Risk Prediction of Coal and Gas Outburst Based on Abnormal Gas Concentration in Blasting Driving Face
GEOFLUIDS(2022)
摘要
In order to realize dynamic, continuous, and real-time prediction of coal and gas outburst risk in real time in blasting driving face, an outburst risk prediction method based on the characteristics of gas emission after blasting is proposed. In this study, the causes of abnormal gas concentration in blasting driving face are analyzed, and the identification method of abnormal gas concentration based on weighted K-nearest neighbor (weighted KNN) is proposed. The correlation between gas emission characteristics after blasting and K-1 value is analyzed, and the prediction model of outburst risk based on convolutional neural networks (CNN) is established and applied in Jinjia coal mine in China. The results show that the causes of abnormal gas concentration mainly include ventilation stop, blasting operation, sensor adjustment, and other abnormalities. The accuracy of the identification method is 86.1%. Especially, the identification accuracy of blasting operation is 92%. There are strong correlations between the growth rate, peak value, and decay rate of gas concentration after blasting and K-1 value, and the maximum correlation coefficient is 0.92. Using the prediction model, 28 times of jet holes and 1 small outburst event are predicted successfully, and the efficiency of the prediction model is 76.39%. By this technology, the utilization rate of gas information is improved, and the relationship between the change characteristics of gas concentration after blasting and the risk of coal seam outburst is established, which is of significant for improving the prediction accuracy and risk management ability of coal and gas outburst.
更多查看译文
AI 理解论文
溯源树
样例
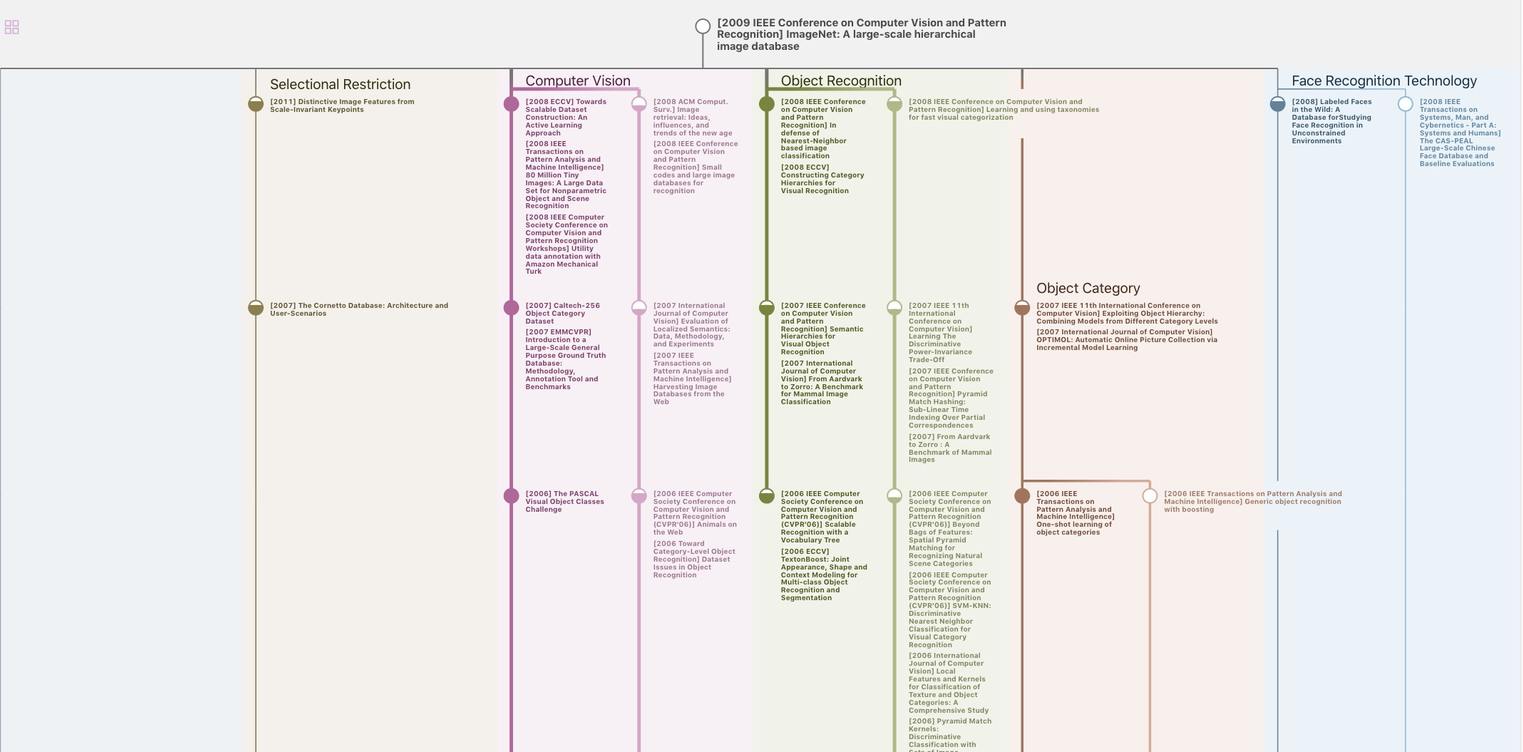
生成溯源树,研究论文发展脉络
Chat Paper
正在生成论文摘要