Deep-Learning-Based Automatic Detection and Segmentation of Brain Metastases with Small Volume for Stereotactic Ablative Radiotherapy
CANCERS(2022)
摘要
Simple Summary With advances in radiotherapy (RT) technique and more frequent use of stereotactic ablative radiotherapy (SABR), precise segmentation of all brain metastases (BM) including a small volume of BM is essential to choose an appropriate treatment modality. However, the process of detecting and manually delineating BM with small volumes often results in missing delineation and requires a great amount of labor. To address this issue, we present a useful deep learning (DL) model for the detection and segmentation of BMwith contrast-enhanced magnetic resonance images. Specifically, we applied effective training techniques to detect and segment a BM of less than 0.04 cc, which is relatively small compared to previous studies. The results of our DL model demonstrated that the proposed methods provide considerable benefit for BM, even small-volume BM, detection, and segmentation for SABR. Recently, several efforts have been made to develop the deep learning (DL) algorithms for automatic detection and segmentation of brain metastases (BM). In this study, we developed an advanced DL model to BM detection and segmentation, especially for small-volume BM. From the institutional cancer registry, contrast-enhanced magnetic resonance images of 65 patients and 603 BM were collected to train and evaluate our DL model. Of the 65 patients, 12 patients with 58 BM were assigned to test-set for performance evaluation. Ground-truth for BM was assigned to one radiation oncologist to manually delineate BM and another one to cross-check. Unlike other previous studies, our study dealt with relatively small BM, so the area occupied by the BM in the high-resolution images were small. Our study applied training techniques such as the overlapping patch technique and 2.5-dimensional (2.5D) training to the well-known U-Net architecture to learn better in smaller BM. As a DL architecture, 2D U-Net was utilized by 2.5D training. For better efficacy and accuracy of a two-dimensional U-Net, we applied effective preprocessing include 2.5D overlapping patch technique. The sensitivity and average false positive rate were measured as detection performance, and their values were 97% and 1.25 per patient, respectively. The dice coefficient with dilation and 95% Hausdorff distance were measured as segmentation performance, and their values were 75% and 2.057 mm, respectively. Our DL model can detect and segment BM with small volume with good performance. Our model provides considerable benefit for clinicians with automatic detection and segmentation of BM for stereotactic ablative radiotherapy.
更多查看译文
关键词
brain metastases, magnetic resonance imaging, autosegmentation, deep learning, convolutional neural network, stereotactic ablative radiotherapy
AI 理解论文
溯源树
样例
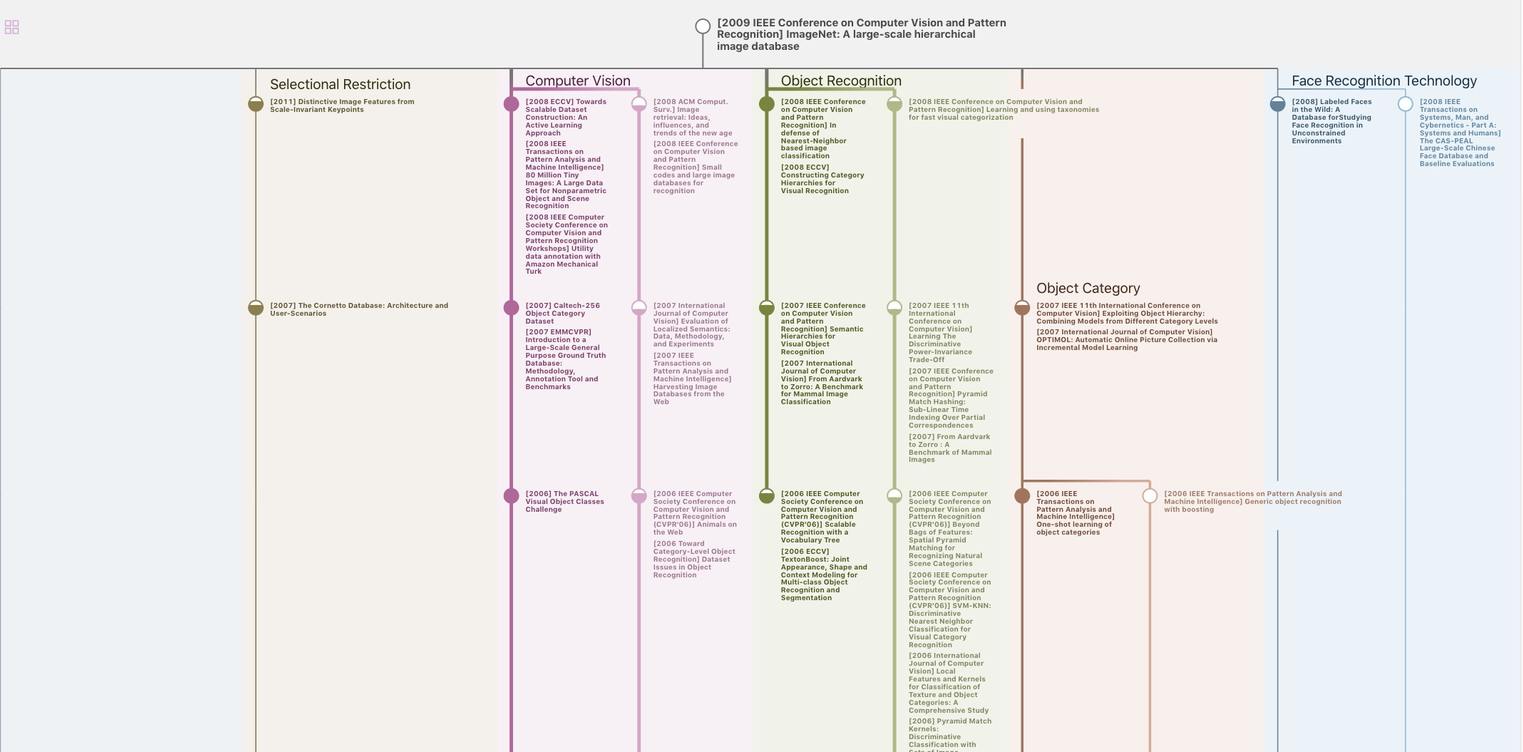
生成溯源树,研究论文发展脉络
Chat Paper
正在生成论文摘要