Few-Shot Adaptation of Pre-Trained Networks for Domain Shift
International Joint Conference on Artificial Intelligence(2022)
摘要
Deep networks are prone to performance degradation when there is a domain shift between the source (training) data and target (test) data. Recent test-time adaptation methods update batch normalization layers of pre-trained source models deployed in new target environments with streaming data to mitigate such performance degradation. Although such methods can adapt on-the-fly without first collecting a large target domain dataset, their performance is dependent on streaming conditions such as mini-batch size and class-distribution, which can be unpredictable in practice. In this work, we propose a framework for few-shot domain adaptation to address the practical challenges of data-efficient adaptation. Specifically, we propose a constrained optimization of feature normalization statistics in pre-trained source models supervised by a small support set from the target domain. Our method is easy to implement and improves source model performance with as few as one sample per class for classification tasks. Extensive experiments on 5 cross-domain classification and 4 semantic segmentation datasets show that our method achieves more accurate and reliable performance than test-time adaptation, while not being constrained by streaming conditions.
更多查看译文
关键词
Computer Vision: Transfer, low-shot, semi- and un- supervised learning,Machine Learning: Robustness
AI 理解论文
溯源树
样例
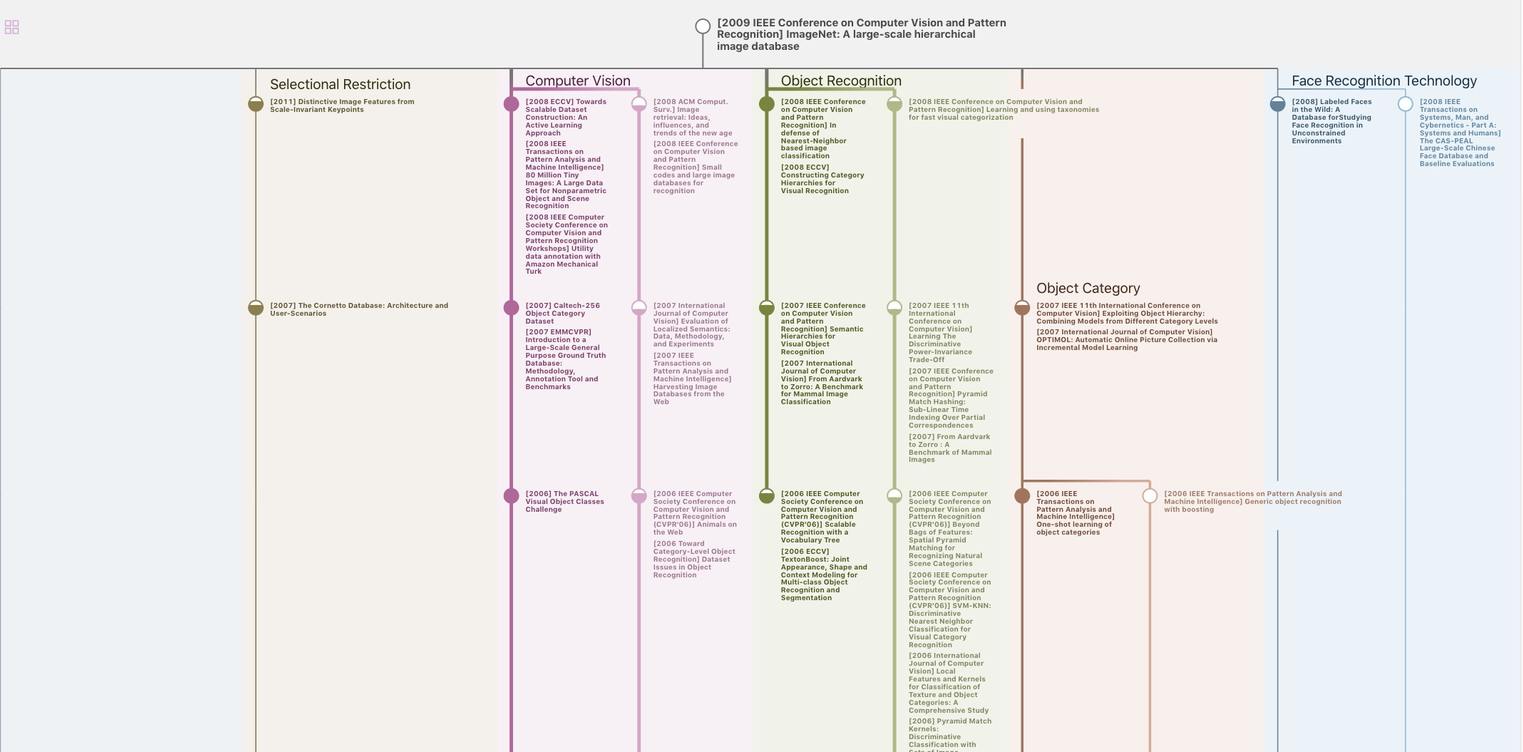
生成溯源树,研究论文发展脉络
Chat Paper
正在生成论文摘要