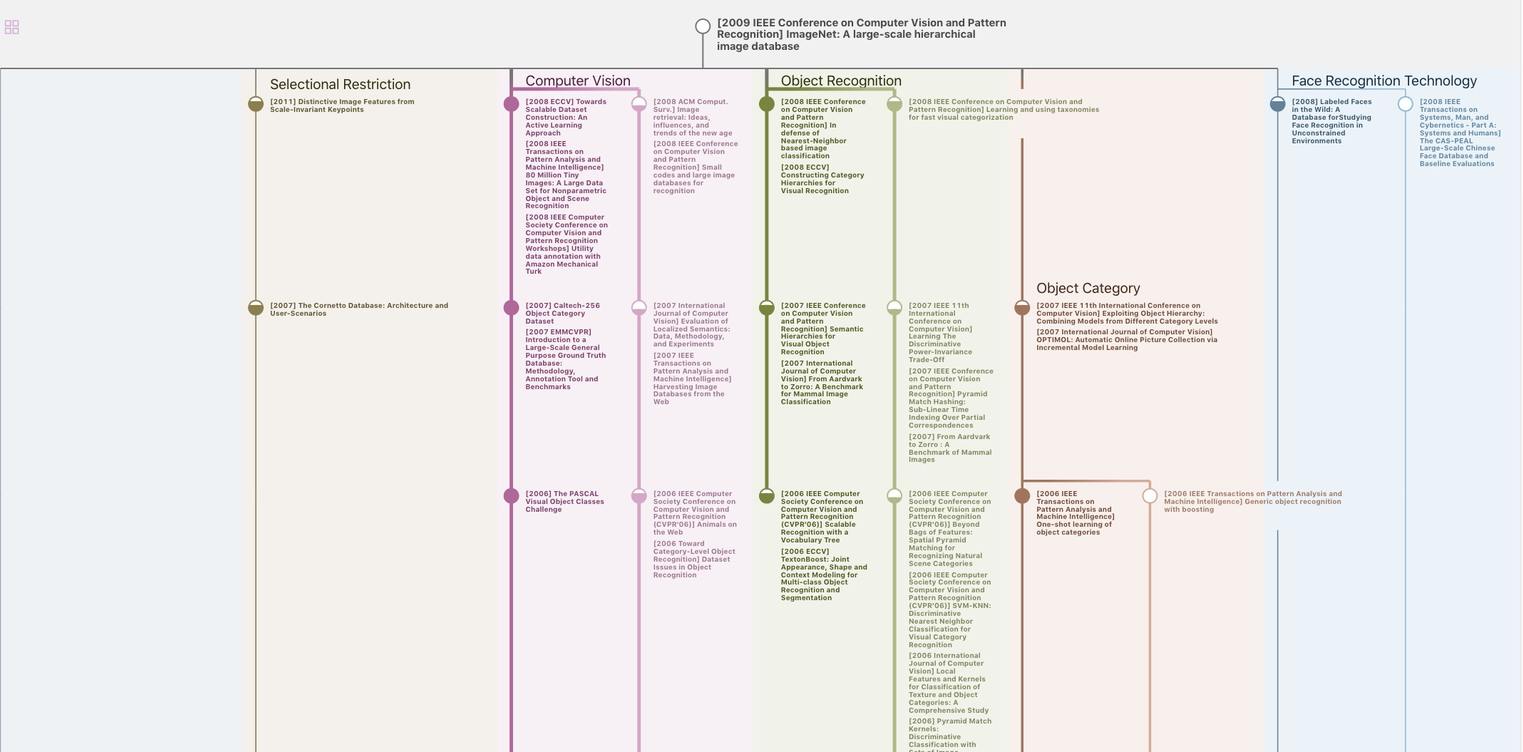
生成溯源树,研究论文发展脉络
Chat Paper
正在生成论文摘要