Semantic Graph Attention With Explicit Anatomical Association Modeling for Tooth Segmentation From CBCT Images
IEEE Transactions on Medical Imaging(2022)
摘要
Accurate tooth identification and delineation in dental CBCT images are essential in clinical oral diagnosis and treatment. Teeth are positioned in the alveolar bone in a particular order, featuring similar appearances across adjacent and bilaterally symmetric teeth. However, existing tooth segmentation methods ignored such specific anatomical topology, which hampers the segmentation accuracy. Here we propose a semantic graph-based method to explicitly model the spatial associations between different anatomical targets (i.e., teeth) for their precise delineation in a coarse-to-fine fashion. First, to efficiently control the bilaterally symmetric confusion in segmentation, we employ a lightweight network to roughly separate teeth as four quadrants. Then, designing a semantic graph attention mechanism to explicitly model the anatomical topology of the teeth in each quadrant, based on which voxel-wise discriminative feature embeddings are learned for the accurate delineation of teeth boundaries. Extensive experiments on a clinical dental CBCT dataset demonstrate the superior performance of the proposed method compared with other state-of-the-art approaches.
更多查看译文
关键词
Semantics,Spiral Cone-Beam Computed Tomography,Tooth,Image Processing, Computer-Assisted
AI 理解论文
溯源树
样例
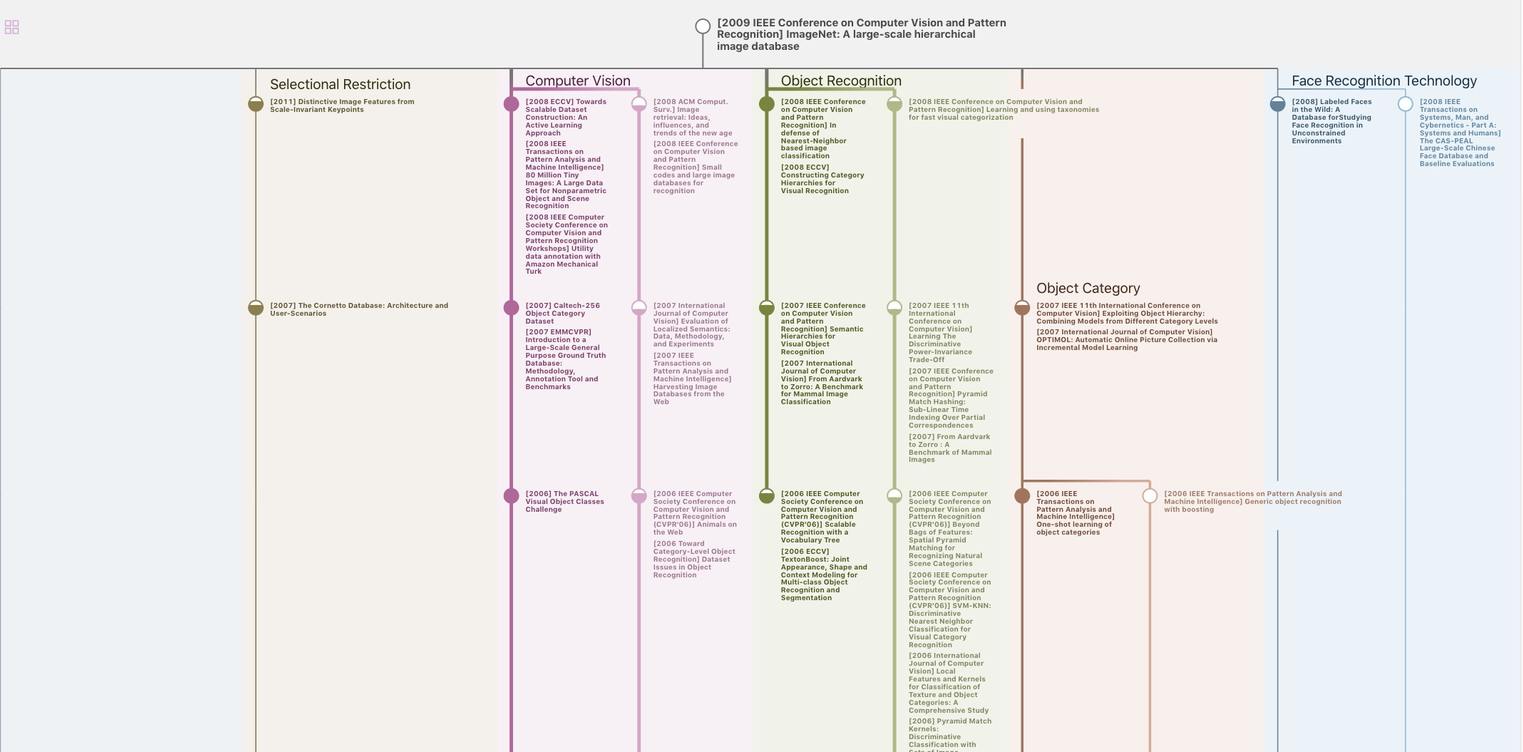
生成溯源树,研究论文发展脉络
Chat Paper
正在生成论文摘要