Contrasting Quadratic Assignments for Set-Based Representation Learning.
European Conference on Computer Vision(2022)
摘要
The standard approach to contrastive learning is to maximize the agreement between different views of the data. The views are ordered in pairs, such that they are either positive, encoding different views of the same object, or negative, corresponding to views of different objects. The supervisory signal comes from maximizing the total similarity over positive pairs, while the negative pairs are needed to avoid collapse. In this work, we note that the approach of considering individual pairs cannot account for both intra-set and inter-set similarities when the sets are formed from the views of the data. It thus limits the information content of the supervisory signal available to train representations. We propose to go beyond contrasting individual pairs of objects by focusing on contrasting objects as sets. For this, we use combinatorial quadratic assignment theory designed to evaluate set and graph similarities and derive set-contrastive objective as a regularizer for contrastive learning methods. We conduct experiments and demonstrate that our method improves learned representations for the tasks of metric learning and self-supervised classification.
更多查看译文
关键词
Contrastive learning,Representation learning,Self-supervised,Optimal assignment
AI 理解论文
溯源树
样例
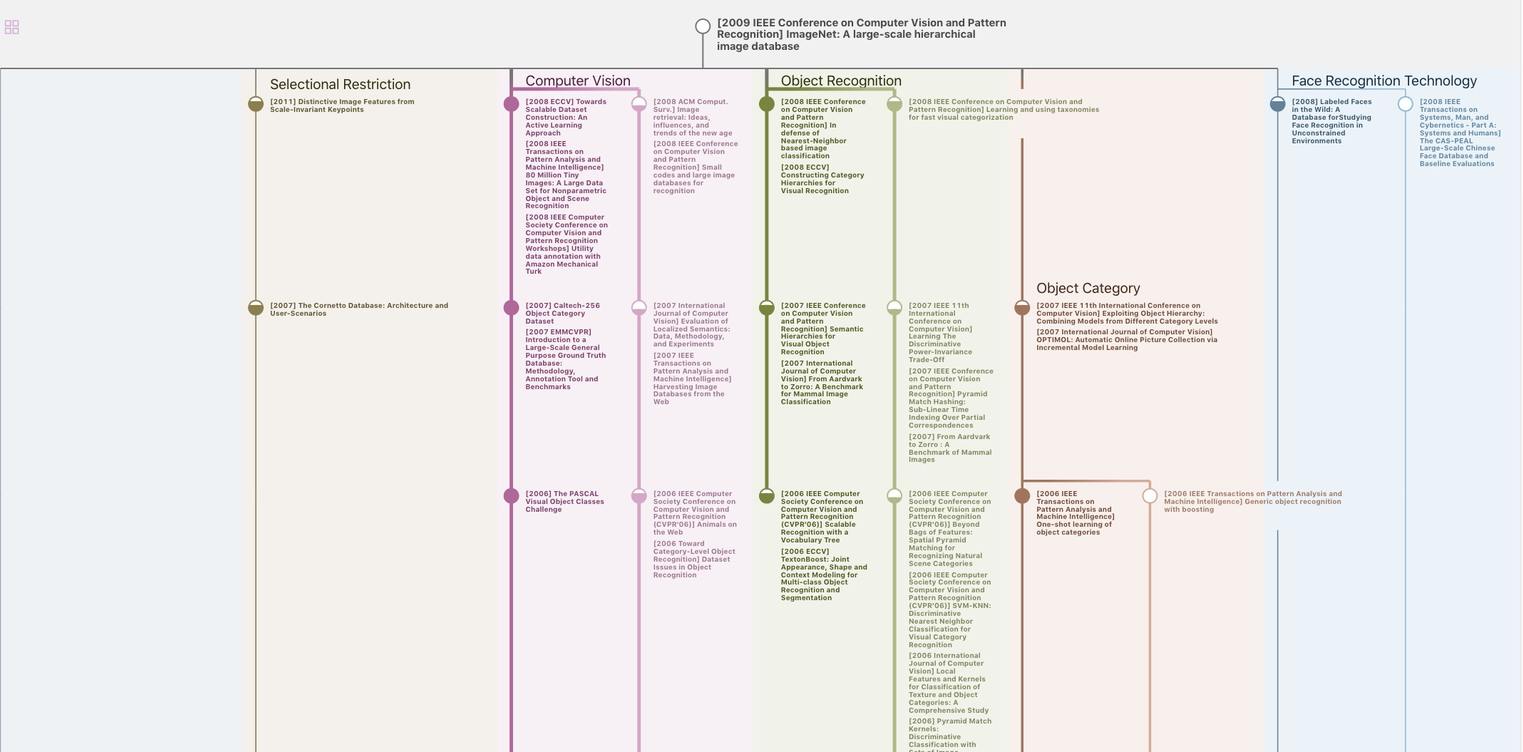
生成溯源树,研究论文发展脉络
Chat Paper
正在生成论文摘要