The impact of memory on learning sequence-to-sequence tasks
MACHINE LEARNING-SCIENCE AND TECHNOLOGY(2024)
摘要
The recent success of neural networks in natural language processing has drawn renewed attention to learning sequence-to-sequence (seq2seq) tasks. While there exists a rich literature that studies classification and regression tasks using solvable models of neural networks, seq2seq tasks have not yet been studied from this perspective. Here, we propose a simple model for a seq2seq task that has the advantage of providing explicit control over the degree of memory, or non-Markovianity, in the sequences-the stochastic switching-Ornstein-Uhlenbeck (SSOU) model. We introduce a measure of non-Markovianity to quantify the amount of memory in the sequences. For a minimal auto-regressive (AR) learning model trained on this task, we identify two learning regimes corresponding to distinct phases in the stationary state of the SSOU process. These phases emerge from the interplay between two different time scales that govern the sequence statistics. Moreover, we observe that while increasing the integration window of the AR model always improves performance, albeit with diminishing returns, increasing the non-Markovianity of the input sequences can improve or degrade its performance. Finally, we perform experiments with recurrent and convolutional neural networks that show that our observations carry over to more complicated neural network architectures.
更多查看译文
关键词
sequence-to-sequence task,auto-regressive model,recurrent neural network,memory,non-Markovianity,statistical physics
AI 理解论文
溯源树
样例
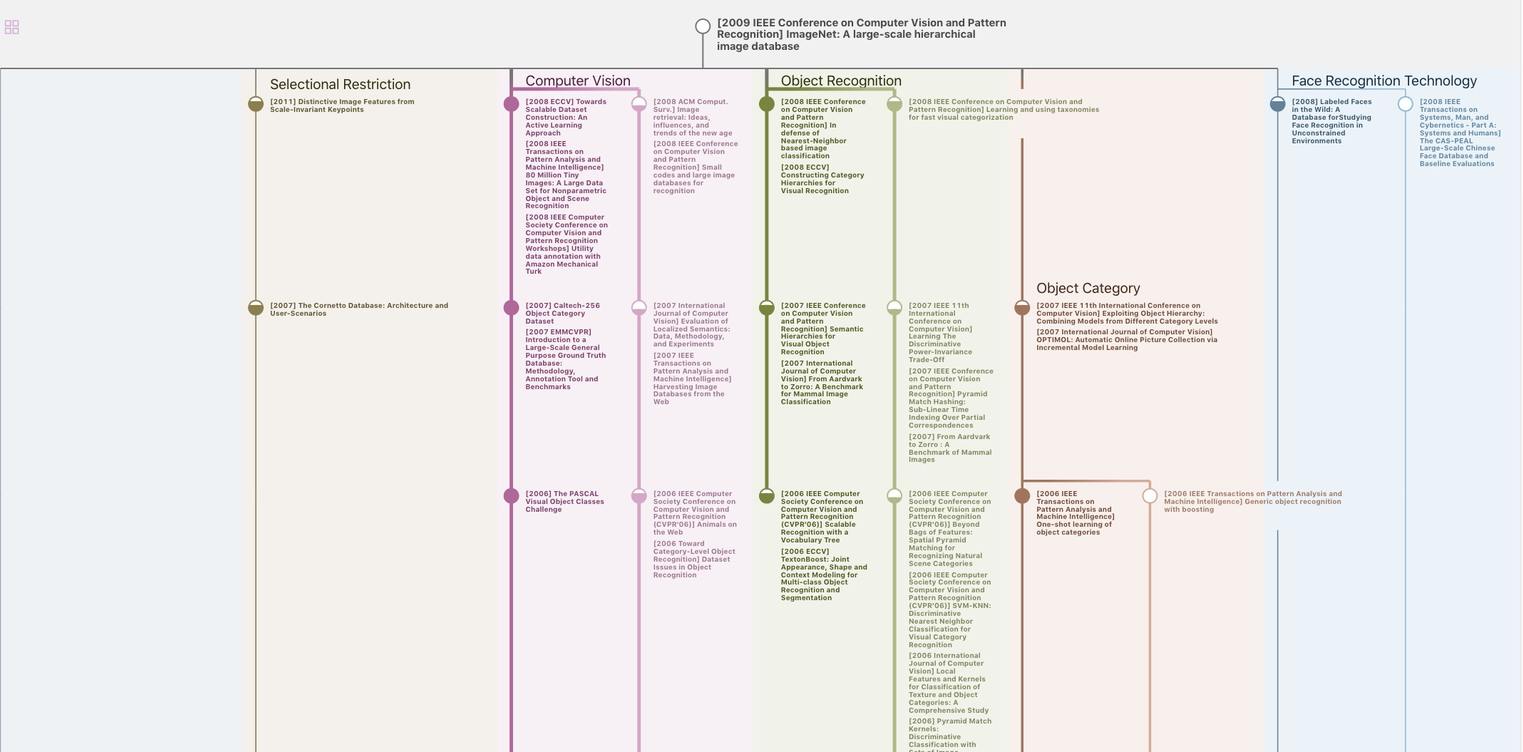
生成溯源树,研究论文发展脉络
Chat Paper
正在生成论文摘要