Meta-SysId: A Meta-Learning Approach for Simultaneous Identification and Prediction
arxiv(2022)
摘要
In this paper, we propose Meta-SysId, a meta-learning approach to model sets of systems that have behavior governed by common but unknown laws and that differentiate themselves by their context. Inspired by classical modeling-and-identification approaches, Meta-SysId learns to represent the common law through shared parameters and relies on online optimization to compute system-specific context. Compared to optimization-based meta-learning methods, the separation between class parameters and context variables reduces the computational burden while allowing batch computations and a simple training scheme. We test Meta-SysId on polynomial regression, time-series prediction, model-based control, and real-world traffic prediction domains, empirically finding it outperforms or is competitive with meta-learning baselines.
更多查看译文
关键词
simultaneous identification,prediction,meta-sysid,meta-learning
AI 理解论文
溯源树
样例
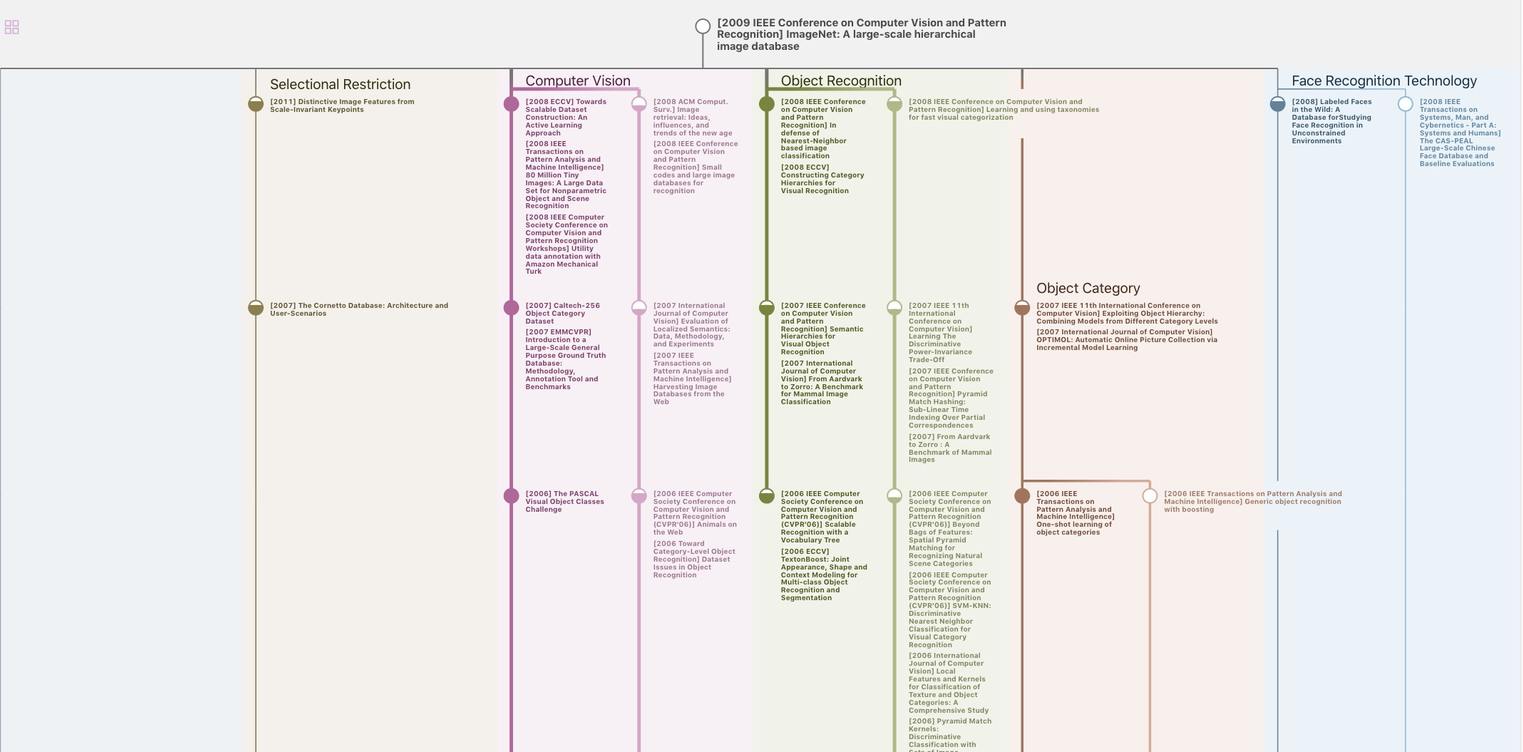
生成溯源树,研究论文发展脉络
Chat Paper
正在生成论文摘要