Retraining an Artificial Intelligence Algorithm to Calculate Left Ventricular Ejection Fraction in Pediatrics
Journal of Cardiothoracic and Vascular Anesthesia(2022)
摘要
Objectives
Identifying patients with low left ventricular ejection fraction (LVEF) and monitoring LVEF responses to treatment are important clinical goals. Can a deep-learning algorithm predict pediatric LVEF within clinically acceptable error?
Design
The study authors wanted to fine-tune an adult deep-learning algorithm to calculate LVEF in pediatric patients. A priori, their objective was to refine the algorithm to perform LVEF calculation with a mean absolute error (MAE) ≤5%.
Setting
A quaternary pediatric hospital
Participants
A convenience sample (n = 321) of echocardiograms from newborns to 18 years old with normal cardiac anatomy or hemodynamically insignificant anomalies. Echocardiograms were chosen from a group of healthy controls with known normal LVEF (n = 267) and a dilated cardiomyopathy patient group with reduced LVEF (n = 54).
Interventions
The artificial intelligence model EchoNet-Dynamic was tested on this data set and then retrained, tested, and further validated to improve LVEF calculation. The gold standard value was LVEF calculated by clinical experts.
Measurements and Main Results
In a random subset of subjects (n = 40) not analyzed prior to selection of the final model, EchoNet-Dynamic calculated LVEF with a MAE of 8.39%, R2 = 0.47 without, and MAE 4.47%, R2 = 0.87 with fine-tuning. Bland-Altman analysis suggested that the model slightly underestimates LVEF (bias = –2.42%). The 95% limits of agreement between actual and calculated values were –12.32% to 7.47%.
Conclusions
The fine-tuned model calculates LVEF in a range of pediatric patients within clinically acceptable error. Potential advantages include reducing operator error in LVEF calculation and supporting independent LVEF assessment by inexperienced users.
更多查看译文
关键词
artificial intelligence,deep learning,pediatric patients,cardiac function
AI 理解论文
溯源树
样例
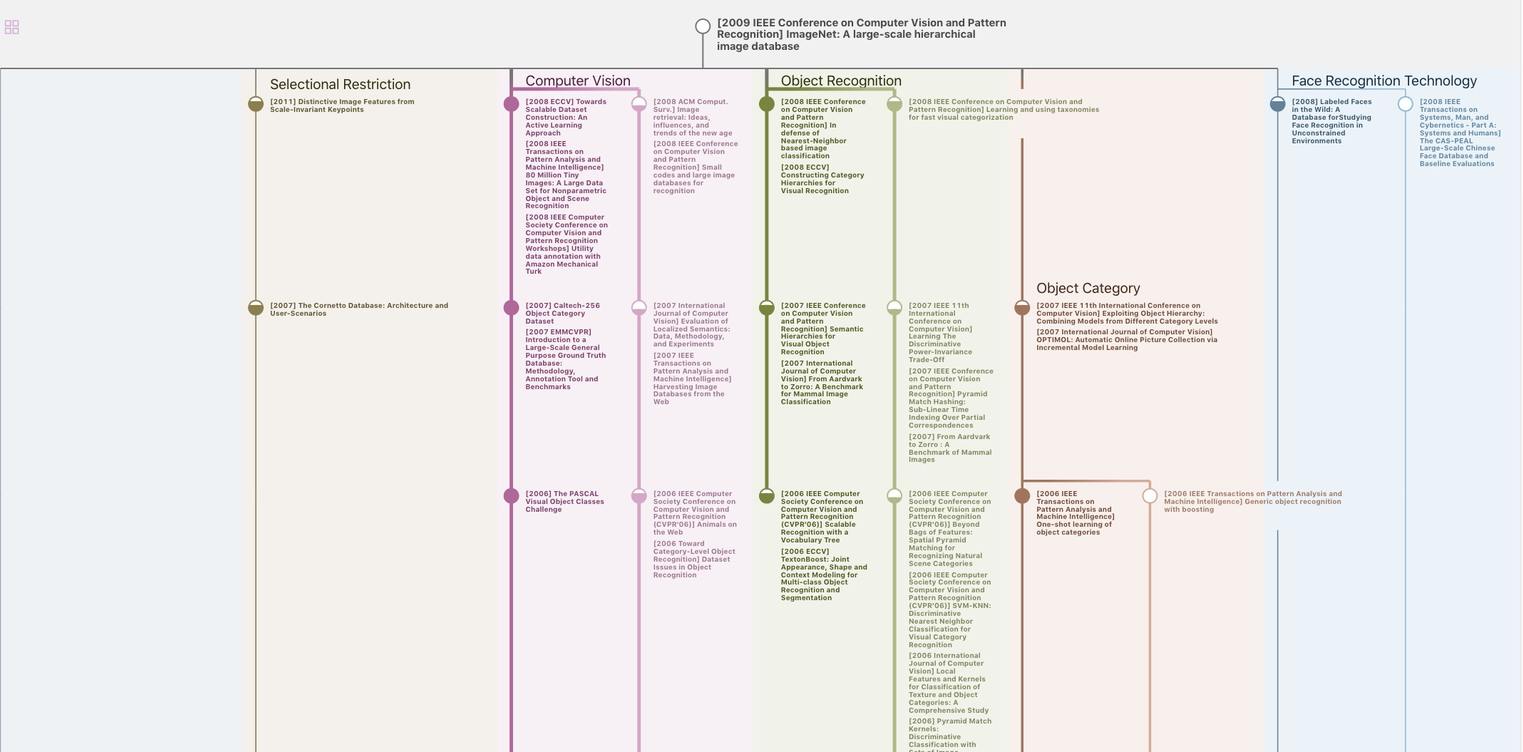
生成溯源树,研究论文发展脉络
Chat Paper
正在生成论文摘要