Incorporating Linear Regression Problems Into an Adaptive Framework With Feasible Optimizations
IEEE TRANSACTIONS ON MULTIMEDIA(2023)
摘要
Accompanied with the increasing popularity of linear regression approaches, most of the existing minimization problems are related with several convex measurements, e.g., l(1)/l(2) /l(2,1) norm of a vector and L-1/L-2,L-1/Frobenius/nuclear norm of amatrix, where the regularized function and the loss function are usually studied for two objective terms case by case, respectively. To address this issue, this work combines these linear regression problems into a unified expression framework by employing an adaptive and flexible function, in which we need to choose different variable elements and adjust an inner parameter, properly. Besides this, they are equipped with some corresponding relationships and their interesting properties. Intuitively speaking, the proposed framework can generalize several traditional linear regression formulations and even more complex ones into an extended representation. For further optimizations, an iteratively re-weighted penalty solution (IRwPS) is devised without any inner loops, making the iteration programming easy to perform. Meanwhile, the theoretical results are provided for guaranteeing that themathematical convergence analysis is solid andmeaningful. Finally, by performing real-world applications in supervised, unsupervised, and semi-supervised tasks, numerical experiments are conducted to validate the theoretical properties and the superiority over some of the state-of-the-art.
更多查看译文
关键词
Linear regression,Optimization,Loss measurement,Adaptation models,Matrices,Sparse matrices,Convergence,Linear regression,convex vector and matrix norm,iteratively re-weighted algorithm,convergence analysis
AI 理解论文
溯源树
样例
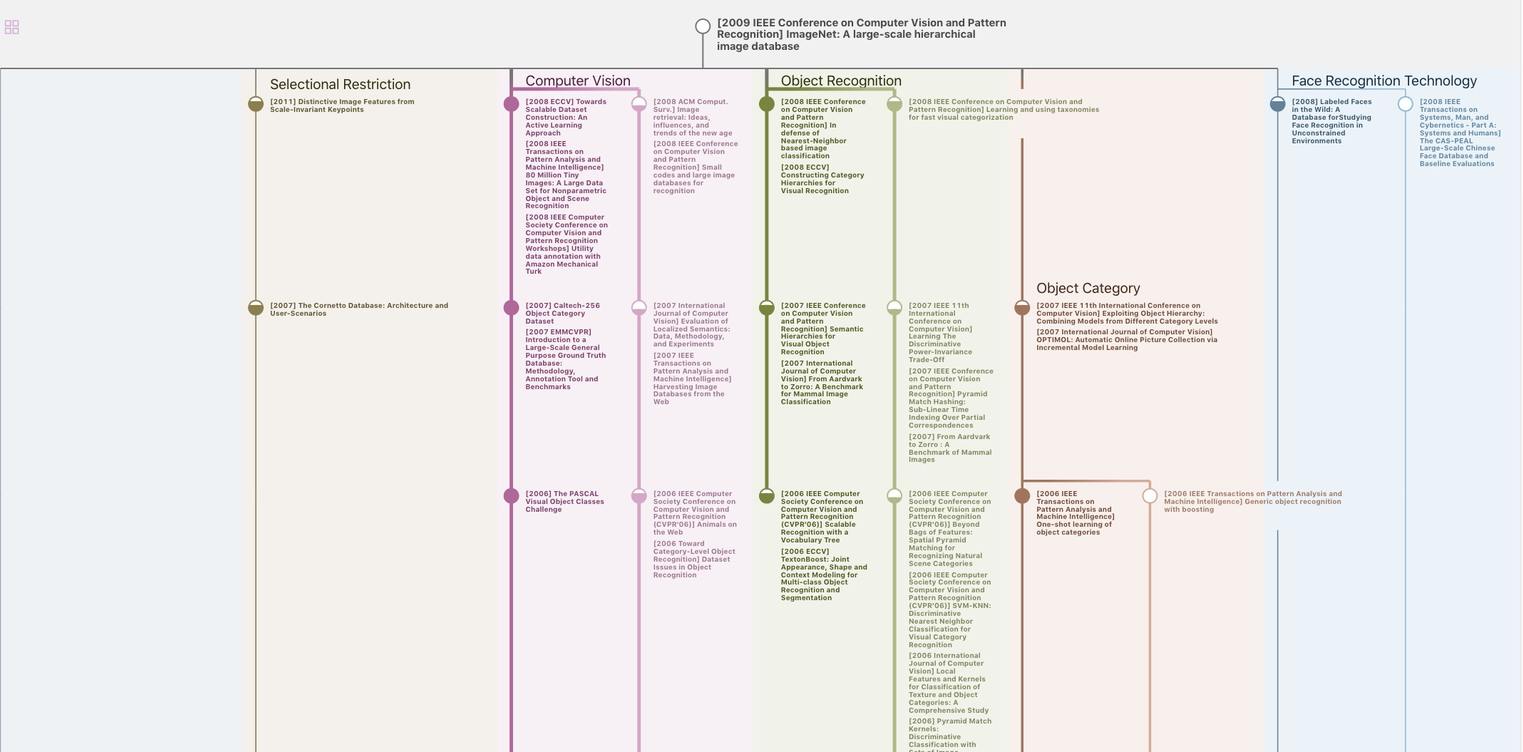
生成溯源树,研究论文发展脉络
Chat Paper
正在生成论文摘要