Selecting a clustering algorithm: A semi-automated hyperparameter tuning framework for effective persona development
Array(2022)
摘要
When approaching a clustering problem, such as during persona development, selecting the most appropriate algorithm and parameter combination is essential. The hyperparameter tuning process required to determine the best combination is often tedious and thus automated through evaluation metrics. However, there are no ground truth values available for the empirical evaluation of clustering algorithms and existing internal metrics cannot comment on the quality of a set of clusters for their proposed use case. This paper presents a semi-automated framework for the hyperparameter tuning of clustering algorithms for persona development, HyPersona, which minimises the manual intervention required through simple evaluation and the production of informative graphs and early-stage personas. Within HyPersona, an internal metric focused on aspects necessary to developing quality persons, average feature significance (AFS), is proposed to assist in the evaluation of results. HyPersona was validated through application to a real-world persona development problem, evaluating the three most widely used clustering algorithms for persona development. HyPersona was compared to existing hyperparameter tuning and persona development methods and developed personas of a comparable quality whilst reducing manual intervention. The proposed internal metric, AFS, was found to provide a unique insight into the performance of cluster sets for persona development.
更多查看译文
关键词
Automated persona development,Machine learning,Clustering algorithms,Hyperparameter tuning,Internal evaluation metrics
AI 理解论文
溯源树
样例
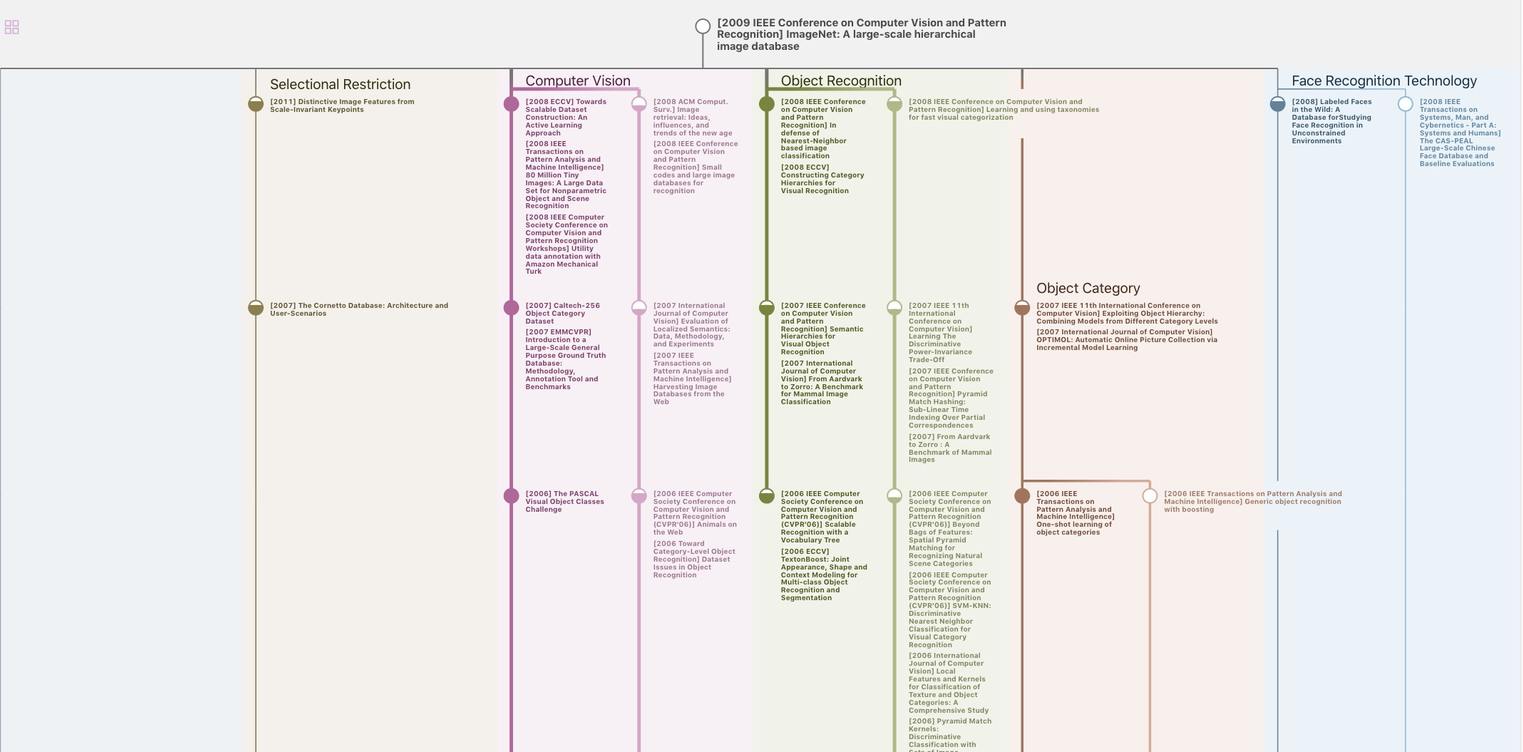
生成溯源树,研究论文发展脉络
Chat Paper
正在生成论文摘要