3D Human Pose and Shape Reconstruction From Videos via Confidence-Aware Temporal Feature Aggregation
user-61447a76e55422cecdaf7d19(2023)
摘要
Estimating 3D human body shapes and poses from videos is a challenging computer vision task. The intrinsic temporal information embedded in adjacent frames is helpful in making accurate estimations. Existing approaches learn temporal features of the target frames simply by aggregating features of their adjacent frames, using off-the-shelf deep neural networks. Consequently these approaches cannot explicitly and effectively use the correlations between adjacent frames to help infer the parameters of the target frames. In this paper, we propose a novel framework that can measure the correlations amongst adjacent frames in the form of an estimated confidence metric. The confidence value will indicate to what extent the adjacent frames can help predict the target frames' 3D shapes and poses. Based on the estimated confidence values, temporally aggregated features are then obtained by adaptively allocating different weights to the temporal predicted features from the adjacent frames. The final 3D shapes and poses are estimated by regressing from the temporally aggregated features. Experimental results on three benchmark datasets show that the proposed method outperforms state-ofthe-art approaches (even without the motion priors involved in training). In particular, the proposed method is more robust against corrupted frames.
更多查看译文
关键词
Three-dimensional displays,Feature extraction,Shape,Training,Correlation,Solid modeling,Videos,Human pose,temporal estimation,uncertainty
AI 理解论文
溯源树
样例
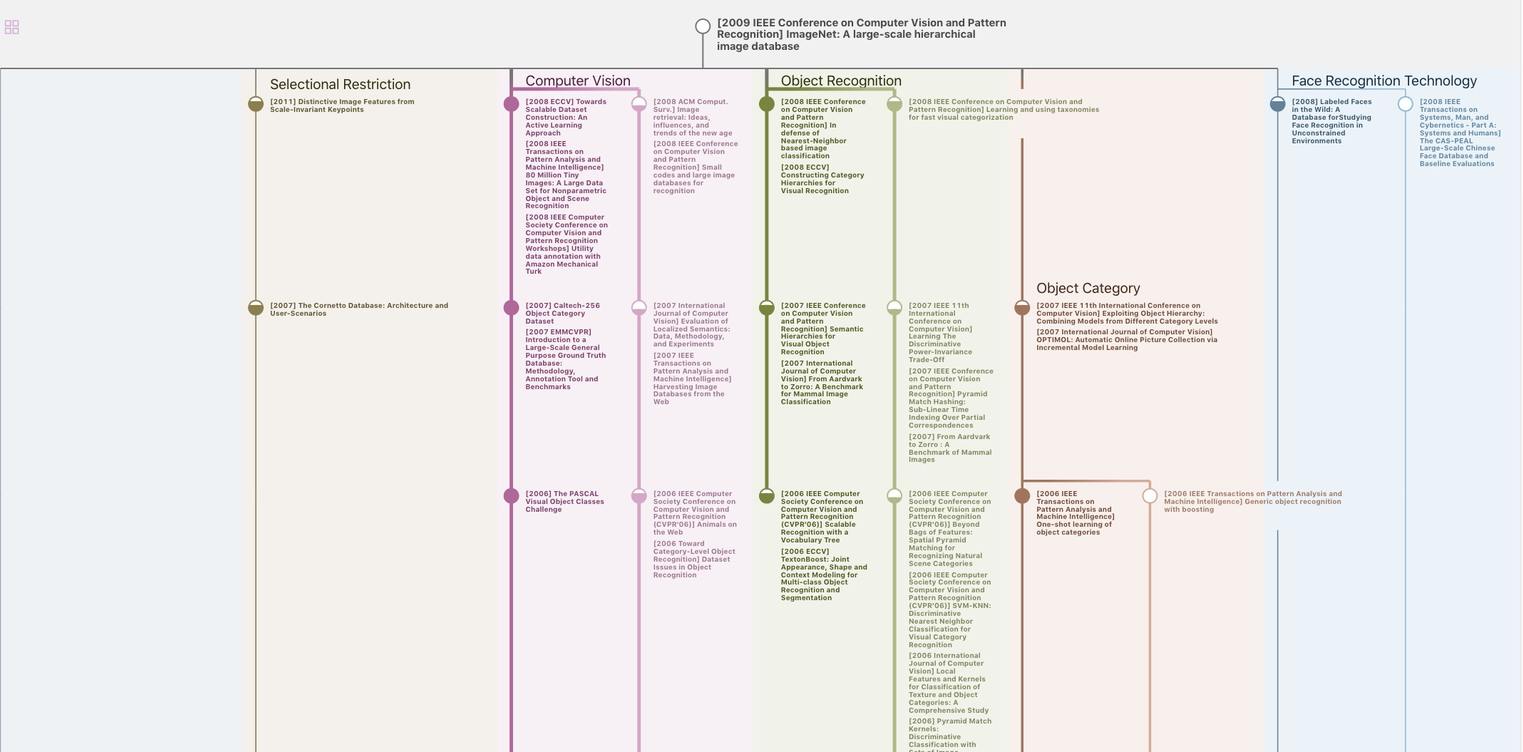
生成溯源树,研究论文发展脉络
Chat Paper
正在生成论文摘要