Texture-suppression-based surface defect detection of milled aluminum ingot
user-61447a76e55422cecdaf7d19(2022)
摘要
The surface quality of aluminum ingot has a great influence on the subsequent rolling process, so defect detection is a critical step after milling. However, it is a challenging task, owing to multi-direction and multi-scale of milling texture pattern, and sometimes uneven distribution of texture primitives. In this paper, a novel texture-suppression-based defect detection method combined with wavelet decomposition and relative total variation (RTV) for milled surface is proposed. In order to adaptively decide the scale factor of RTV which is vital in texture-structure separation, we first resort to mine intrinsic priors of defect and milling grains in the detail sub images derived from the wavelet decomposition. Secondly, based on the mined prior rules, a detail sub image is automatically selected from different decomposition levels and the corresponding scale factor for RTV is also determined. Then, by feeding the selected sub image into the RTV model, the texture is suppressed and the main structures that containing defects are extracted. Compared with taking the original image as the input, the wavelet preprocessed sub image effectively weakens the influence of the scale and direction change of texture pattern, and greatly improves the time efficiency of RTV. Finally, through the simple gradient calculation and binarization of the structure image, the defects are segmented. The experimental results show that the proposed method is robust and effective to detect various surface defects of steel ingot with complex milling texture.
更多查看译文
AI 理解论文
溯源树
样例
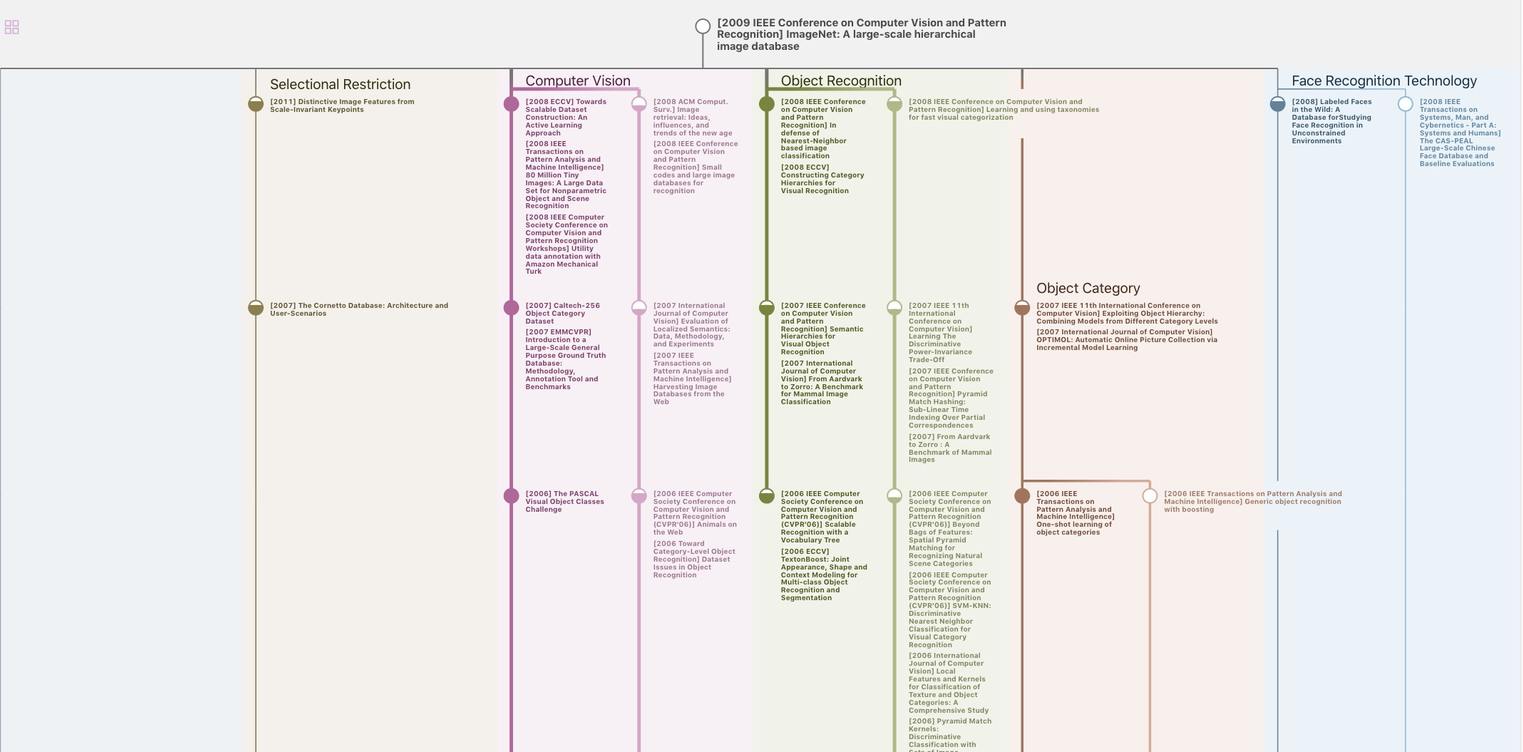
生成溯源树,研究论文发展脉络
Chat Paper
正在生成论文摘要