Graph Backup: Data Efficient Backup Exploiting Markovian Transitions
ICLR 2023(2022)
摘要
The successes of deep Reinforcement Learning (RL) are limited to settings where we have a large stream of online experiences, but applying RL in the data-efficient setting with limited access to online interactions is still challenging. A key to data-efficient RL is good value estimation, but current methods in this space fail to fully utilise the structure of the trajectory data gathered from the environment. In this paper, we treat the transition data of the MDP as a graph, and define a novel backup operator, Graph Backup, which exploits this graph structure for better value estimation. Compared to multi-step backup methods such as $n$-step $Q$-Learning and TD($\lambda$), Graph Backup can perform counterfactual credit assignment and gives stable value estimates for a state regardless of which trajectory the state is sampled from. Our method, when combined with popular value-based methods, provides improved performance over one-step and multi-step methods on a suite of data-efficient RL benchmarks including MiniGrid, Minatar and Atari100K. We further analyse the reasons for this performance boost through a novel visualisation of the transition graphs of Atari games.
更多查看译文
关键词
reinforcement learning,graph structure,neuro-symbolic methods,data efficient reinforcement learning
AI 理解论文
溯源树
样例
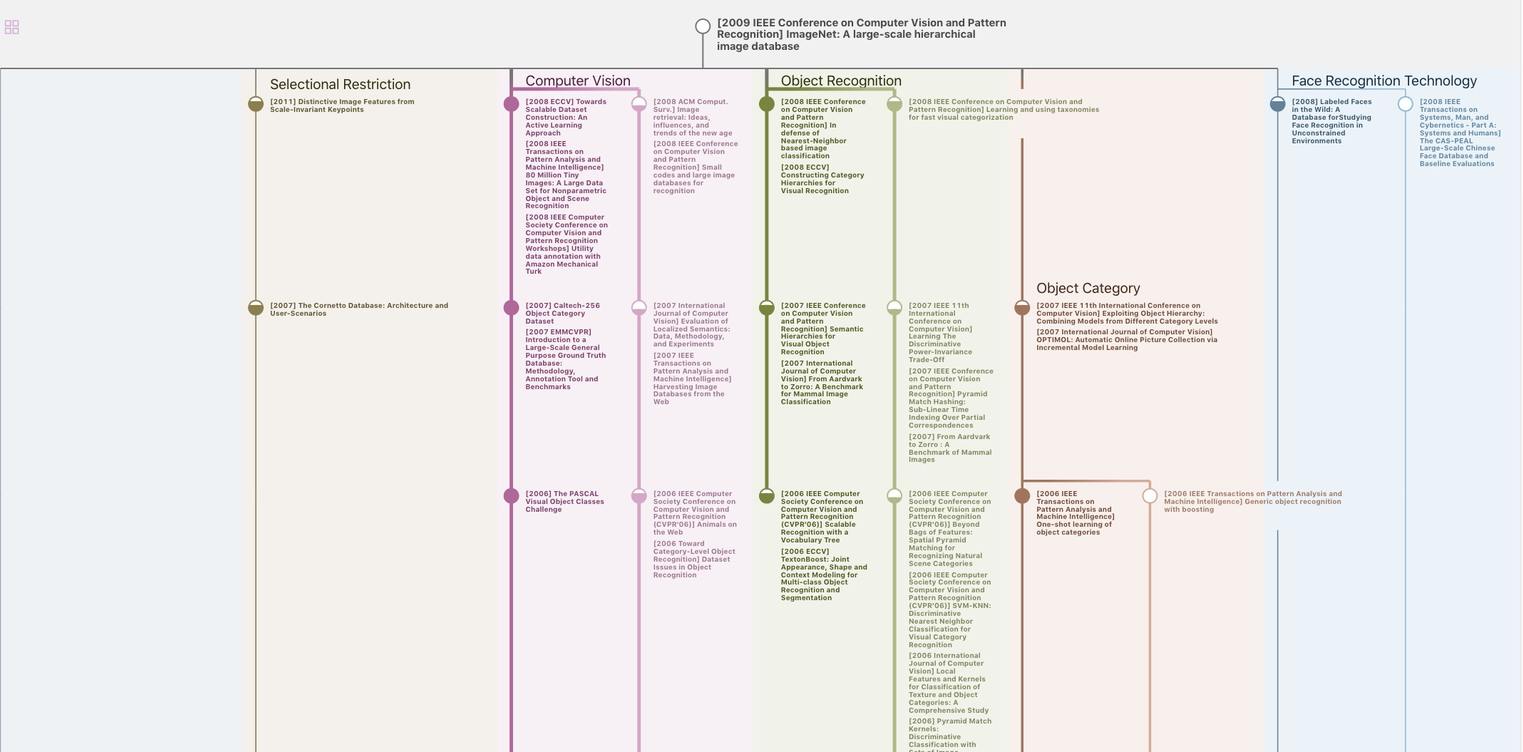
生成溯源树,研究论文发展脉络
Chat Paper
正在生成论文摘要