Deep Ensembles for Graphs with Higher-order Dependencies
ICLR 2023(2022)
摘要
Graph neural networks (GNNs) continue to achieve state-of-the-art performance on many graph learning tasks, but rely on the assumption that a given graph is a sufficient approximation of the true neighborhood structure. When a system contains higher-order sequential dependencies, we show that the tendency of traditional graph representations to underfit each node's neighborhood causes existing GNNs to generalize poorly. To address this, we propose a novel Deep Graph Ensemble (DGE), which captures neighborhood variance by training an ensemble of GNNs on different neighborhood subspaces of the same node within a higher-order network structure. We show that DGE consistently outperforms existing GNNs on semisupervised and supervised tasks on six real-world data sets with known higher-order dependencies, even under a similar parameter budget. We demonstrate that learning diverse and accurate base classifiers is central to DGE's success, and discuss the implications of these findings for future work on ensembles of GNNs.
更多查看译文
关键词
graph neural networks,higher order networks,deep ensembles,representation learning,semisupervised learning
AI 理解论文
溯源树
样例
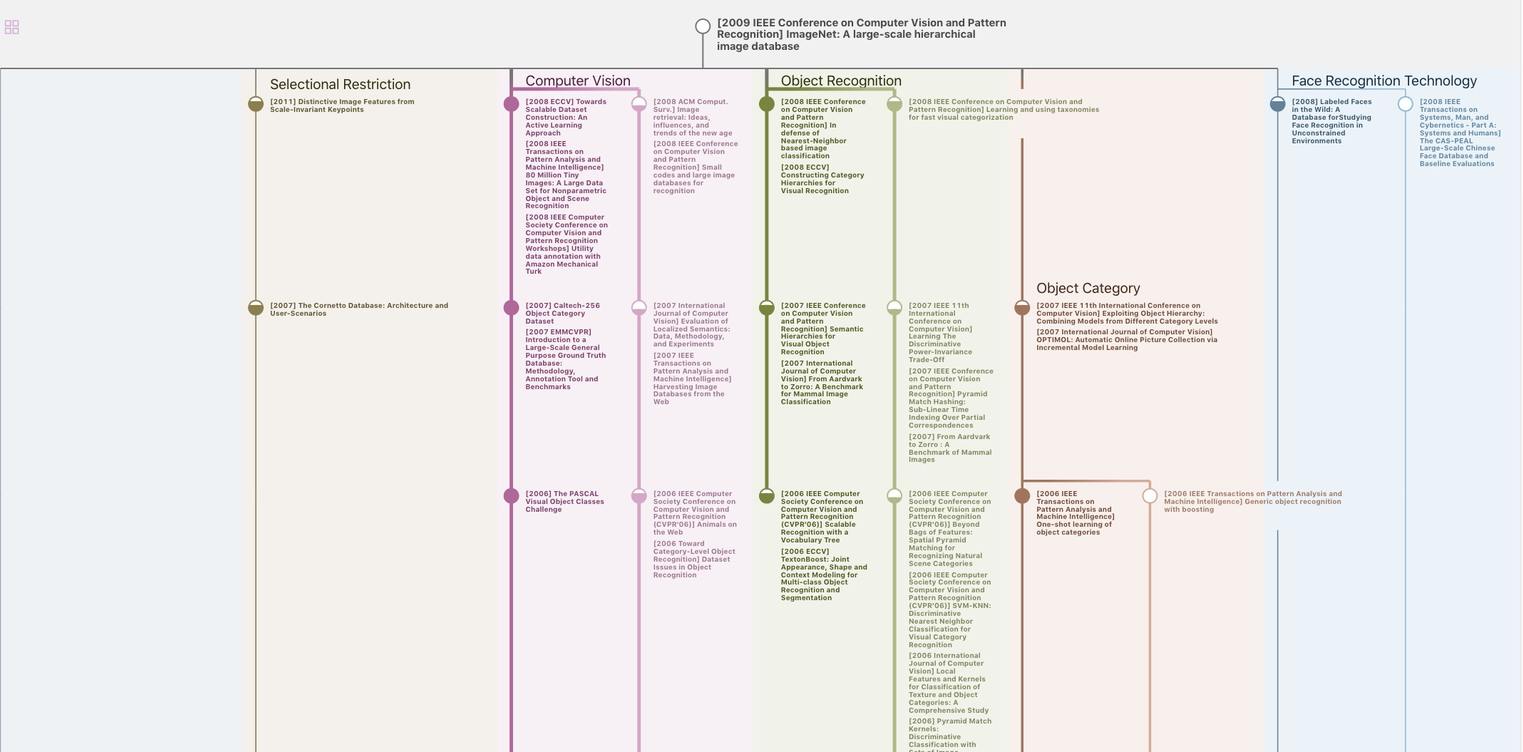
生成溯源树,研究论文发展脉络
Chat Paper
正在生成论文摘要