Parkinson's Disease Detection from Resting-State EEG Signals Using Common Spatial Pattern, Entropy, and Machine Learning Techniques
DIAGNOSTICS(2022)
摘要
Parkinson's disease (PD) is a very common brain abnormality that affects people all over the world. Early detection of such abnormality is critical in clinical diagnosis in order to prevent disease progression. Electroencephalography (EEG) is one of the most important PD diagnostic tools since this disease is linked to the brain. In this study, novel efficient common spatial pattern-based approaches for detecting Parkinson's disease in two cases, off-medication and on-medication, are proposed. First, the EEG signals are preprocessed to remove major artifacts before spatial filtering using a common spatial pattern. Several features are extracted from spatially filtered signals using different metrics, namely, variance, band power, energy, and several types of entropy. Machine learning techniques, namely, random forest, linear/quadratic discriminant analysis, support vector machine, and k-nearest neighbor, are investigated to classify the extracted features. The impacts of frequency bands, segment length, and reduction number on the results are also investigated in this work. The proposed methods are tested using two EEG datasets: the SanDiego dataset (31 participants, 93 min) and the UNM dataset (54 participants, 54 min). The results show that the proposed methods, particularly the combination of common spatial patterns and log energy entropy, provide competitive results when compared to methods in the literature. The achieved results in terms of classification accuracy, sensitivity, and specificity in the case of off-medication PD detection are around 99%. In the case of on-medication PD, the results range from 95% to 98%. The results also reveal that features extracted from the alpha and beta bands have the highest classification accuracy.
更多查看译文
关键词
common spatial pattern, discriminant analysis, electroencephalogram, entropy, k-nearest neighbor, machine learning, Parkinson's detection, random forest, support vector machines
AI 理解论文
溯源树
样例
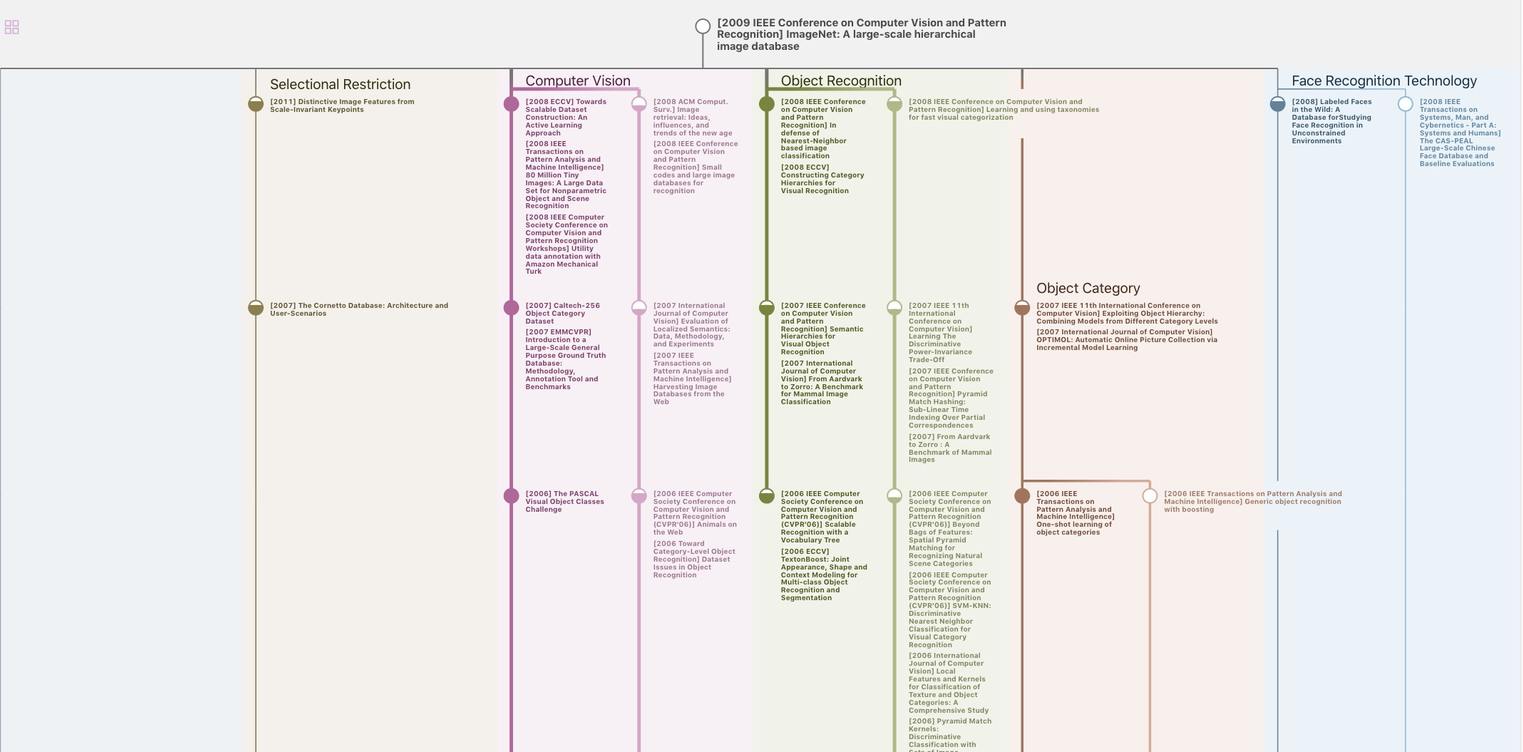
生成溯源树,研究论文发展脉络
Chat Paper
正在生成论文摘要