Generative Adversarial Network (GAN) for Automatic Reconstruction of the 3D Spine Structure by Using Simulated Bi-Planar X-ray Images
DIAGNOSTICS(2022)
摘要
In this study, we modified the previously proposed X2CT-GAN to build a 2Dto3D-GAN of the spine. This study also incorporated the radiologist's perspective in the adjustment of input signals to prove the feasibility of the automatic production of three-dimensional (3D) structures of the spine from simulated bi-planar two-dimensional (2D) X-ray images. Data from 1012 computed tomography (CT) studies of 984 patients were retrospectively collected. We tested this model under different dataset sizes (333, 666, and 1012) with different bone signal conditions to observe the training performance. A 10-fold cross-validation and five metrics-Dice similarity coefficient (DSC) value, Jaccard similarity coefficient (JSC), overlap volume (OV), and structural similarity index (SSIM)- were applied for model evaluation. The optimal mean values for DSC, JSC, OV, SSIM anteroposterior (AP), and SSIM Lateral (Lat) were 0.8192, 0.6984, 0.8624, 0.9261, and 0.9242, respectively. There was a significant improvement in the training performance under empirically enhanced bone signal conditions and with increasing training dataset sizes. These results demonstrate the potential of the clinical implantation of GAN for automatic production of 3D spine images from 2D images. This prototype model can serve as a foundation in future studies applying transfer learning for the development of advanced medical diagnostic techniques.
更多查看译文
关键词
3-dimensional (3D) spine, generative adversarial network (GAN), simulated biplanar X-ray
AI 理解论文
溯源树
样例
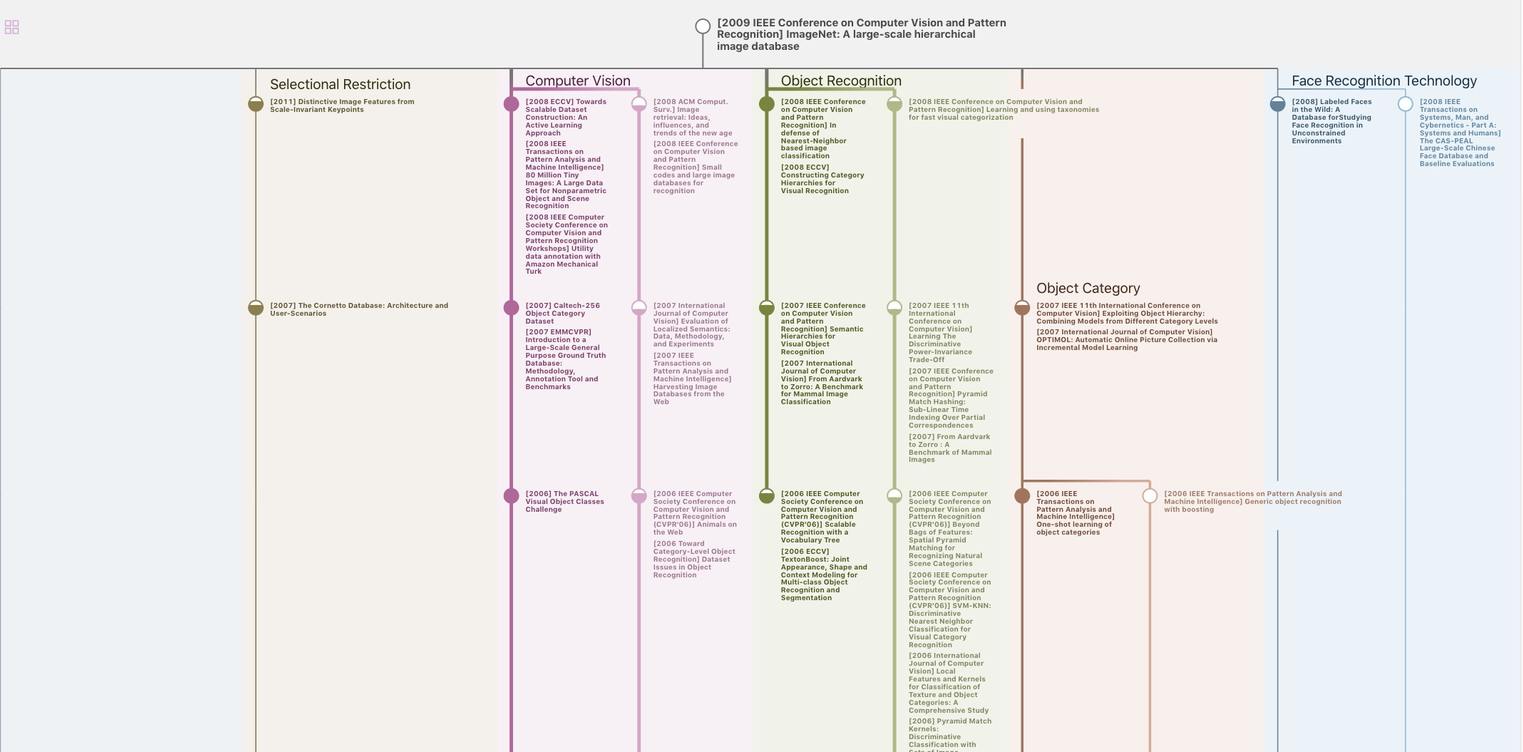
生成溯源树,研究论文发展脉络
Chat Paper
正在生成论文摘要