Towards Efficient 3D Object Detection with Knowledge Distillation
ADVANCES IN NEURAL INFORMATION PROCESSING SYSTEMS 35, NEURIPS 2022(2022)
Abstract
Despite substantial progress in 3D object detection, advanced 3D detectors often suffer from heavy computation overheads. To this end, we explore the potential of knowledge distillation (KD) for developing efficient 3D object detectors, focusing on popular pillar- and voxel-based detectors. In the absence of well-developed teacher-student pairs, we first study how to obtain student models with good trade offs between accuracy and efficiency from the perspectives of model compression and input resolution reduction. Then, we build a benchmark to assess existing KD methods developed in the 2D domain for 3D object detection upon six well-constructed teacher-student pairs. Further, we propose an improved KD pipeline incorporating an enhanced logit KD method that performs KD on only a few pivotal positions determined by teacher classification response, and a teacher-guided student model initialization to facilitate transferring teacher model's feature extraction ability to students through weight inheritance. Finally, we conduct extensive experiments on the Waymo and KITTI dataset. Our best performing model achieves 65.75% LEVEL 2 mAPH, surpassing its teacher model and requiring only 44% of teacher flops on Waymo. Our most efficient model runs 51 FPS on an NVIDIA A100, which is 2.2x faster than PointPillar with even higher accuracy on Waymo. Code is available at https://github.com/CVMI-Lab/SparseKD.
MoreTranslated text
AI Read Science
Must-Reading Tree
Example
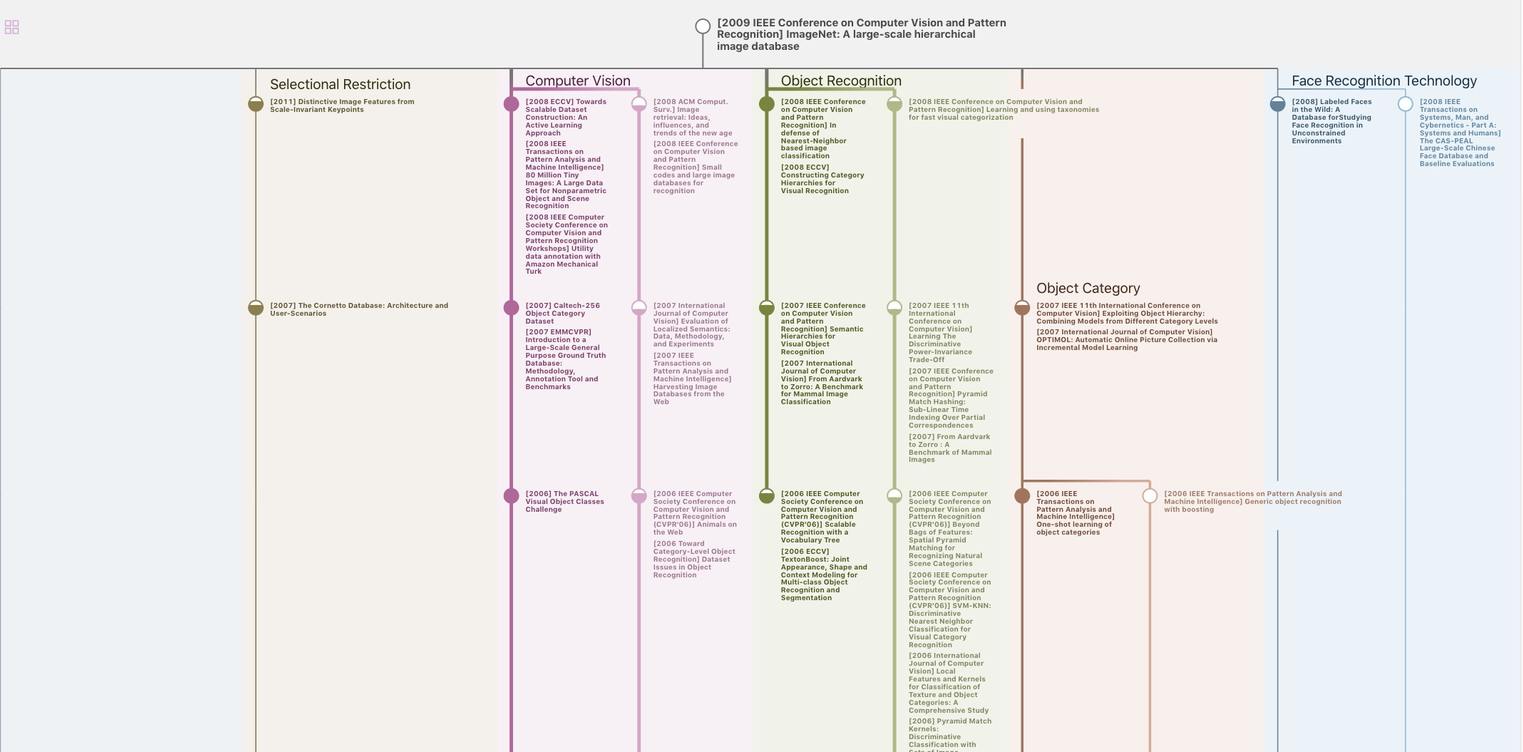
Generate MRT to find the research sequence of this paper
Chat Paper
Summary is being generated by the instructions you defined