On Gap-dependent Bounds for Offline Reinforcement Learning
NeurIPS 2022(2022)
摘要
This paper presents a systematic study on gap-dependent sample complexity in offline reinforcement learning. Prior work showed when the density ratio between an optimal policy and the behavior policy is upper bounded (the optimal policy coverage assumption), then the agent can achieve an $O\left(\frac{1}{\epsilon^2}\right)$ rate, which is also minimax optimal. We show under the optimal policy coverage assumption, the rate can be improved to $O\left(\frac{1}{\epsilon}\right)$ when there is a positive sub-optimality gap in the optimal $Q$-function. Furthermore, we show when the visitation probabilities of the behavior policy are uniformly lower bounded for states where an optimal policy's visitation probabilities are positive (the uniform optimal policy coverage assumption), the sample complexity of identifying an optimal policy is independent of $\frac{1}{\epsilon}$. Lastly, we present nearly-matching lower bounds to complement our gap-dependent upper bounds.
更多查看译文
关键词
offline reinforcement learning,gap-dependent
AI 理解论文
溯源树
样例
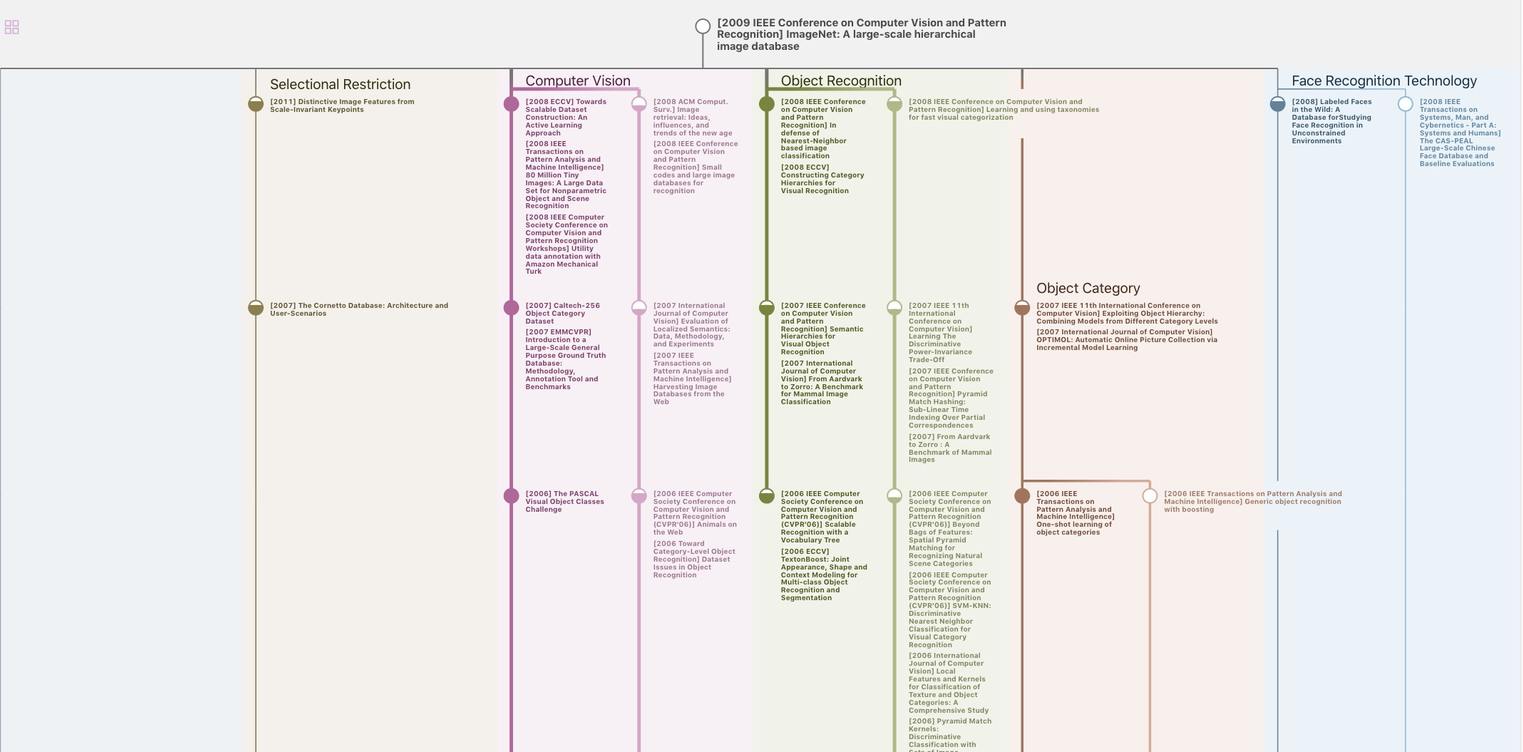
生成溯源树,研究论文发展脉络
Chat Paper
正在生成论文摘要