A Theoretical Framework for Inference Learning
NeurIPS 2022(2022)
摘要
Backpropagation (BP) is the most successful and widely used algorithm in deep learning. However, the computations required by BP are challenging to reconcile with known neurobiology. This difficulty has stimulated interest in more biologically plausible alternatives to BP. One such algorithm is the inference learning algorithm (IL). IL has close connections to neurobiological models of cortical function and has achieved equal performance to BP on supervised learning and auto-associative tasks. In contrast to BP, however, the mathematical foundations of IL are not well-understood. Here, we develop a novel theoretical framework for IL. Our main result is that IL closely approximates an optimization method known as implicit stochastic gradient descent (implicit SGD), which is distinct from the explicit SGD implemented by BP. Our results further show how the standard implementation of IL can be altered to better approximate implicit SGD. Our novel implementation considerably improves the stability of IL across learning rates, which is consistent with our theory, as a key property of implicit SGD is its stability. We provide extensive simulation results that further support our theoretical interpretations and also demonstrate IL achieves quicker convergence when trained with small mini-batches while matching the performance of BP for large mini-batches.
更多查看译文
关键词
Predictive Coding,Backpropagation,Synaptic Plasticity,Local Learning,Inference Learning
AI 理解论文
溯源树
样例
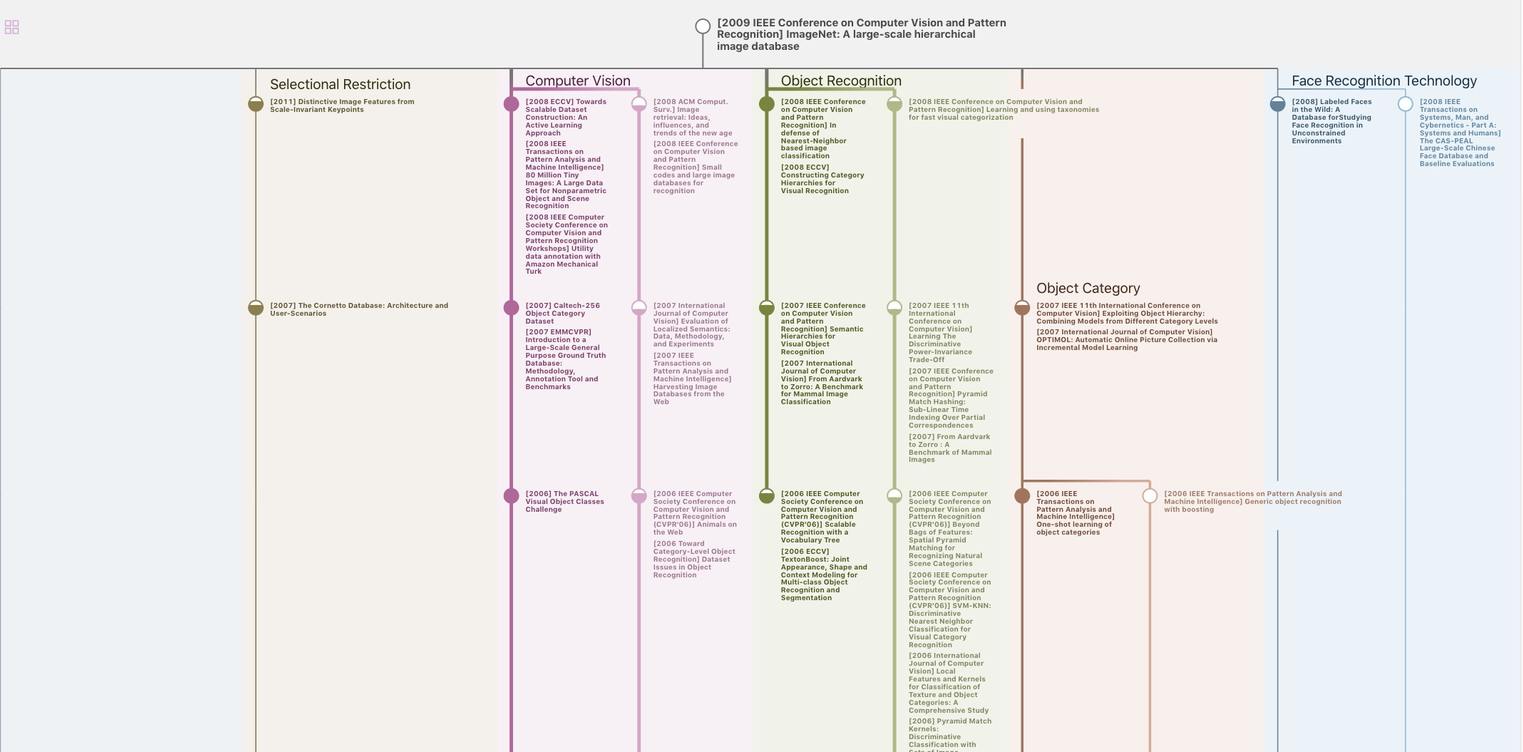
生成溯源树,研究论文发展脉络
Chat Paper
正在生成论文摘要