Ground-Truth Labels Matter: A Deeper Look into Input-Label Demonstrations
emnlp 2022(2022)
摘要
Despite recent explosion of interests in in-context learning, the underlying mechanism and the precise impact of the quality of demonstrations remain elusive. Intuitively, ground-truth labels should have as much impact in in-context learning (ICL) as supervised learning, but recent work reported that the input-label correspondence is significantly less important than previously thought. Intrigued by this counter-intuitive observation, we re-examine the importance of ground-truth labels in in-context learning. With the introduction of two novel metrics, namely Label-Correctness Sensitivity and Ground-truth Label Effect Ratio (GLER), we were able to conduct quantifiable analysis on the impact of ground-truth label demonstrations. Through extensive analyses, we find that the correct input-label mappings can have varying impacts on the downstream in-context learning performances, depending on the experimental configuration. Through additional studies, we identify key components, such as the verbosity of prompt templates and the language model size, as the controlling factor to achieve more noise-resilient ICL.
更多查看译文
关键词
deeper look,ground-truth,input-label
AI 理解论文
溯源树
样例
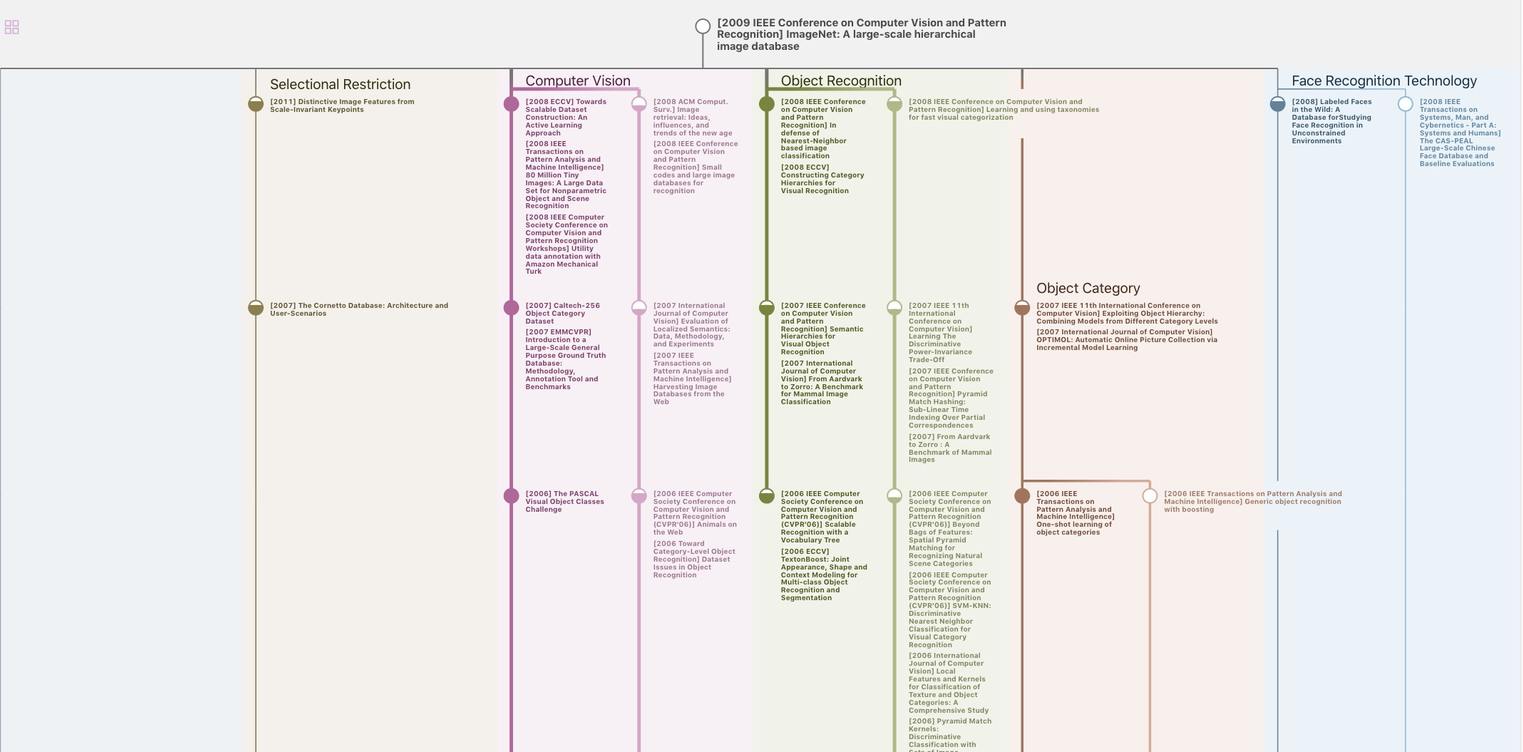
生成溯源树,研究论文发展脉络
Chat Paper
正在生成论文摘要