Continual evaluation for lifelong learning: Identifying the stability gap
ICLR 2023(2022)
摘要
Introducing a time dependency on the data generating distribution has proven to be difficult for gradient-based training of neural networks, as the greedy updates result in catastrophic forgetting of previous timesteps. Continual learning aims to overcome the greedy optimization to enable continuous accumulation of knowledge over time. The data stream is typically divided into locally stationary distributions, called tasks, allowing task-based evaluation on held-out data from the training tasks. Contemporary evaluation protocols and metrics in continual learning are task-based and quantify the trade-off between stability and plasticity only at task transitions. However, our empirical evidence suggests that between task transitions significant, temporary forgetting can occur, remaining unidentified in task-based evaluation. Therefore, we propose a framework for continual evaluation that establishes per-iteration evaluation and define a new set of metrics that enables identifying the worst-case performance of the learner over its lifetime. Performing continual evaluation, we empirically identify that replay suffers from a stability gap: upon learning a new task, there is a substantial but transient decrease in performance on past tasks. Further conceptual and empirical analysis suggests not only replay-based, but also regularization-based continual learning methods are prone to the stability gap.
更多查看译文
关键词
Continual learning,lifelong learning,incremental learning,evaluation metrics
AI 理解论文
溯源树
样例
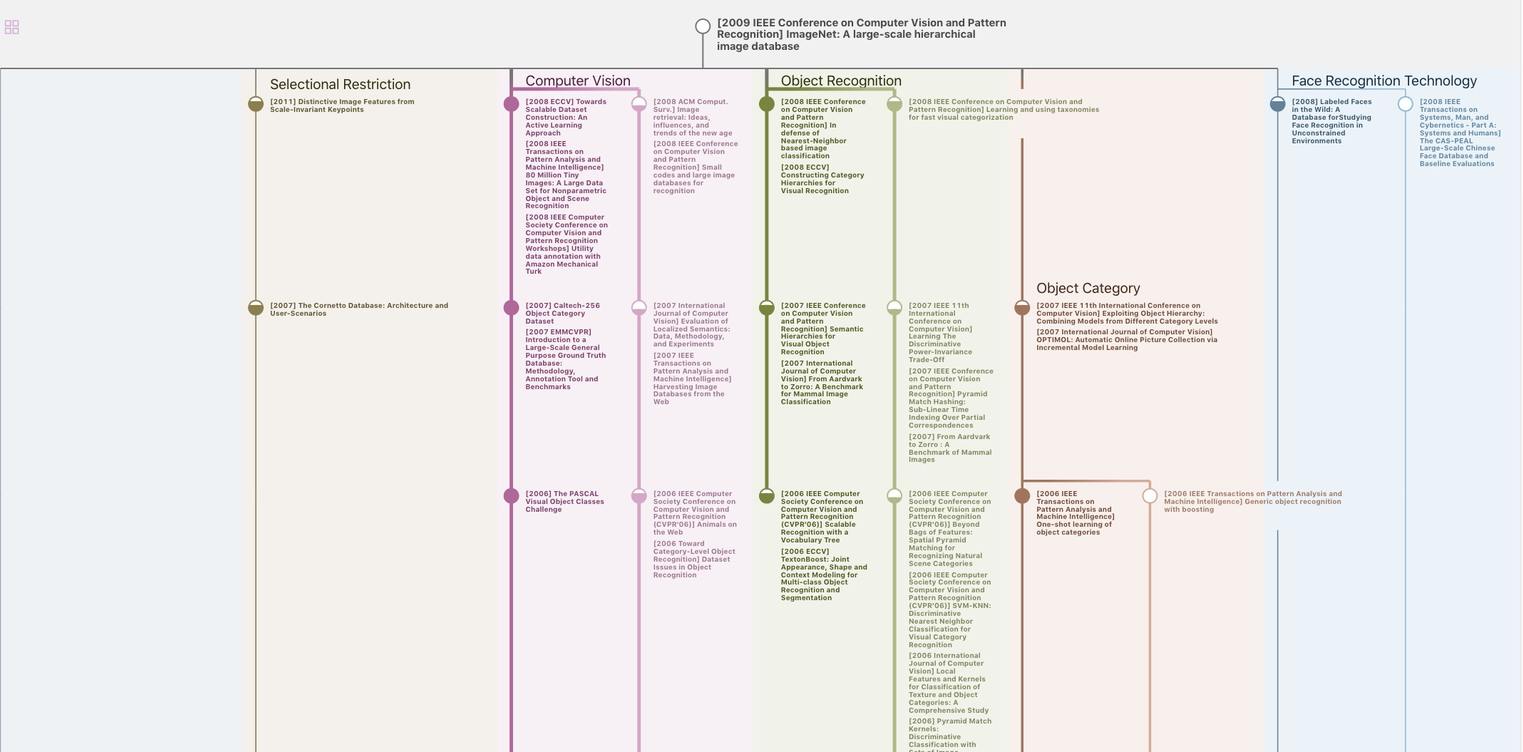
生成溯源树,研究论文发展脉络
Chat Paper
正在生成论文摘要