Diffusion-LM Improves Controllable Text Generation
NeurIPS 2022(2022)
摘要
Controlling the behavior of language models (LMs) without re-training is a major open problem in natural language generation. While recent works have demonstrated successes on controlling simple sentence attributes (e.g., sentiment), there has been little progress on complex, fine-grained controls (e.g., syntactic structure). To address this challenge, we develop a new non-autoregressive language model based on continuous diffusions that we call Diffusion-LM. Building upon the recent successes of diffusion models in continuous domains, Diffusion-LM iteratively denoises a sequence of Gaussian vectors into word vectors, yielding a sequence of intermediate latent variables. The continuous, hierarchical nature of these intermediate variables enables a simple gradient-based algorithm to perform complex, controllable generation tasks. We demonstrate successful control of Diffusion-LM for six challenging fine-grained control tasks, significantly outperforming prior work.
更多查看译文
关键词
controllable text generation,controlled generation,infilling,language model,diffusion model
AI 理解论文
溯源树
样例
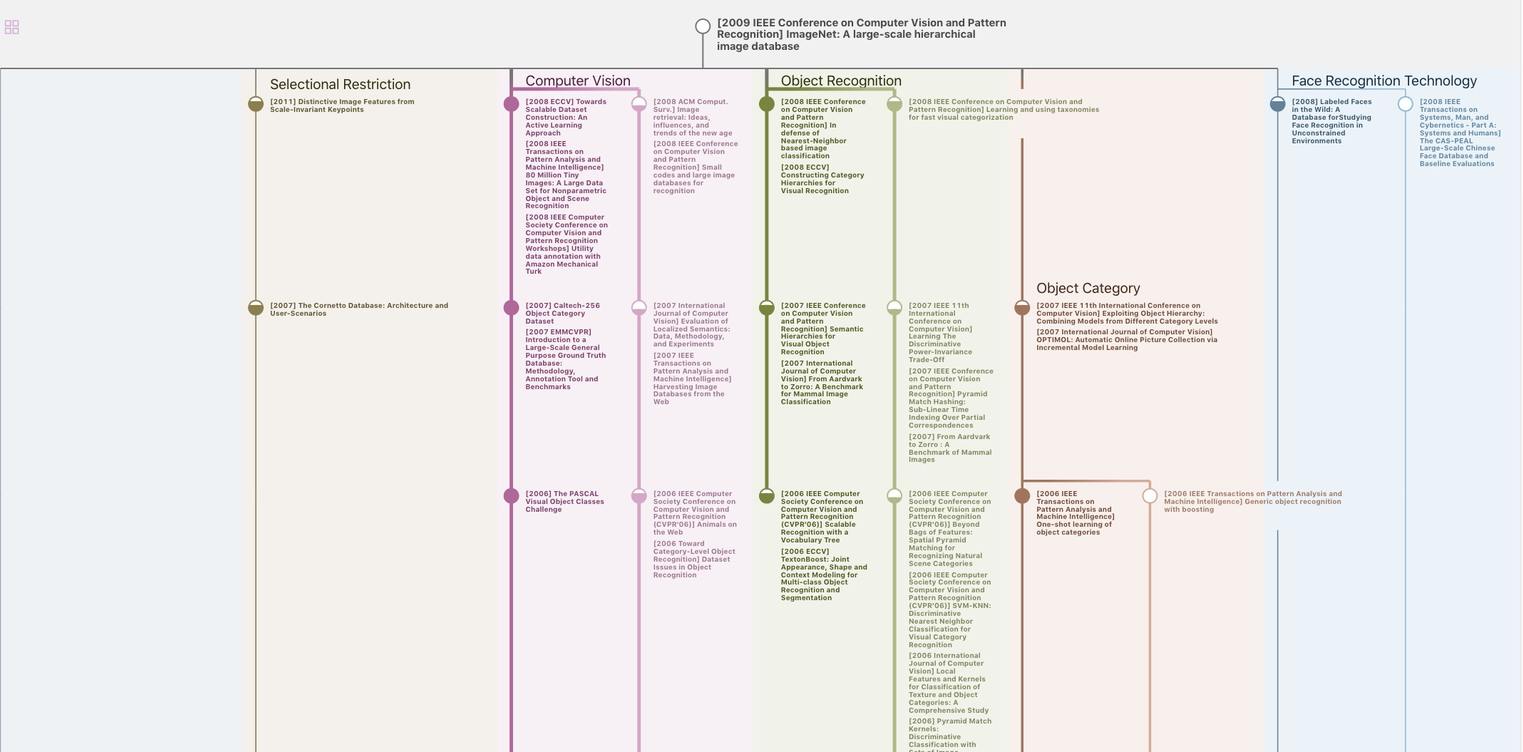
生成溯源树,研究论文发展脉络
Chat Paper
正在生成论文摘要