AutoTSG: learning and synthesis for incident troubleshooting.
ACM SIGSOFT Conference on the Foundations of Software Engineering (FSE)(2022)
摘要
Incident management is a key aspect of operating large-scale cloud services. To aid with faster and efficient resolution of incidents, engineering teams document frequent troubleshooting steps in the form of Troubleshooting Guides (TSGs), to be used by on-call engineers (OCEs). However, TSGs are siloed, unstructured, and often incomplete, requiring developers to manually understand and execute necessary steps. This results in a plethora of issues such as on-call fatigue, reduced productivity, and human errors. In this work, we conduct a large-scale empirical study of over 4K+ TSGs mapped to 1000s of incidents and find that TSGs are widely used and help significantly reduce mitigation efforts. We then analyze feedback on TSGs provided by 400+ OCEs and propose a taxonomy of issues that highlights significant gaps in TSG quality. To alleviate these gaps, we investigate the automation of TSGs and propose AutoTSG -- a novel framework for automation of TSGs to executable workflows by combining machine learning and program synthesis. Our evaluation of AutoTSG on 50 TSGs shows the effectiveness in both identifying TSG statements (accuracy 0.89) and parsing them for execution (precision 0.94 and recall 0.91). Lastly, we survey ten Microsoft engineers and show the importance of TSG automation and the usefulness of AutoTSG.
更多查看译文
关键词
troubleshooting,learning
AI 理解论文
溯源树
样例
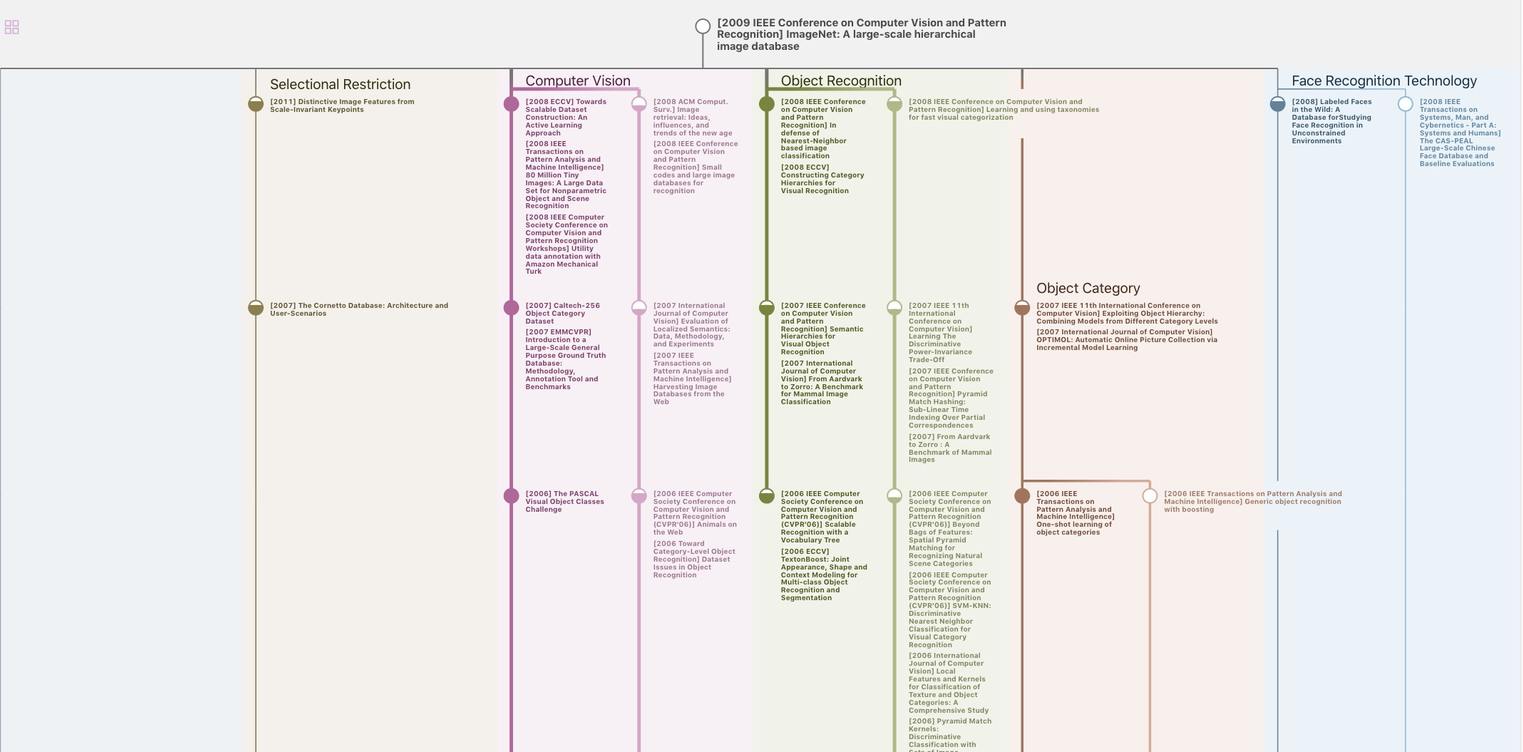
生成溯源树,研究论文发展脉络
Chat Paper
正在生成论文摘要