Convergence of Stein Variational Gradient Descent under a Weaker Smoothness Condition
arxiv(2022)
摘要
Stein Variational Gradient Descent (SVGD) is an important alternative to the Langevin-type algorithms for sampling from probability distributions of the form $\pi(x) \propto \exp(-V(x))$. In the existing theory of Langevin-type algorithms and SVGD, the potential function $V$ is often assumed to be $L$-smooth. However, this restrictive condition excludes a large class of potential functions such as polynomials of degree greater than $2$. Our paper studies the convergence of the SVGD algorithm for distributions with $(L_0,L_1)$-smooth potentials. This relaxed smoothness assumption was introduced by Zhang et al. [2019a] for the analysis of gradient clipping algorithms. With the help of trajectory-independent auxiliary conditions, we provide a descent lemma establishing that the algorithm decreases the $\mathrm{KL}$ divergence at each iteration and prove a complexity bound for SVGD in the population limit in terms of the Stein Fisher information.
更多查看译文
关键词
stein variational gradient descent,smoothness condition,convergence
AI 理解论文
溯源树
样例
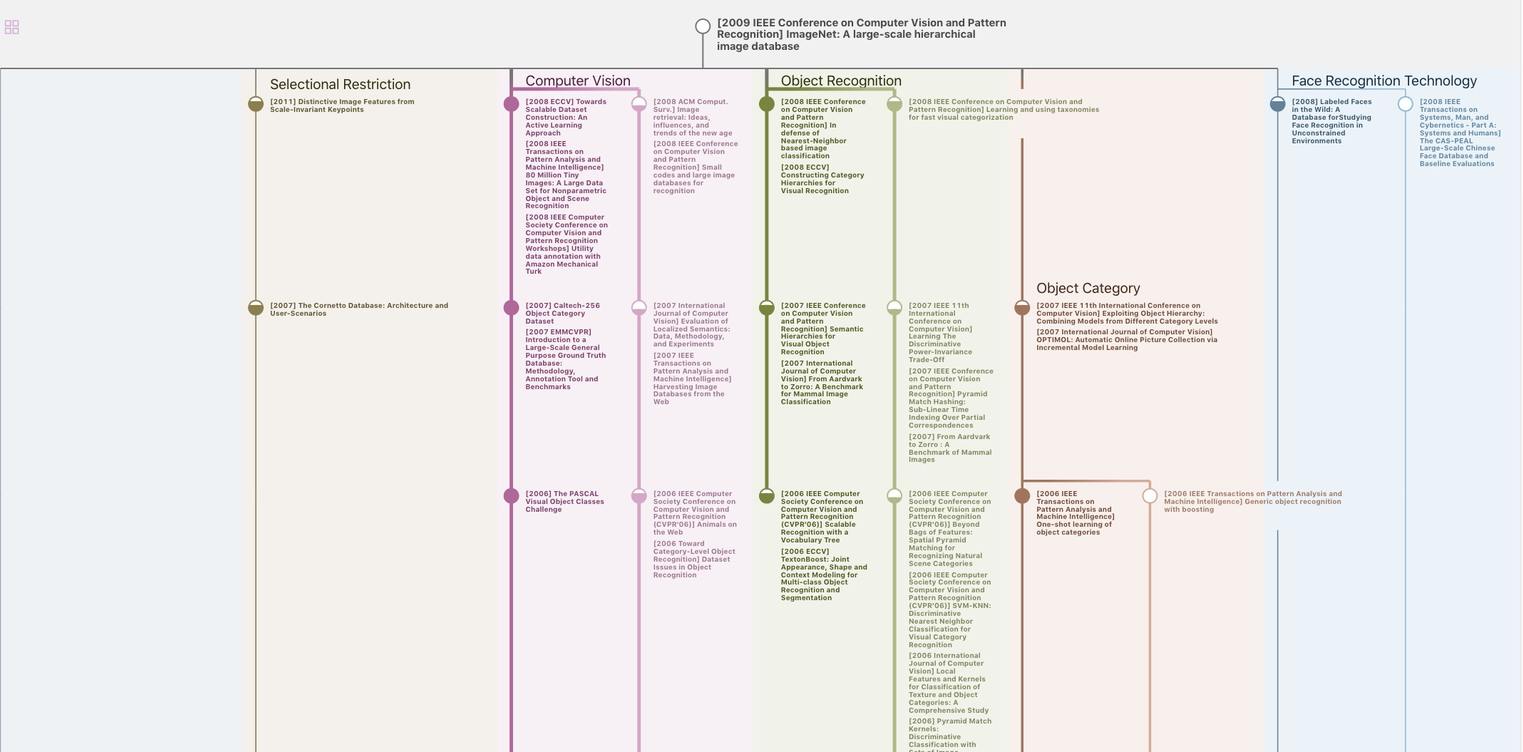
生成溯源树,研究论文发展脉络
Chat Paper
正在生成论文摘要