Domain Adaptation With Self-Guided Adaptive Sampling Strategy: Feature Alignment for Cross-User Myoelectric Pattern Recognition
IEEE TRANSACTIONS ON NEURAL SYSTEMS AND REHABILITATION ENGINEERING(2022)
摘要
Gestural interfaces based on surface electromyographic (sEMG) signal have been widely explored. Nevertheless, due to the individual differences in the sEMG signals, it is very challenging for a myoelectric pattern recognition control system to adapt cross-user variability. Unsupervised domain adaptation (UDA) has achieved unprecedented success in improving the cross-domain robustness, and it is a promising approach to solve the cross-user challenge. Existing UDA methods largely ignore the instantaneous data distribution during model updating, thus deteriorating the feature representation given a large domain shift. To address this issue, a novel method is proposed based on a UDA model incorporated with a self-guided adaptive sampling (SGAS) strategy. This strategy is designed to utilize the domain distance in a kernel space as an indicator to screen out reliable instantaneous samples for updating the classifier. Thus, it enables improved alignment of feature representations of myoelectric patterns across users. To evaluate the performance of the proposed method, sEMG data were recorded from forearm muscles of nine subjects performing six finger and wrist gestures. Experiment results show that the UDA method with the SGAS strategy achieved a mean accuracy of 90.41% +/- 14.44% in a cross-user classification manner, outperformed the state-of-the-art methods with statistical significance (p < 0.05). This study demonstrates the effectiveness of the proposed UDA framework and offers a novel tool for implementing cross-user myoelectric pattern recognition towards a multi-user and user-independent control.
更多查看译文
关键词
Electromyography (EMG), domain adaptation, cross-user variability, myoelectric control, gestural interfaces
AI 理解论文
溯源树
样例
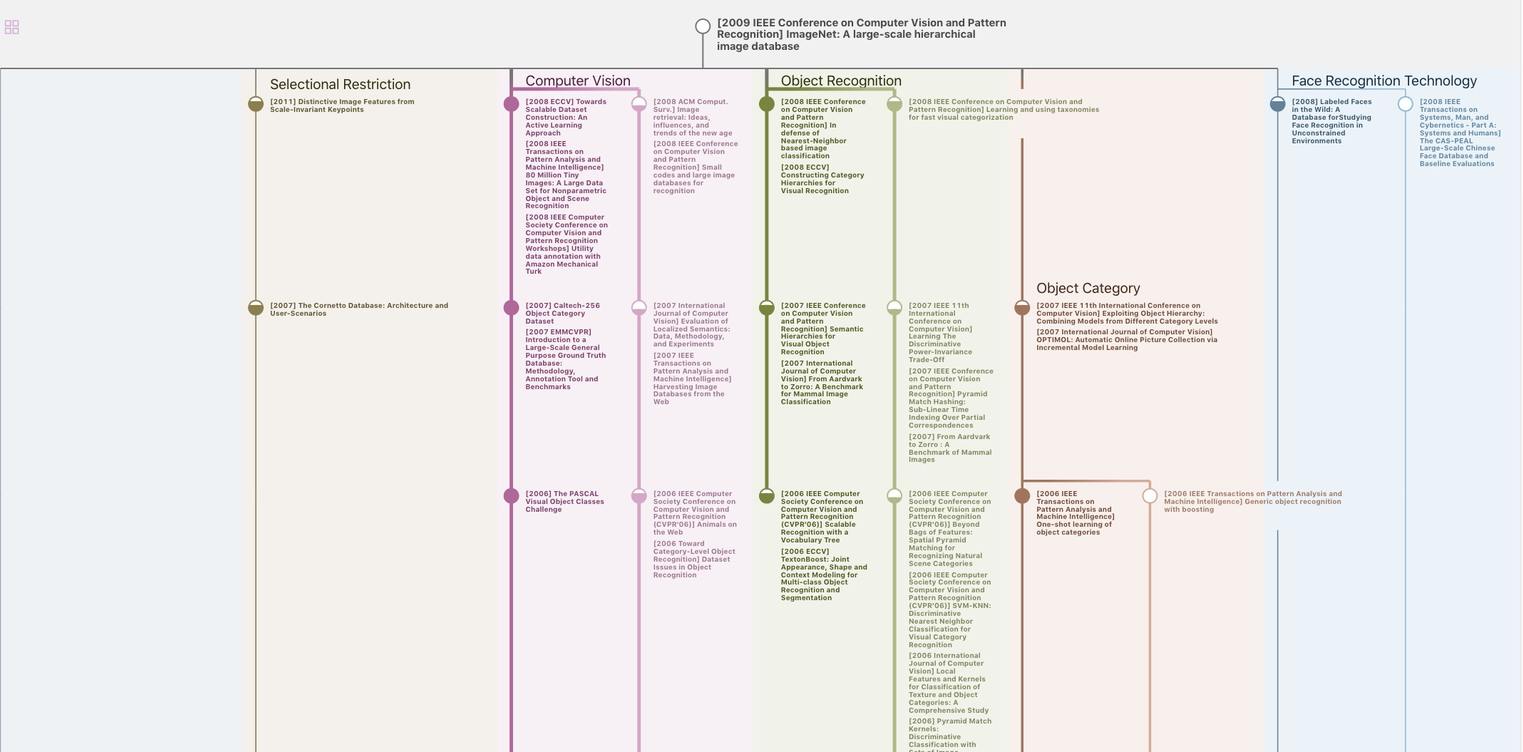
生成溯源树,研究论文发展脉络
Chat Paper
正在生成论文摘要