Combined Use of Glucose-Specific Model Identification and Alarm Strategy Based on Prediction-Funnel to Improve Online Forecasting of Hypoglycemic Events
JOURNAL OF DIABETES SCIENCE AND TECHNOLOGY(2023)
摘要
Background: Advanced decision support systems for type 1 diabetes (T1D) management often embed prediction modules, which allow T1D people to take preventive actions to avoid critical episodes like hypoglycemia. Real-time prediction of blood glucose (BG) concentration relies on a subject-specific model of glucose-insulin dynamics. Model parameter identification is usually based on the mean square error (MSE) cost function, and the model is usually used to predict BG at a single prediction horizon (PH). Finally, a hypo-alarm is raised if the predicted BG crosses a threshold. This work aims to show that real-time hypoglycemia forecasting can be improved by leveraging: a glucose-specific mean square error (gMSE) cost function in model's parameters identification, and a "prediction-funnel," that is, confidence intervals (CIs) for multiple PHs, within the hypo-alarm-raising strategy. Methods: Autoregressive integrated moving average with exogenous input (ARIMAX) models are selected to illustrate the proposed solution (use of gMSE and prediction-funnel) and its assessment against the conventional approach (MSE and single PH). The gMSE penalizes the model misfit in unsafe BG ranges (e.g., hypoglycemia), and the prediction-funnel allows raising an alarm by monitoring if the CIs cross a suitable threshold. The algorithms were evaluated by measuring precision (P), recall (R), F1-score (F1), false positive per day (FP/day), and time gain (TG) on a real dataset collected in 11 T1D individuals. Results: The best performance is achieved exploiting both the gMSE and the prediction-funnel: P = 65%, R = 88%, F1 = 75%, FP/day = 0.29, and mean TG = 15 minutes. Conclusions: The combined use of a glucose-specific metric and an alarm-raising strategy based on the prediction-funnel allows achieving a more effective and reliable hypoglycemia prediction algorithm.
更多查看译文
关键词
hypoglycemia forecasting,black-box identification,data-driven,glucose prediction
AI 理解论文
溯源树
样例
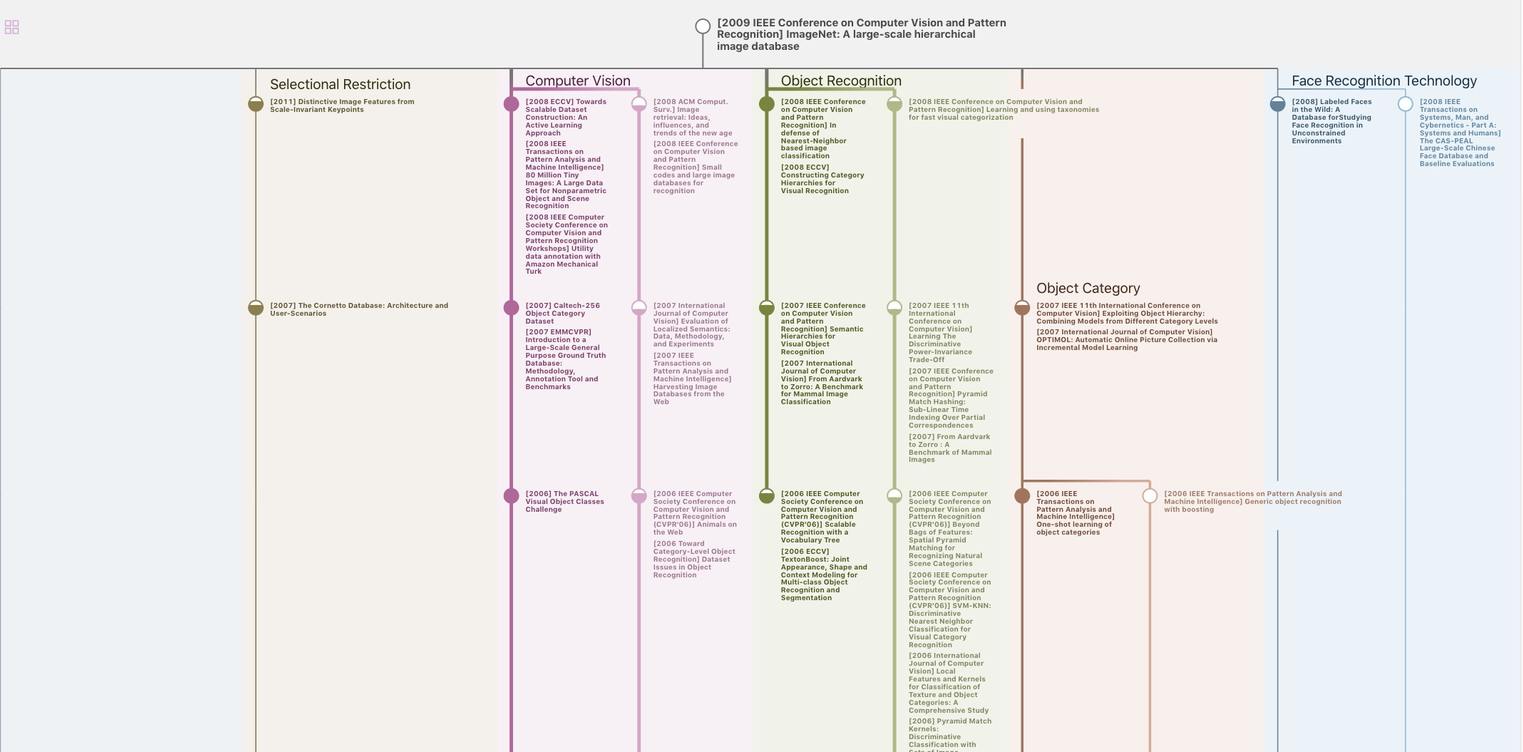
生成溯源树,研究论文发展脉络
Chat Paper
正在生成论文摘要