Associative learning mechanism for drug-target interaction prediction
CAAI TRANSACTIONS ON INTELLIGENCE TECHNOLOGY(2023)
摘要
As a necessary process of modern drug development, finding a drug compound that can selectively bind to a specific protein is highly challenging and costly. Exploring drug-target interaction strength in terms of drug-target affinity (DTA) is an emerging and effective research approach for drug development. However, it is challenging to model drug-target interactions in a deep learning manner, and few studies provide interpretable analysis of models. This paper proposes a DTA prediction method (mutual transformer-drug target affinity [MT-DTA]) with interactive learning and an autoencoder mechanism. The proposed MT-DTA builds a variational autoencoders system with a cascade structure of the attention model and convolutional neural networks. It not only enhances the ability to capture the characteristic information of a single molecular sequence but also establishes the characteristic expression relationship for each substructure in a single molecular sequence. On this basis, a molecular information interaction module is constructed, which adds information interaction paths between molecular sequence pairs and complements the expression of correlations between molecular substructures. The performance of the proposed model was verified on two public benchmark datasets, KIBA and Davis, and the results confirm that the proposed model structure is effective in predicting DTA. Additionally, attention transformer models with different configurations can improve the feature expression of drug/protein molecules. The model performs better in correctly predicting interaction strengths compared with state-of-the-art baselines. In addition, the diversity of drug/protein molecules can be better expressed than existing methods such as SeqGAN and Co-VAE to generate more effective new drugs. The DTA value prediction module fuses the drug-target pair interaction information to output the predicted value of DTA. Additionally, this paper theoretically proves that the proposed method maximises evidence lower bound for the joint distribution of the DTA prediction model, which enhances the consistency of the probability distribution between actual and predicted values. The source code of proposed method is available at .
更多查看译文
关键词
deep learning,medical applications
AI 理解论文
溯源树
样例
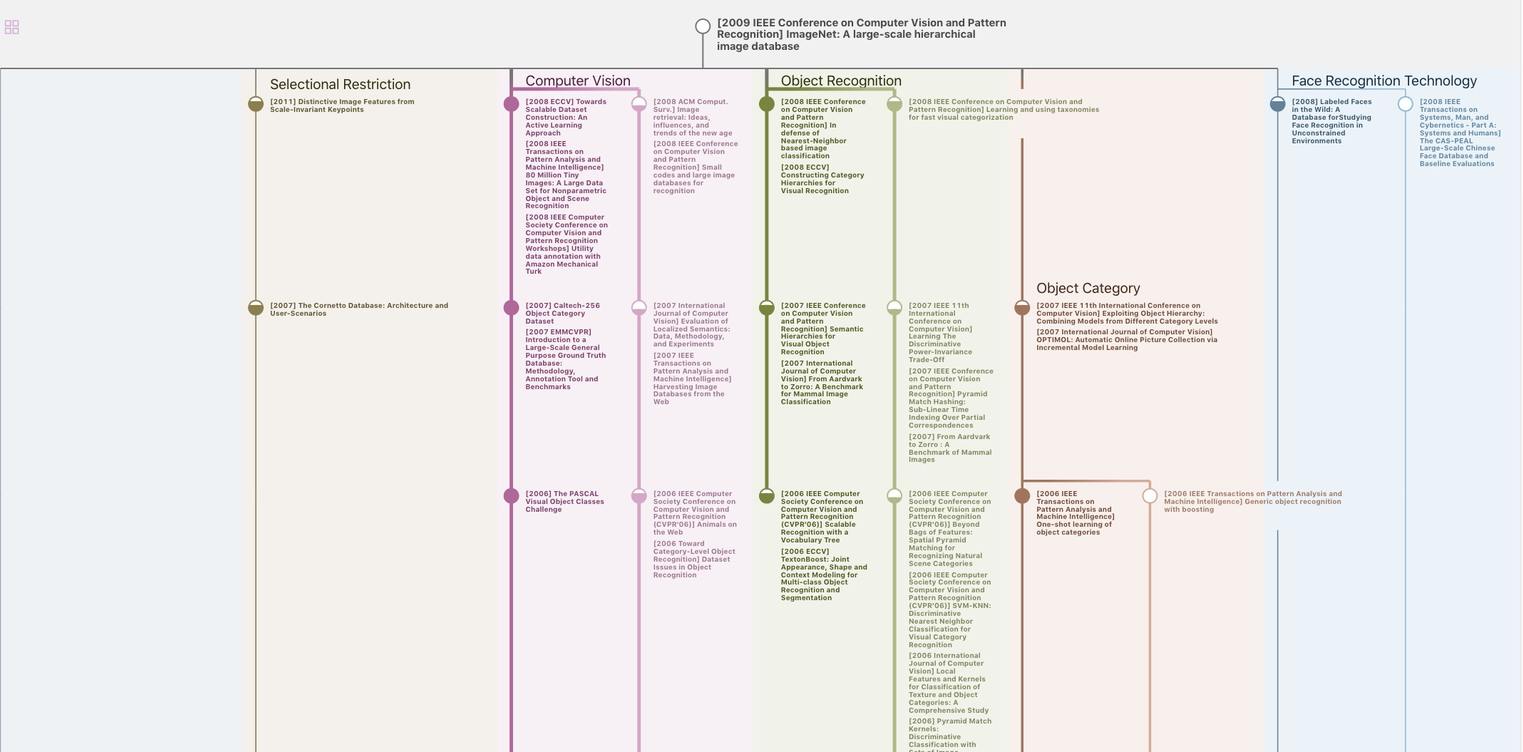
生成溯源树,研究论文发展脉络
Chat Paper
正在生成论文摘要