Hierarchies of Reward Machines
ICLR 2023(2022)
摘要
Reward machines (RMs) are a recent formalism for representing the reward function of a reinforcement learning task through a finite-state machine whose edges encode landmarks of the task using high-level events. The structure of RMs enables the decomposition of a task into simpler and independently solvable subtasks that help tackle long-horizon and/or sparse reward tasks. We propose a formalism for further abstracting the subtask structure by endowing an RM with the ability to call other RMs, thus composing a hierarchy of RMs (HRM). We exploit HRMs by treating each call to an RM as an independently solvable subtask using the options framework, and describe a curriculum-based method to induce HRMs from example traces observed by the agent. Our experiments reveal that exploiting a handcrafted HRM leads to faster convergence than with a flat HRM, and that learning an HRM is more scalable than learning an equivalent flat HRM.
更多查看译文
关键词
Hierarchical Reinforcement Learning,Reward Machines
AI 理解论文
溯源树
样例
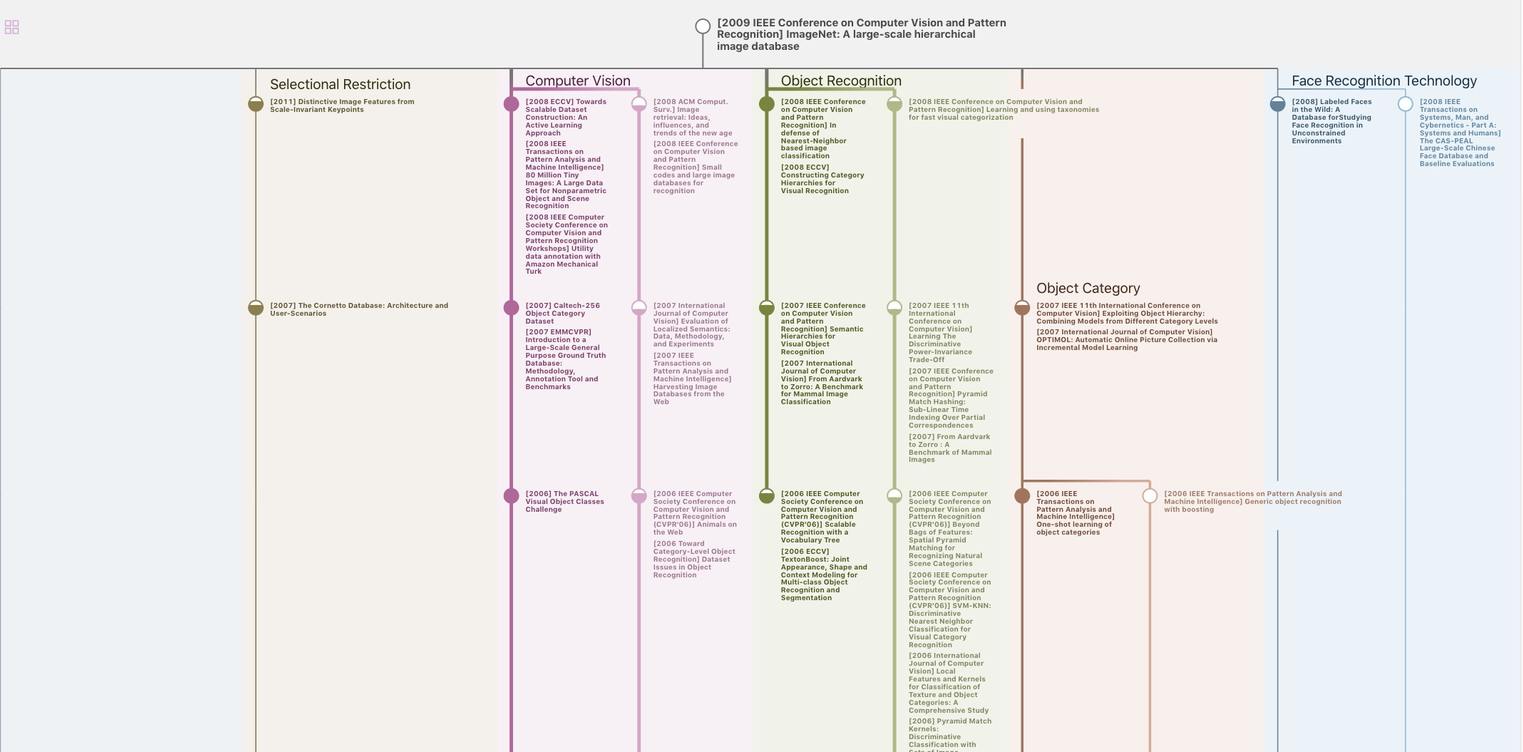
生成溯源树,研究论文发展脉络
Chat Paper
正在生成论文摘要