Methodology for the identification of relevant loci for milk traits in dairy cattle, using machine learning algorithms
MethodsX(2022)
摘要
Machine learning methods were considered efficient in identifying single nucleotide polymorphisms (SNP) underlying a trait of interest. This study aimed to construct predictive models using machine learning algorithms, to identify loci that best explain the variance in milk traits of dairy cattle. Further objectives involved validating the results by comparison with reported relevant regions and retrieving the pathways overrepresented by the genes flanking relevant SNPs. Regression models using XGBoost (XGB), LightGBM (LGB), and Random Forest (RF) algorithms were trained using estimated breeding values for milk production (EBVM), milk fat content (EBVF) and milk protein content (EBVP) as phenotypes and genotypes on 40417 SNPs as predictor variables. To evaluate their efficiency, metrics for actual vs. predicted values were determined in validation folds (XGB and LGB) and out-of-bag data (RF). Less than 4500 relevant SNPs were retrieved for each trait. Among the genes flanking them, signaling and transmembrane transporter activities were overrepresented.
更多查看译文
关键词
Single nucleotide polymorphisms,Estimated breeding values,Dairy cattle,XGBoost,LightGBM,Random forest,Milk production,Milk fat content,Milk protein content
AI 理解论文
溯源树
样例
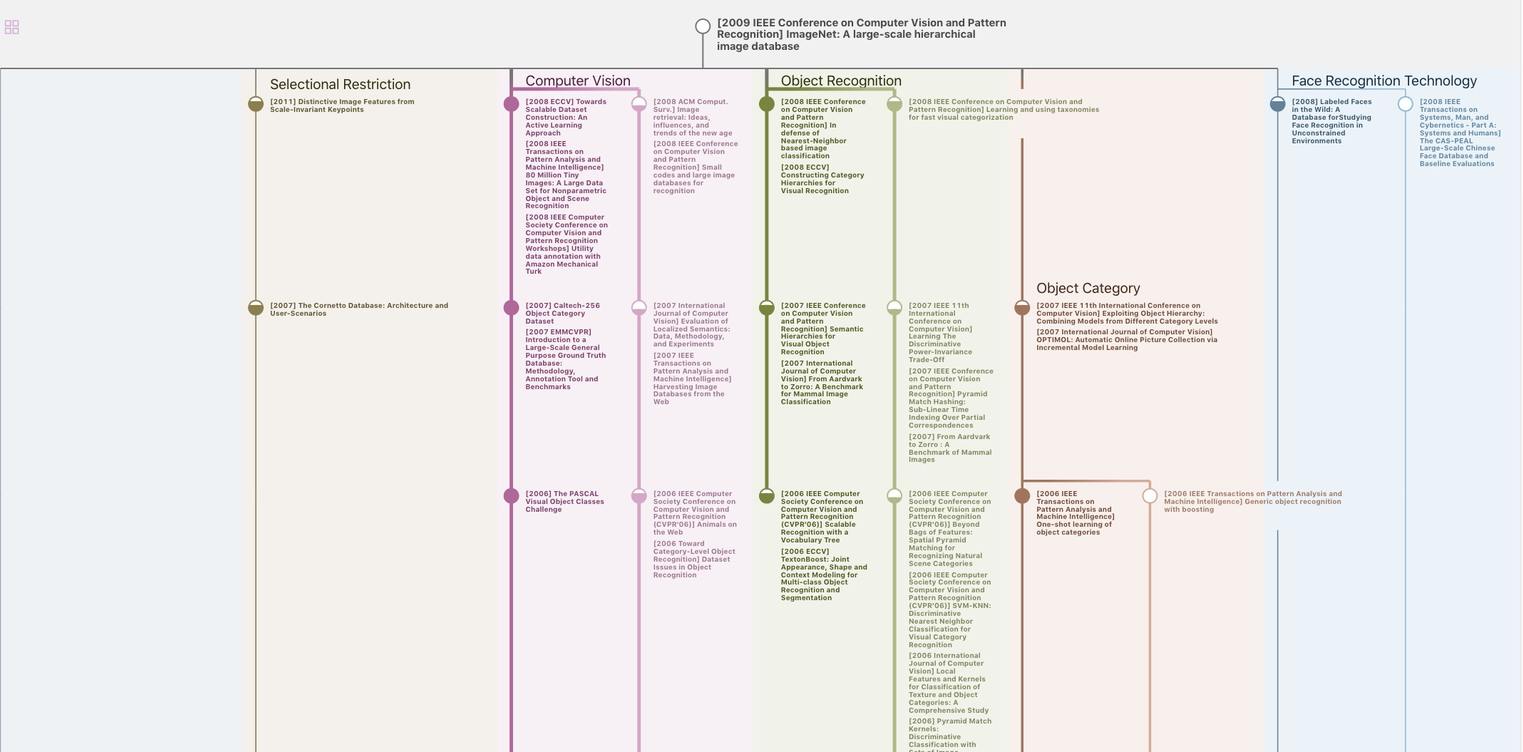
生成溯源树,研究论文发展脉络
Chat Paper
正在生成论文摘要