Incomplete Multi-View Clustering With Sample-Level Auto-Weighted Graph Fusion.
user-61447a76e55422cecdaf7d19(2023)
摘要
Incomplete multi-view clustering (IMC) has received considerable attention due to its flexibility in fusing the multi-view information when the view samples are partly missing. However, existing methods seldom consider the affection of the missing samples to the contributions of the views. In this paper, we propose a novel graph fusion based IMC model (SAGF_IMC) to handle this problem. Instead of directly weighting the whole view, SAGF_IMC learns the sample-level auto weight, which allows considering both the contributions of different views and the affection of the missing samples. An effective iterative algorithm is developed, together with its convergence analysis. Experiments are provided to demonstrate that SAGF_IMC is superior to the related state-of-the-art methods by using several real-world datasets.
更多查看译文
关键词
Incomplete multi-view clustering,graph fusion,sample weight,auto-weight learning
AI 理解论文
溯源树
样例
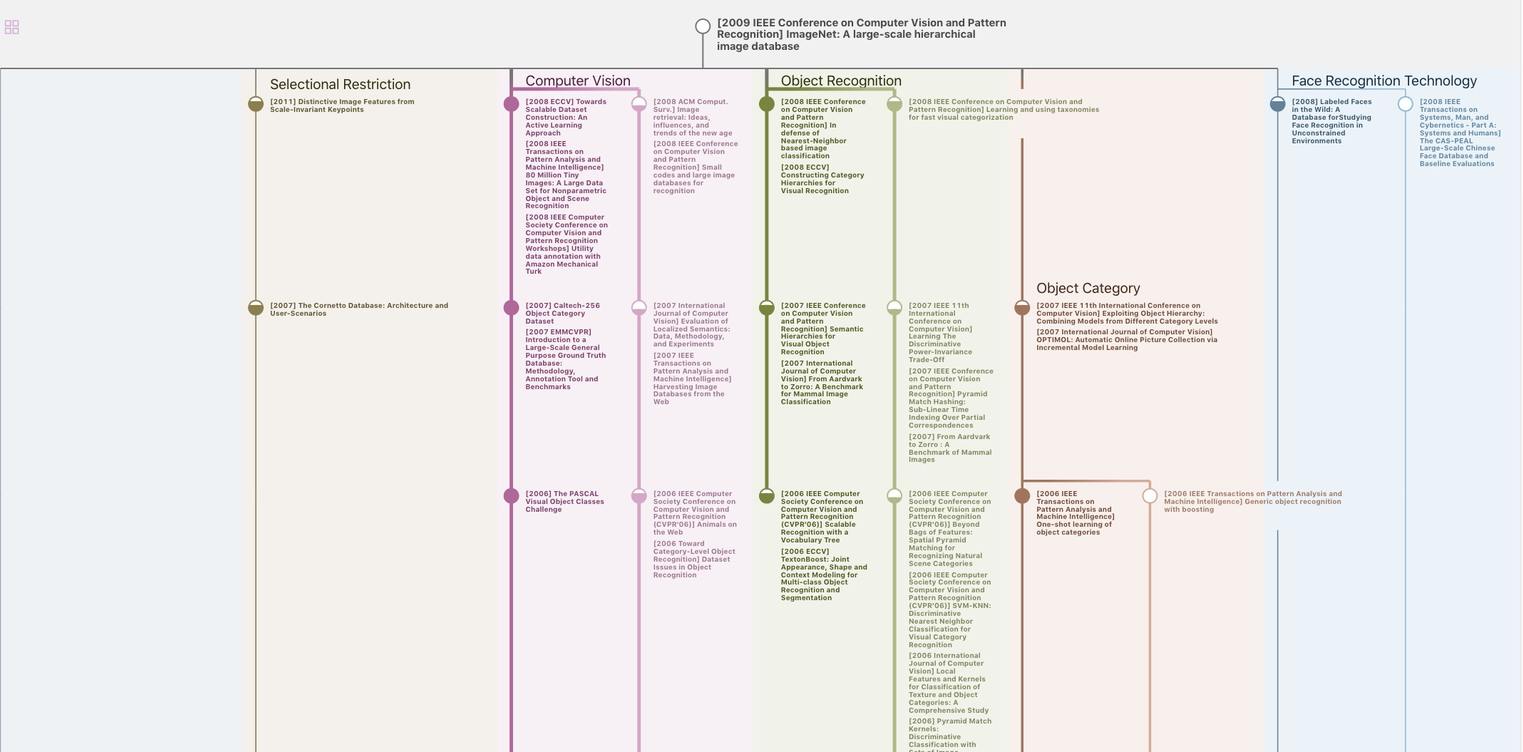
生成溯源树,研究论文发展脉络
Chat Paper
正在生成论文摘要