Random RotBoost: An Ensemble Classification Method Based on Rotation Forest and AdaBoost in Random Subsets and Its Application to Clinical Decision Support.
ENTROPY(2022)
摘要
In the era of bathing in big data, it is common to see enormous amounts of data generated daily. As for the medical industry, not only could we collect a large amount of data, but also see each data set with a great number of features. When the number of features is ramping up, a common dilemma is adding computational cost during inferring. To address this concern, the data rotational method by PCA in tree-based methods shows a path. This work tries to enhance this path by proposing an ensemble classification method with an AdaBoost mechanism in random, automatically generating rotation subsets termed Random RotBoost. The random rotation process has replaced the manual pre-defined number of subset features (free pre-defined process). Therefore, with the ensemble of the multiple AdaBoost-based classifier, overfitting problems can be avoided, thus reinforcing the robustness. In our experiments with real-world medical data sets, Random RotBoost reaches better classification performance when compared with existing methods. Thus, with the help from our proposed method, the quality of clinical decisions can potentially be enhanced and supported in medical tasks.
更多查看译文
关键词
classification, Rotation Forest, AdaBoost, clinical decision support
AI 理解论文
溯源树
样例
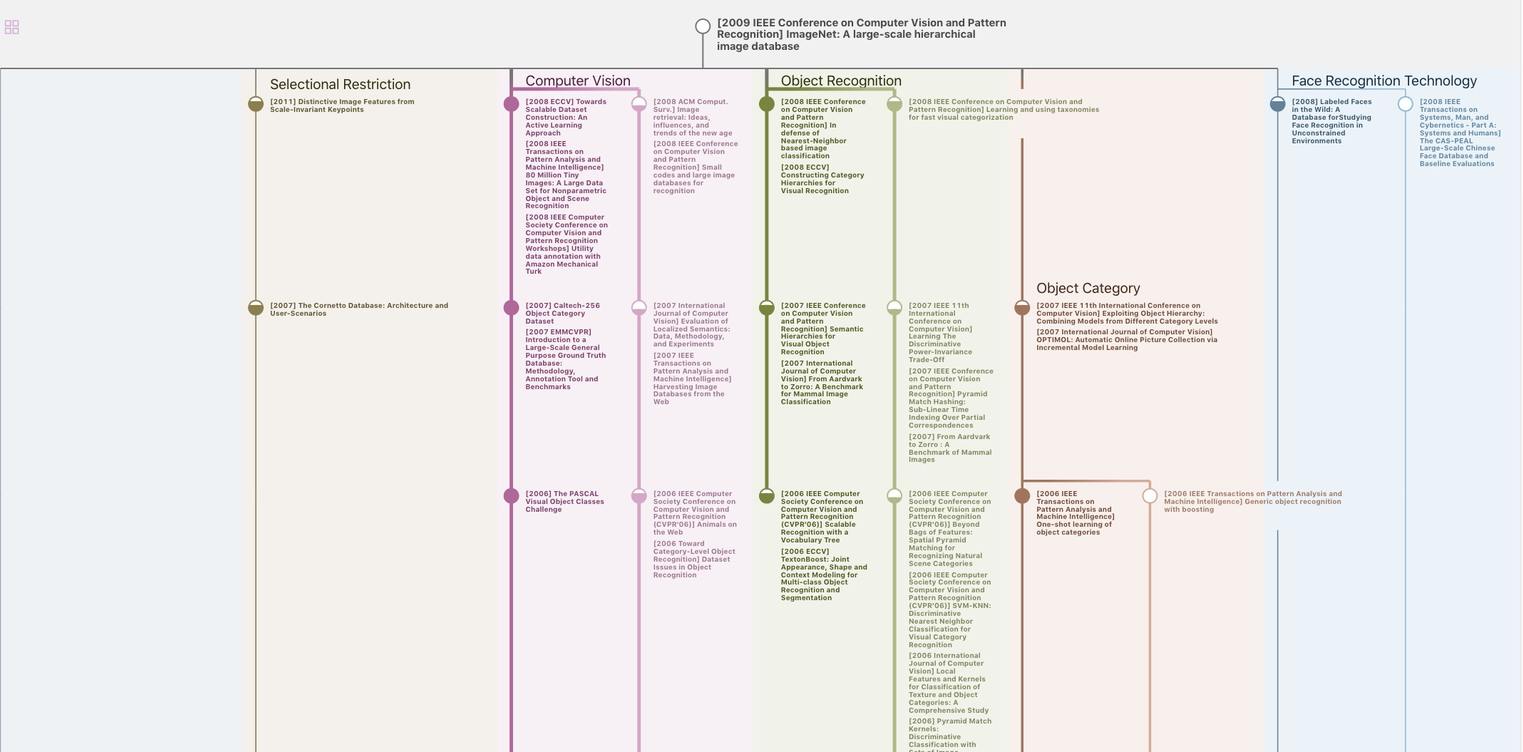
生成溯源树,研究论文发展脉络
Chat Paper
正在生成论文摘要