Attention Flows for General Transformers
ICLR 2023(2022)
摘要
In this paper, we study the computation of how much an input token in a Transformer model influences its prediction. We formalize a method to construct a flow network out of the attention values of encoder-only Transformer models and extend it to general Transformer architectures including an auto-regressive decoder. We show that running a maxflow algorithm on the flow network construction yields Shapley values, which determine the impact of a player in cooperative game theory. By interpreting the input tokens in the flow network as players, we can compute their influence on the total attention flow leading to the decoder's decision. Additionally, we provide a library that computes and visualizes the attention flow of arbitrary Transformer models. We show the usefulness of our implementation on various models trained on natural language processing and reasoning tasks.
更多查看译文
关键词
transformer,explanations,attention flow,shapley value
AI 理解论文
溯源树
样例
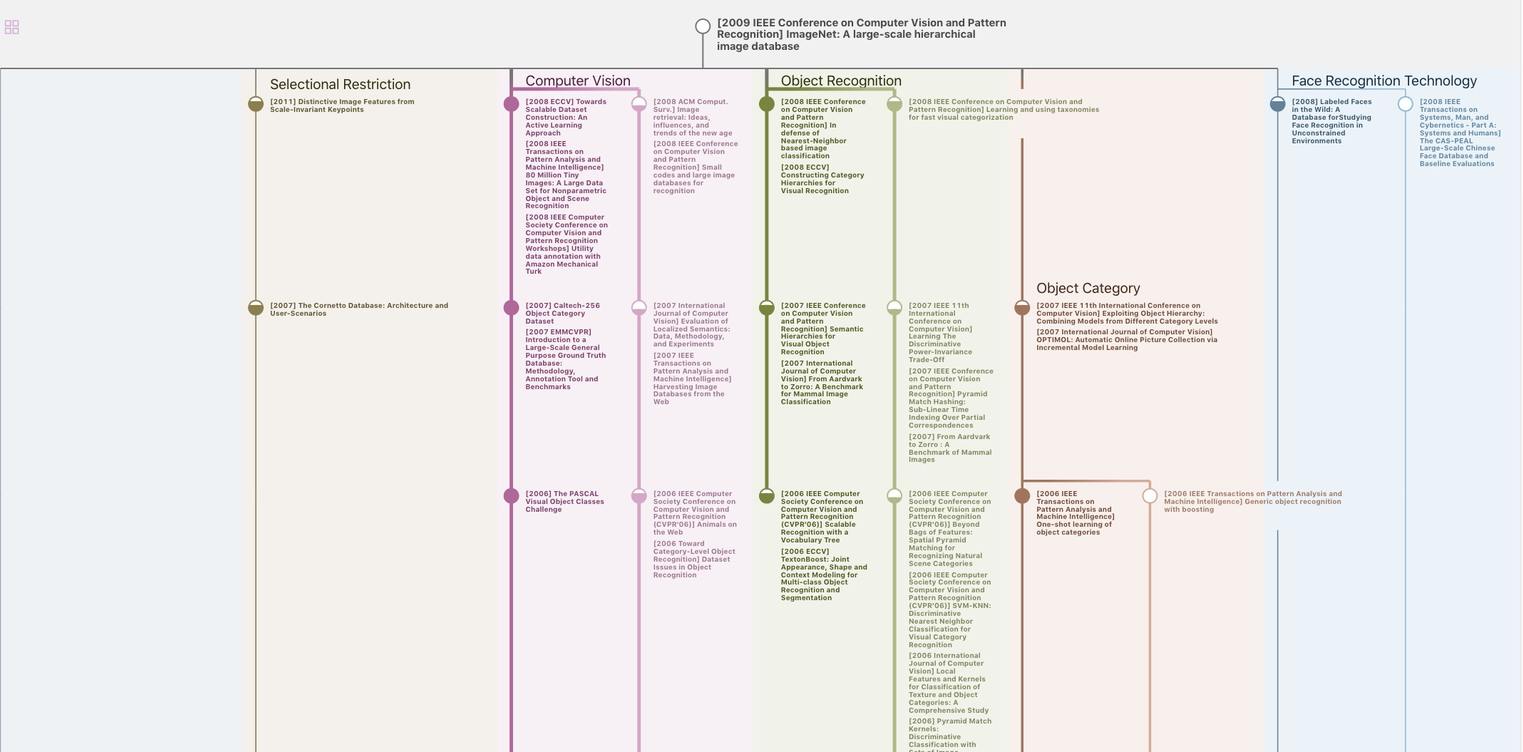
生成溯源树,研究论文发展脉络
Chat Paper
正在生成论文摘要