COMB-MCM: Computing-on-Memory-Boundary NN Processor with Bipolar Bitwise Sparsity Optimization for Scalable Multi-Chiplet-Module Edge Machine Learning.
International Solid-State Circuits Conference(2022)
摘要
Recently, computing-in-memory (CIM) macros, originally designed to reduce the intensive memory accesses of Al tasks, have been employed in low-power machine learning SoCs due to their ultra-high computing efficiency [1]–[3]. These CIM macros still access weight data through on/off-chip memories, similar to processing elements in near-memory-computing architectures. The implementation poses challenges when counting the overall SoC energy efficiency (Fig. 15.3.1). First, the memory wall issue is unsolved. The weight updates affect overall system performance when large networks are deployed and massive off-chip weight data transfer occurs. Even for tiny machine learning tasks, power consumption and latency of constant weight updates cannot be neglected, because MAC computing efficiency is optimized and closely matches the efficiency of on-chip memory access (2pJ/b vs. 1pJ/b). Second, the viability of structured and coarse-grained sparsity optimization is highly algorithm dependent and requires explicit zero-detection blocks. Power optimization schemes for fine-grained or even arbitrary-sparsity patterns are lacking. Third, edge machine learning chips are cost sensitive. The conventional monolithic SoC design strategy, fabricating one specific SoC for each application, is not affordable in terms of NRE costs.
更多查看译文
关键词
COMB-MCM,computing-on-memory-boundary NN processor,bipolar bitwise sparsity optimization,scalable multichiplet-module edge machine learning,computing-in-memory macros,CIM,intensive memory accesses,low-power machine learning SoCs,ultra-high computing efficiency,access weight data,processing elements,near-memory-computing architectures,SoC energy efficiency,memory wall issue,off-chip weight data transfer,tiny machine learning tasks,power consumption,constant weight updates,MAC computing efficiency,on-chip memory access,coarse-grained sparsity optimization,power optimization schemes,arbitrary-sparsity patterns,edge machine learning chips,conventional monolithic SoC design strategy
AI 理解论文
溯源树
样例
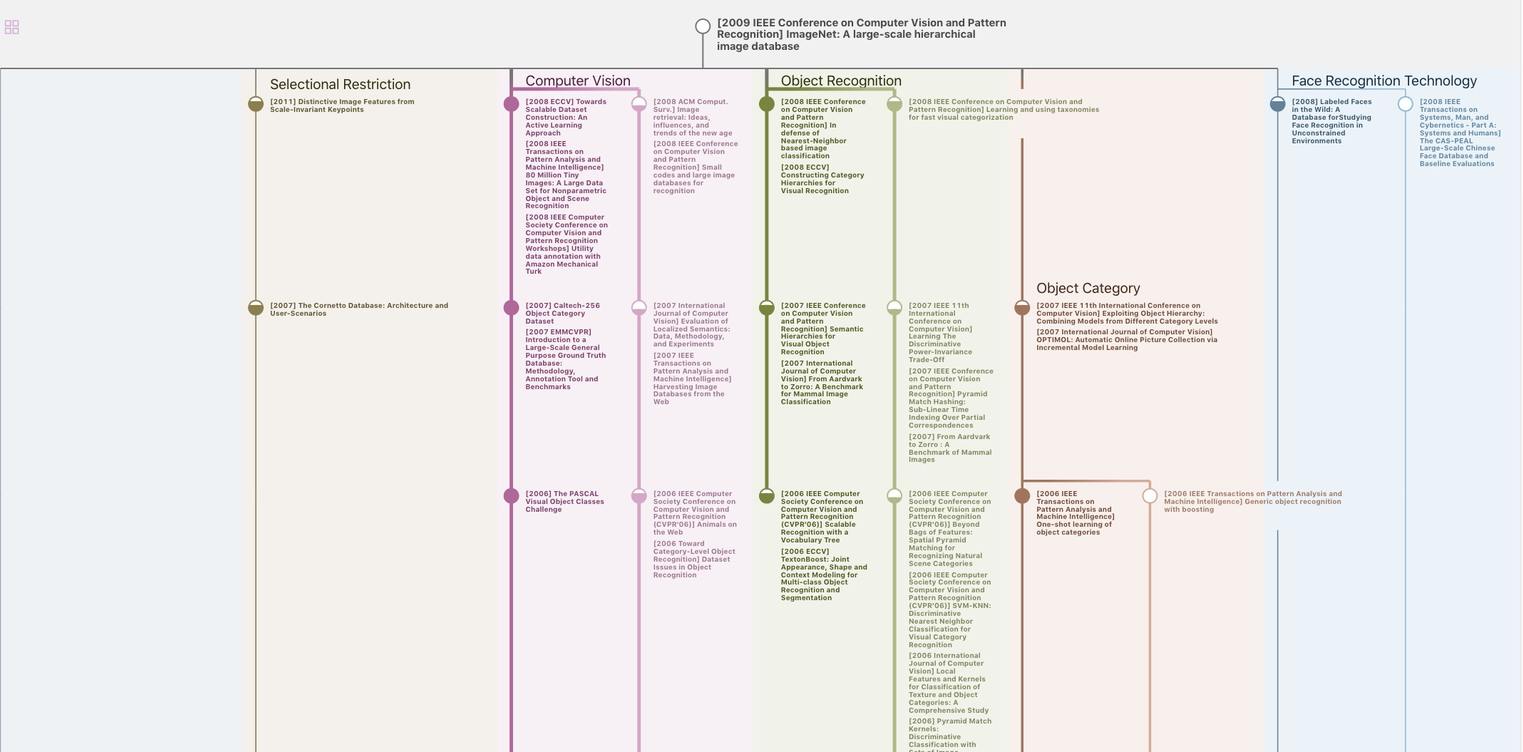
生成溯源树,研究论文发展脉络
Chat Paper
正在生成论文摘要