Prototype Based Classification from Hierarchy to Fairness.
International Conference on Machine Learning(2022)
摘要
Artificial neural nets can represent and classify many types of high-dimensional data but are often tailored to particular applications – e.g., for “fair” or “hierarchical” classification. Once an architecture has been selected, it is often difficult for humans to adjust models for a new task; for example, a hierarchical classifier cannot be easily transformed into a fair classifier that shields a protected field. Our contribution in this work is a new neural network architecture, the concept subspace network (CSN), which generalizes existing specialized classifiers to produce a unified model capable of learning a spectrum of multi-concept relationships. We demonstrate that CSNs reproduce state-of-the-art results in fair classification when enforcing concept independence, may be transformed into hierarchical classifiers, or may even reconcile fairness and hierarchy within a single classifier. The CSN is inspired by and matches the performance of existing prototype-based classifiers that promote interpretability.
更多查看译文
关键词
classification,fairness,hierarchy,prototype
AI 理解论文
溯源树
样例
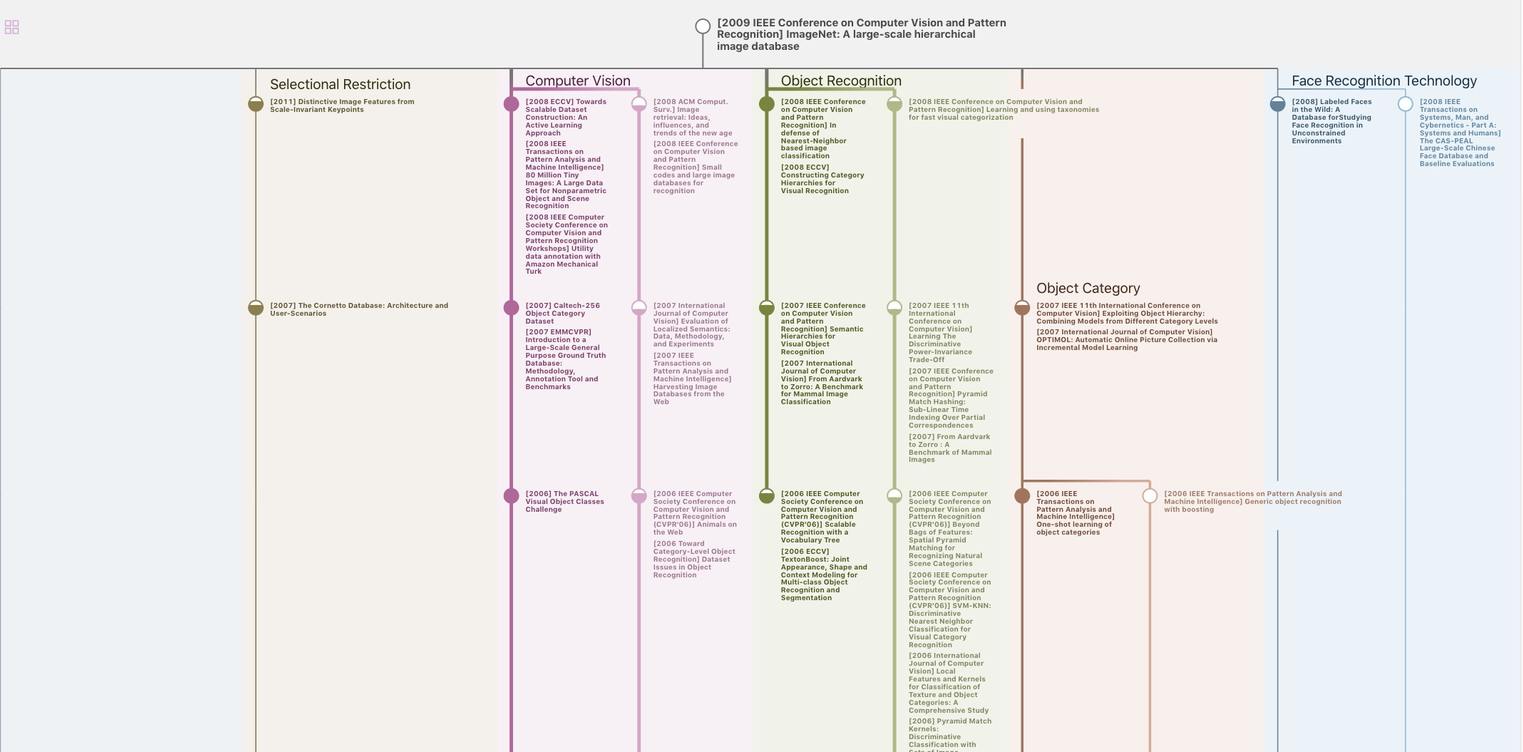
生成溯源树,研究论文发展脉络
Chat Paper
正在生成论文摘要