Multivariate Gaussian Copula Mutual Information to Estimate Functional Connectivity with Less Random Architecture
ENTROPY(2022)
摘要
Recognition of a brain region's interaction is an important field in neuroscience. Most studies use the Pearson correlation to find the interaction between the regions. According to the experimental evidence, there is a nonlinear dependence between the activities of different brain regions that is ignored by Pearson correlation as a linear measure. Typically, the average activity of each region is used as input because it is a univariate measure. This dimensional reduction, i.e., averaging, leads to a loss of spatial information across voxels within the region. In this study, we propose using an information-theoretic measure, multivariate mutual information (mvMI), as a nonlinear dependence to find the interaction between regions. This measure, which has been recently proposed, simplifies the mutual information calculation complexity using the Gaussian copula. Using simulated data, we show that the using this measure overcomes the mentioned limitations. Additionally using the real resting-state fMRI data, we compare the level of significance and randomness of graphs constructed using different methods. Our results indicate that the proposed method estimates the functional connectivity more significantly and leads to a smaller number of random connections than the common measure, Pearson correlation. Moreover, we find that the similarity of the estimated functional networks of the individuals is higher when the proposed method is used.
更多查看译文
关键词
mutual information, functional connectivity, resting-state fMRI, linear correlation
AI 理解论文
溯源树
样例
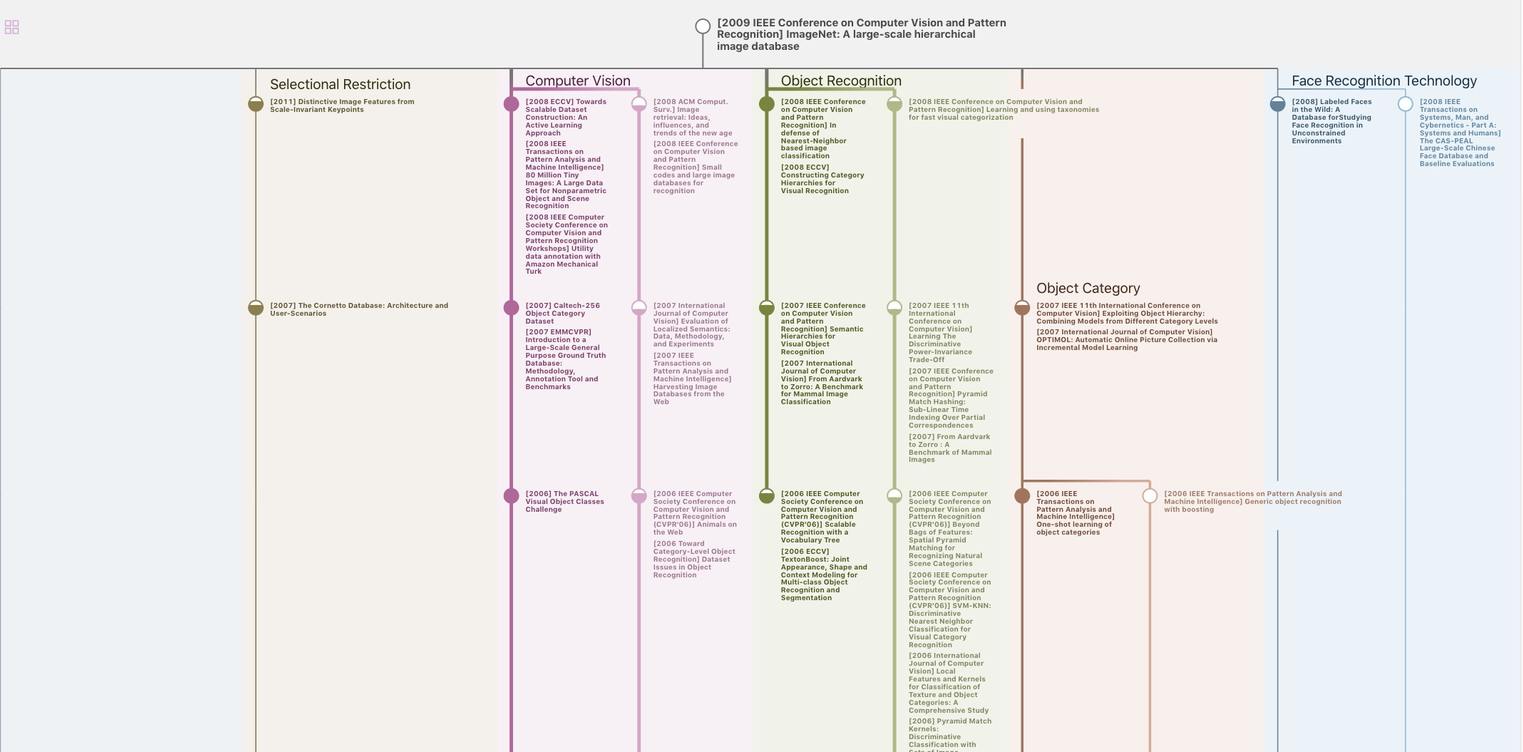
生成溯源树,研究论文发展脉络
Chat Paper
正在生成论文摘要