Multi-Scan Multi-Sensor Multi-Object State Estimation.
IEEE Trans. Signal Process.(2022)
摘要
If computational tractability were not an issue, multi-object estimation should integrate all measurements from multiple sensors across multiple scans. In this article, we propose an efficient numerical solution to the multi-scan multi-sensor multi-object estimation problem by computing the (labeled) multi-sensor multi-object posterior density. Minimizing the $L_{1}$-norm error from the exact posterior density requires solving large-scale multi-dimensional assignment problems that are NP-hard. An efficient multi-dimensional assignment algorithm is developed based on Gibbs sampling, together with convergence analysis. The resulting multi-scan multi-sensor multi-object estimation algorithm can be applied either offline in one batch or recursively. The efficacy of the algorithm is demonstrated using numerical experiments with a simulated dataset.
更多查看译文
关键词
State estimation,Smoothing,Random finite sets,Multi-sensor,Multi-dimensional assignment,Gibbs sampling
AI 理解论文
溯源树
样例
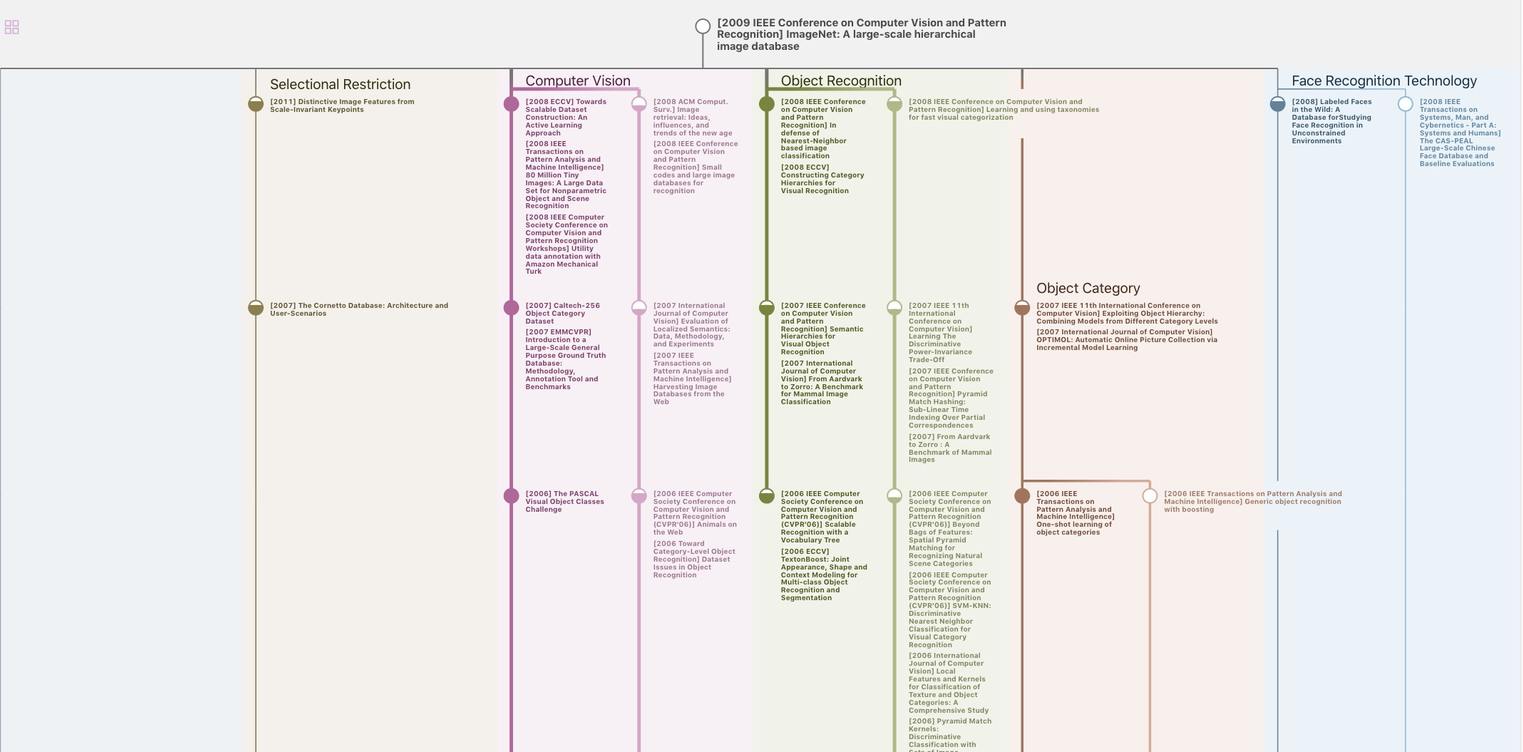
生成溯源树,研究论文发展脉络
Chat Paper
正在生成论文摘要