KL-Entropy-Regularized RL with a Generative Model is Minimax Optimal
arxiv(2022)
摘要
In this work, we consider and analyze the sample complexity of model-free reinforcement learning with a generative model. Particularly, we analyze mirror descent value iteration (MDVI) by Geist et al. (2019) and Vieillard et al. (2020a), which uses the Kullback-Leibler divergence and entropy regularization in its value and policy updates. Our analysis shows that it is nearly minimax-optimal for finding an $\varepsilon$-optimal policy when $\varepsilon$ is sufficiently small. This is the first theoretical result that demonstrates that a simple model-free algorithm without variance-reduction can be nearly minimax-optimal under the considered setting.
更多查看译文
关键词
generative model,kl-entropy-regularized
AI 理解论文
溯源树
样例
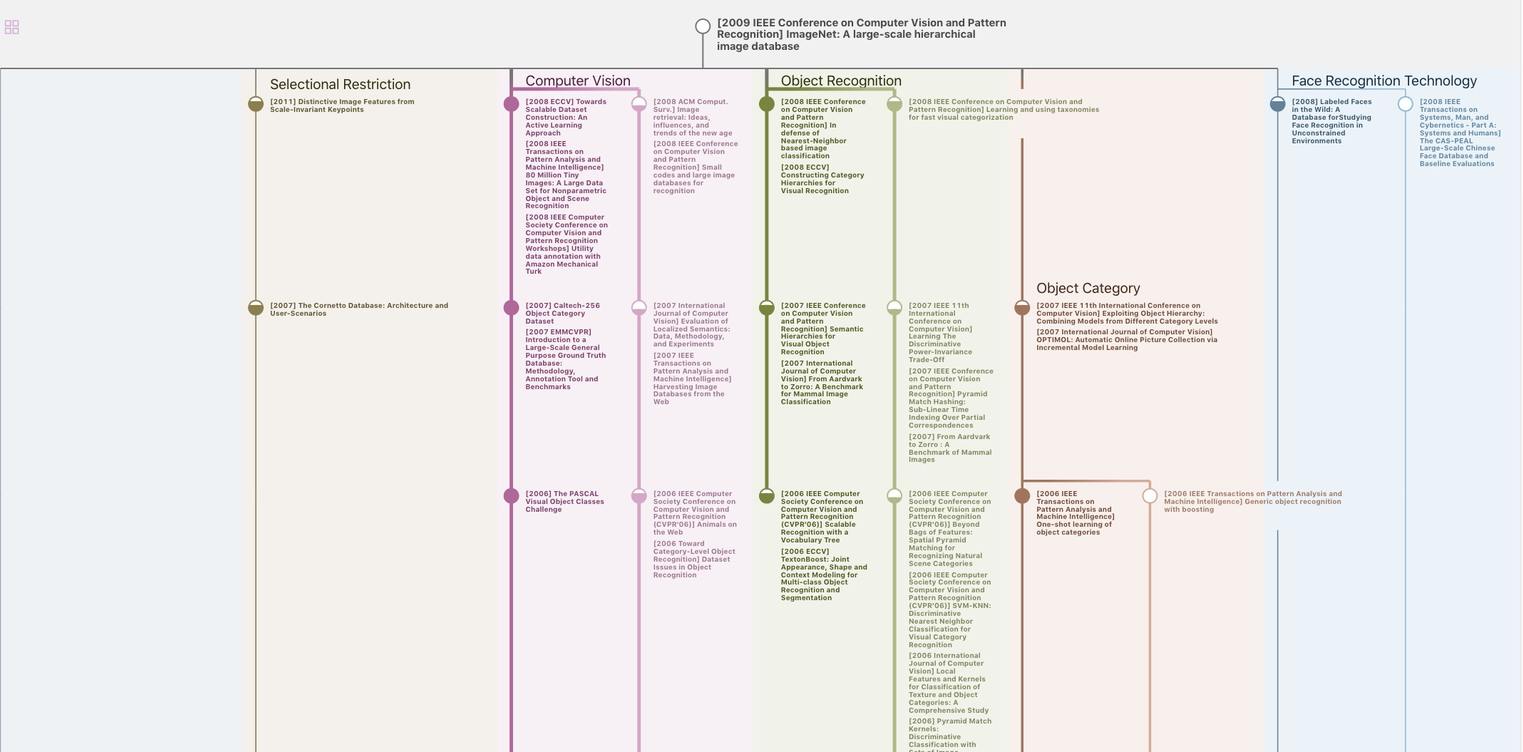
生成溯源树,研究论文发展脉络
Chat Paper
正在生成论文摘要