An Interactive Online App for Predicting Diabetes via Machine Learning from Environment-Polluting Chemical Exposure Data
INTERNATIONAL JOURNAL OF ENVIRONMENTAL RESEARCH AND PUBLIC HEALTH(2022)
摘要
The early prediction and identification of risk factors for diabetes may prevent or delay diabetes progression. In this study, we developed an interactive online application that provides the predictive probabilities of prediabetes and diabetes in 4 years based on a Bayesian network (BN) classifier, which is an interpretable machine learning technique. The BN was trained using a dataset from the Ansung cohort of the Korean Genome and Epidemiological Study (KoGES) in 2008, with a follow-up in 2012. The dataset contained not only traditional risk factors (current diabetes status, sex, age, etc.) for future diabetes, but it also contained serum biomarkers, which quantified the individual level of exposure to environment-polluting chemicals (EPC). Based on accuracy and the area under the curve (AUC), a tree-augmented BN with 11 variables derived from feature selection was used as our prediction model. The online application that implemented our BN prediction system provided a tool that performs customized diabetes prediction and allows users to simulate the effects of controlling risk factors for the future development of diabetes. The prediction results of our method demonstrated that the EPC biomarkers had interactive effects on diabetes progression and that the use of the EPC biomarkers contributed to a substantial improvement in prediction performance.
更多查看译文
关键词
diabetes mellitus, glucose intolerance, machine learning, Bayesian network, environmental pollutants
AI 理解论文
溯源树
样例
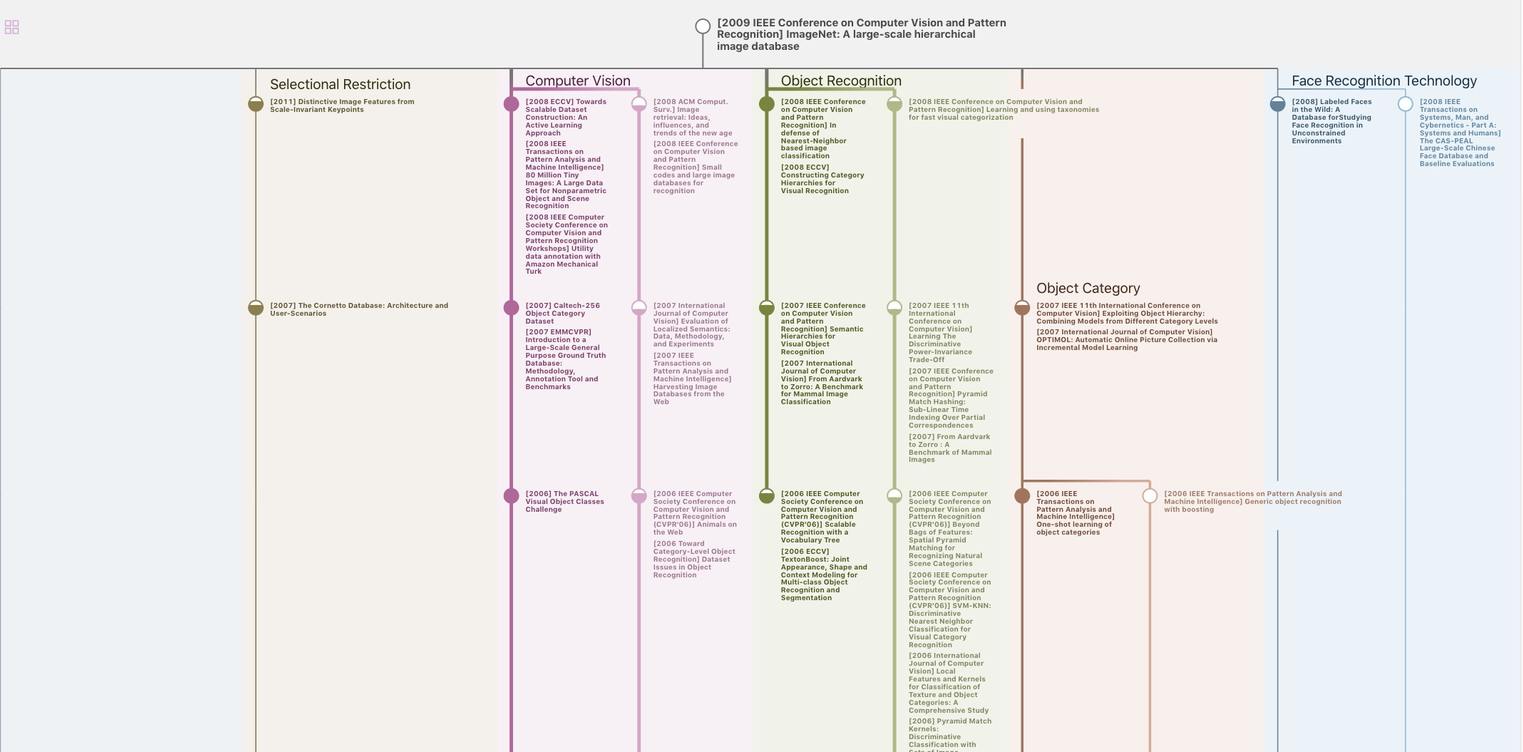
生成溯源树,研究论文发展脉络
Chat Paper
正在生成论文摘要