Treatment plan prediction for lung IMRT using deep learning based fluence map generation.
Physica medica : PM : an international journal devoted to the applications of physics to medicine and biology : official journal of the Italian Association of Biomedical Physics (AIFB)(2022)
摘要
PURPOSE:Recently, it has been shown that automated treatment planning can be executed by direct fluence prediction from patient anatomy using convolutional neural networks. Proof of principle publications utilise a fixed dose prescription and fixed collimator (0°) and gantry angles. The goal of this work is to further develop these principles for the challenging lung cancer indication with variable dose prescriptions, collimator and gantry angles. First we investigate the impact of clinical applicable collimator angles and various input parameters. Then, the model is tested in a complete user independent planning workflow.
METHODS:The dataset consists of 152 lung cancer patients, previously treated with IMRT. The patients are treated with either a left or a right beam setup and collimator angles and dose prescriptions adjusted to their tumour shape and stage. First we compare two CNNs with standard vs. personalised, clinical collimator angles. Next, four CNNs are trained with various combinations of CT and contour inputs. Finally, a complete user free treatment planning workflow is evaluated.
RESULTS:The difference between the predicted and ground truth fluence maps for the fluence prediction CNN with all anatomical inputs in terms of the mean mean absolute error (MAE) is 4.17 × 10-4 for a fixed collimator angle and 5.46 × 10-4 for variable collimator angles. These differences vanish in terms of DVH metrics. Furthermore, the impact of anatomical inputs is small. The mean MAE is 5.88 × 10-4 if no anatomical information is given to the network. The DVH differences increase when a total user free planning workflow is examined.
CONCLUSIONS:Fluence prediction with personalised collimator angles performs as good as fluence prediction with a standard collimator angle of zero degrees. The impact of anatomical inputs is small. The combination of a dose prediction and fluence prediction CNN deteriorates the fluence predictions. More investigation is required.
更多查看译文
AI 理解论文
溯源树
样例
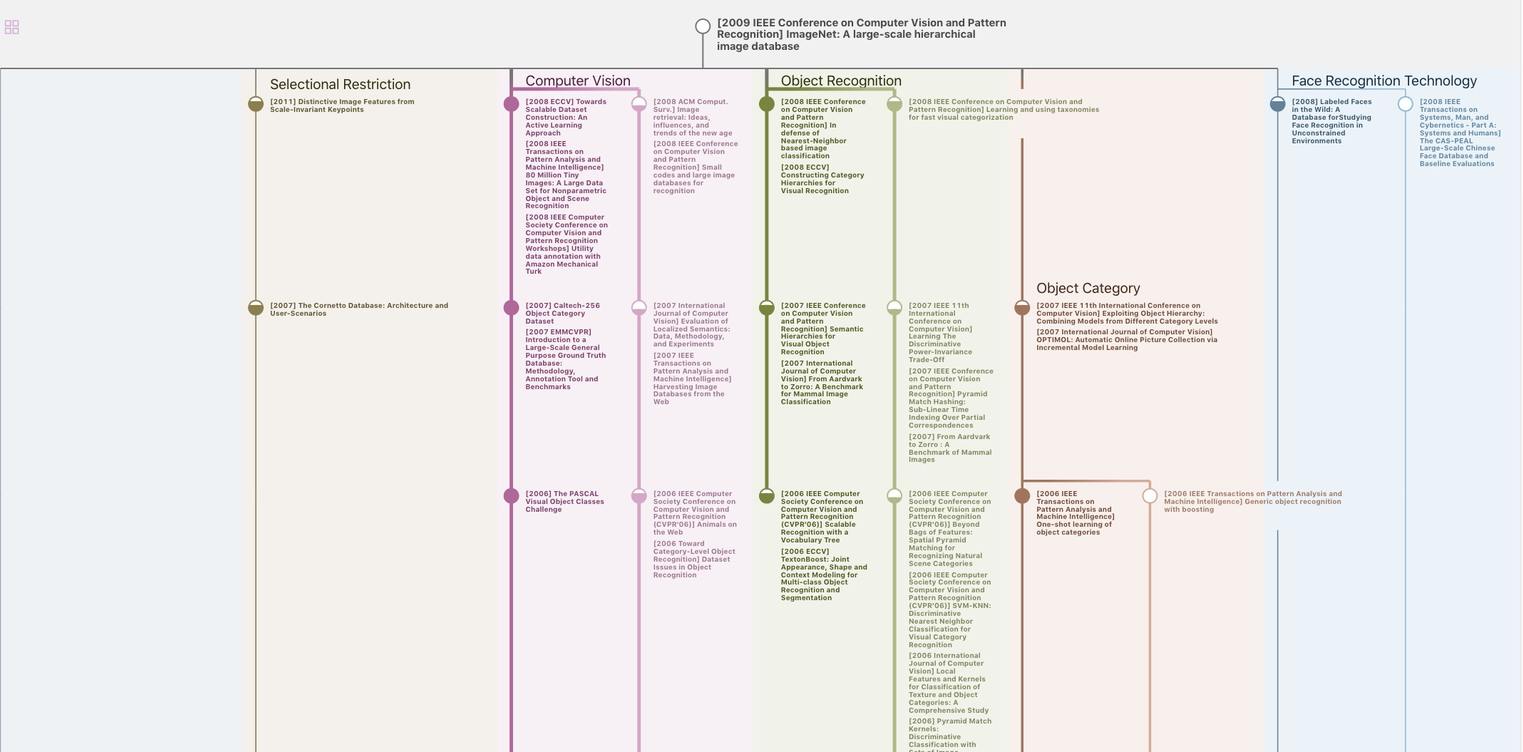
生成溯源树,研究论文发展脉络
Chat Paper
正在生成论文摘要